Quality Is As Quality Processes Do! With Rohit Choudhary, Kyle Kirwan, And Marie Hense
Almost everything is complex in businesses. Careful is as careful does! An old cliche but a good one whose wisdom strikes at the heart of the ongoing data quality renaissance. In the old days, data quality initiatives were one-off projects of cleansing addresses or fixing misplaced surnames. But on day one after the project, entropy slowly crept in again as false data overwrote the excellent stuff. For today’s savvy practitioners, many effective ways of fixing data quality are much more durable and efficient. Find out how this episode of DM Radio as Host @eric_kavanagh interviews several experts, including Rohit Choudhary of Acceldata, Kyle Kirwan of Bigeye, and Marie Hense of Toluna. Tune in to have a glimpse of what the future looks like.
—
Transcript
[00:00:45] Eric: We’re going to talk about a pretty important topic in the world of data. We’re going to talk about data quality and quality processes. Quality Is as Quality Processes Do is the title of our show. We have several great guests lined up for you. We’ll be having Kyle Kirwan from a company called Bigeye, Marie Hense from a company called Toluna and my buddy Rohit Choudhary from another company in this space doing some very cool work, Acceldata, all from different angles too.
This is going to be a lot of fun because data quality has always been an issue. If you’ve ever gotten an email address to someone else or with your name misspelled or gotten something in the mail with your name misspelled, it happens to me all the time. People call me Kevin. Kavanagh is my last name. Eric is the first name. They fuse those two and call me Kevin, which happens all the time.
Data quality problems can cause lots of trouble. Years ago, the Data Warehousing Agency did a whole report on the financial cost of bad quality data and it was through the roof. Several money companies spend mailing things to people who don’t live there anymore. Emailing people who don’t read this stuff anymore, getting the names wrong and customer experience is always harmed by bad quality.
In the old days, data quality was typically done in initiatives where you would come in and do a cleansing. You would fix the fields where you had the last name and the phone number, column and things of this nature but then as soon as that project was done, slowly but surely entropy would start chipping away and the quality would go down. You would overwrite good data with bad. There are lots of ways to handle data quality. We’re going to hear from three different perspectives here. Maybe a quick opening statement from each of you. Marie Hense from Toluna, say a couple of words about yourself and your vision of good data quality.
[00:02:30] Marie: Thanks for having me on. Toluna is a global market research company, which means we have access to hundreds of thousands of people across all corners of the world. What we help companies and organizations do is do market research studies and collect data and opinions. I’m responsible for data quality so that means looking at the methodology and how data is collected and cleaned. I also work closely with the fraud department that looks at the quality of our respondents. I’ll be looking at the first step of data quality, which is data collection.
[00:03:09] Eric: That’s such an excellent point. We’ve heard garbage in garbage out for years. That will never go away but I love the methodology and making sure that it is proven that it is auditable and you’re getting good quality data. The best place to address data quality is in jest when you get the data. You do need to be able to update it over time and we’re getting some of these new append-only databases out there, which are pretty cool because you can always roll back.
It used to be you wrote over the data, then you’d have to write over it again. If you write over good data with bad, that’s not so good. These append-only databases are an interesting development and the matrix of technologies out there but I’ll throw it over to Kyle Kirwan from Bigeye. Tell us a bit about yourself and what you want to talk about in this episode.
Collecting external data helps clients integrate that with their systems in their decision-making processes and add to their business intelligence. Share on X[00:03:54] Kyle: Eric, thanks for having me on. I’m the CEO and Cofounder at Bigeye. Bigeye is a data observability platform. Before Bigeye, I was the Product Lead for Uber’s Data Operations Team. A lot of what we do here at Bigeye is inspired by techniques that we pioneered to keep data reliable at Uber. They went from Postgres machines to Uber Data Warehouse to a multi-hundred-petabyte data lake. Several thousand folks are working on data pipelines all at the same time. Data quality is a pretty big challenge at that scale. I’m here to talk about data reliability engineering and how we can bring some practices from software engineering over to the data quality space.
[00:04:36] Eric: That’s great news and I’m glad you offered that history because one of the more fascinating aspects of our industry is that you will get companies like Yahoo which is almost the genesis of this phenomenon. In the late 2000s, you had this exodus from Yahoo. There were probably 30 or 40 senior engineers who went all over creation and started all these different companies. That whole big data movement if you look at it came out of Yahoo.
That’s where MapReduce was first engineered and then it was open-sourced. It became the foundation of Hadoop for that whole big data movement but we’re seeing it again. You’re a perfect example of these folks who were in these big companies doing amazing things with technology. They rolled their own. You learn about the practices of working with distributed systems, which in and of itself is a whole new ocean of challenges, problems and opportunities.
A lot of it has to be learned the hard way. You have to see the pain points, experienced them and figure out engineering ways to work around them. I’m very curious to get your perspective on this. Also, last but not least, we’ve got Rohit Choudhary from Acceldata, doing interesting things on pulling data from lots of different sources to give that strategic view of data quality processes. Tell us a bit about what you’re doing, Rohit.
[00:06:01] Rohit: Eric, thank you so much for having me on the show. We’re a multidimensional data observatory platform. Our goal is to enable enterprises to build better data products. When you look around the industry, everybody’s trying to build a data product and satisfy customer needs faster, get to them sooner and get to the best possible recommendation or the next best action. All of this is getting powered by data.
When we look at what people are trying to accomplish with these data products, it essentially is something that is operational and that is the core of the problem that we solve. How do you become more efficient with your operations? How do you ensure data is reliable as it goes into the hands of the business folks? How do you make sure that your costs and resources are always aligned with your overall financial goals as a company?
You were talking about Hortonworks and Hadoop. I was at Hortonworks as the Director of Engineering running open-source, close-source and commercial projects. Back then, we had this epiphany that this whole world is going to be multi-technology and multi-generational in terms of technology choices. The complexity of data is not going to slow down. We are right in the thick of it. Data is going to explode from here. I’m happy to be on the show and I would love to talk more.
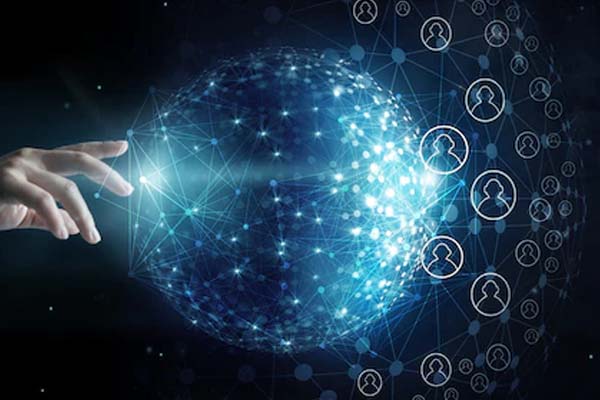
Quality Processes: We have access to hundreds of thousands of people across all corners of the world. We help companies and organizations conduct market research studies and collect data and opinions.
[00:07:19] Eric: You’re exactly right. We are right in the thick of it. It’s a very exciting time. There is this whole array of existing systems that we have to contend with as you’re talking about brick-and-mortar businesses. They’re all different and heterogeneous. They all have their way of solving things. I often think that the blessing and curse of the information technology space are there are so many ways to get any given thing done but first, you need to understand what stack we have or what is the DNA of our organization. What kind of solution is going to work for us? Those are all open questions and they’re moving targets but a focus on first principles always helps and keeping an open mind about what we’re doing.
This whole movement to the cloud is a fantastic opportunity, not just to move somewhere else but to re-factor, re-engineer and think through what you’ve been doing on-prem and find clever ways to leverage what’s available up in the cloud. That whole re-engineering process is extremely enlightening and folks like Marie Hense can help us understand what their market research is, what to look for, where to go and what are the opportunities. Marie, I’ll throw it over to you to dive into this whole research side of understanding what’s happening out there. What are your thoughts on the vector of appreciation for data quality and all the processes that go into helping achieve it?
[00:08:44] Marie: Data quality has become more of a topic over the last few years. We very much look at data collection and we help different organizations with their decision-making but we’re also collecting external data so that our clients can integrate that with their systems in their decision-making processes and add that to their business intelligence.
Garbage in garbage out. Prevention is better, more efficient and cheaper than remedy and it will probably be more accurate as well. Ensuring that the data that goes into an analytic system or the database is good in our eyes is the most important element of data. The decision-making that happens on the back of the data, the marketing or whatever it may be is as good as the data that goes into it and that it’s based on.
What we implement and acknowledge is the scale of the task that we’ve got at hand. There’s no way we can manually go through data and read what people said in the survey because we have hundreds of thousands, if not millions of surveys in our system throughout a year. It’s impossible to do that manually. We have to make sure we leverage technology and work a lot with natural language processing algorithms to identify, especially unstructured text.
Also, identify anything that’s looking out of the ordinary there to make sure that we leverage all of the advancements that we’ve had. Automate a lot of the data cleaning in the backend when it comes to preparing the data for analysis and cleaning it out. Also, making sure we analyze response patterns and the unstructured text that our respondents are giving us. That’s about that first step of making sure the data is as good as it can be before it gets integrated anywhere.
[00:10:43] Eric: These are all interesting points and we could do a roundtable show here. It’s going to be fun. I’ll throw this over to Kyle to pick up on. Marie hit one key nail right on his head, which is the issue of scale and that’s something that you certainly saw in your days at Uber. It’s always important for our audience to appreciate how things change when you get to a scale like that.
The bigger the outcomes, the more stewardship is required. Share on XYou cannot do manual processes when you’re dealing with these scale-out architectures. You have to use algorithms, classify, organize, get very sophisticated about your approach and automate whatever you can. Tell us a bit about that perspective, what you learned in your days and how you’ve used that knowledge to forge ahead with Bigeye.
[00:11:30] Kyle: It was fascinating to see what failed every 3 to 6 months. You’re scaling up things that maybe were a great decision at one point in time where the practical decision doesn’t last. That’s a fact of hyperscale and it’s okay. There’s nothing wrong with it but it was interesting to see what the breakpoints were. The one thing that comes to mind in the data quality areas specifically was switching from the testing paradigm to the serviceability paradigm.
There were 3,000 weekly active users internally when I was on the team and that was anybody that was an analyst writing a query or building a dashboard. It might be a data engineer creating a pipeline for their team to use or somebody adding a feature to the feature store that would be used in the ML platform. We tried initially to help folks say, “When something goes wrong in my part of the pipeline or even in the part of the pipeline that’s upstream for me, how do I know about that before my end users do?”
The easy approach, in the beginning, was, “Let’s build a test harness.” You could go in and write a query and say, “If the row count in the child table doesn’t match the row count in the parent table, then we have a problem. I don’t want any duplicate values in this primary key column,” or something like that. That was a great first step and you can already see where that falls apart. You wind up with a 1,000-column table that’s a bunch of ML features.
Even if you wind up with a 75-column table that is some big fact table or something like that is used by a bunch of different teams, you get to that size and it becomes infeasible to anticipate every single failure case where you want to write a test for it. That’s where we had to start moving to an observability paradigm and instead say, “Why don’t we harvest metrics from each data set periodically?” We can do signal processing techniques on those instead of asking a human to predict each possible thing that could go wrong and write a test case for it upfront.
[00:13:31] Eric: It’s fascinating to remember that you have to reevaluate. You’ve made practical decisions. They were the right decision at the time but then you reach a point where it’s like, “This is not working anymore. How can we change it?” You see this all around in the observability space, which I love because we can see things. Once you can see little signals that you couldn’t see before, that gets very useful for troubleshooting, planning and so forth.
I was impressed with the demo I took from you where you’re pulling from lots of different sources and helping coalesce that bigger picture view. That’s the future. We’re going to have to continue doing exactly that. Adding in new sources, constantly checking them but have that automated and have the dependencies clear. What do you think about all that Rohit?
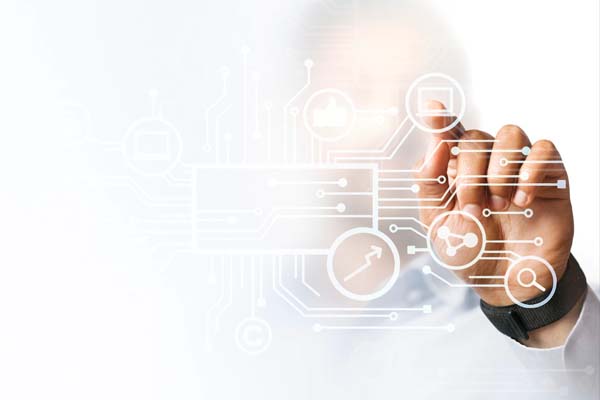
Quality Processes: We’re basically a multidimensional data observatory platform. Our goal is to enable enterprises to build better data products.
[00:14:20] Rohit: You made some interesting points. When you start and make a decision, it feels right but it breaks down pretty quickly. If you abstract out and up-level a little bit, what we’re seeing is the early days of the software development life cycle. I might as well even call it the data development life cycle. The practices are not standardized but the challenge also is that when you have tables that have 1,000 columns, it’s pretty ordinary. You have tables with 20,000 columns sometimes and that almost becomes unmanageable. You have no idea what’s coming. You don’t know which transformation upstream created that table and how long is that table supposed to live.
What’s the veracity of it? What is it supposed to contain? What’s the velocity at which new data is getting added or when is it expected to refresh? This is a reality that everybody’s dealing with, whether it’s a telco, a retailer, a bank or managing transactions. Our viewpoint has been very simple. You got to thread the needle and therefore, to your point, can you bring all these systems together?
Can you observe the signals that are coming from the infrastructure, the quality of data or the pipelines? We tap these business processes and give them a holistic view of what the data product looks like and what’s the inner ongoing. We are out to do a complete visualization of how does the landscape look like. What’s the state of the union? Is it going to get worse or are there ways by which you can make it better?
As times progress and as more people start investing in these practices, there’s going to be a clear delineation between pre-production and post-production. There’s going to be a lot of testing pre-production but with post-production, it has to be observability because testing is not the answer. If you look at frameworks like Great Expectations or DBT, they are exemplary tools and technologies. They will do a lot better but they’ll do a lot better pre-production. With post-production, observability is almost mandatory.
[00:18:57] Eric: Data quality processes and what a fantastic line of guests too. We’re talking to Marie Hense from Toluna. We’ve got Kyle Kirwan from Bigeye and Rohit Choudhry from a company called Acceldata. Look those guys up online. We’re talking about data quality processes and I think to myself that one of the important things we’ve seen in recent years is companies that have an information architecture that is flexible enough to allow front-end workers to make changes to backend systems.
Append-only databases are useful in that regard because you don’t write over the data. You only append the data and you can roll back. It’s version control stuff but it’s always good to know what people in the front lines see. They’re the ones typically interacting with the customers, the partners or whomever. They’re the most intimately engaged with the data as it’s being used for a particular function. Why not let these people do something?
I talk all the time about morale and the importance of having good morale in your organization. Friction points that don’t get solved lead to frustration, which eventually causes morale and that can take a company down frankly. One of those friction points is when I’m an end-user on the front lines of the company. People always tell me their name is misspelled. I am not able to fix that for them. Marie, I’ll throw it over to you to get your thoughts on that and tell us your other thoughts about how to improve data quality programmatically.
The benefit of the cloud is that you can react faster. Share on X[00:20:25] Marie: You are hinting at sharing the responsibility of data quality and data accuracy. This isn’t only 1 department or 1 person’s job. This has to be a cross-company team effort where everyone who is in touch with the data, whether that’s at the collection stage, the management storage stage or the analytics stage is responsible for creating that feedback loop of ensuring that the data quality and data accuracy is there.
If an analyst, for example, realizes that the data that they’re looking at doesn’t match up with market knowledge that they have or with data that they’re getting from elsewhere, that feedback loop should be there to ensure that it can be reviewed to ensure if the data is collected and stored appropriately. Is the data clean enough for the purpose that it’s being used? Those tools that give access to different types of users across that data journey are extremely important to collectively ensure that data quality is at an appropriate level.
[00:21:34] Eric: I do love that you’re doing this market research. You’re capturing what is real-world data and for your clients, you’re giving them access to things like benchmarks, what you should expect in this industry or in that vertical. That’s great stuff. Marie, I’ll throw it back to you. You can have your first-party data and perspective on what’s happening in the world but everyone has a bias. That’s why you want a team around you to point out the blind spots or mention things that don’t look right. You didn’t have a good corporate culture where people feel the psychological confidence to raise their hand and say, “There is a problem over here.” They’re not going to you and that’s a real problem.
There are whole books and movies written about this phenomenon but I love that you’re bringing this real-world data because it’s a bit of a tonic and also a bit of a check and balance to say it could be your view of the world is correct and the rest of the world is different because your business model or DNA is different. It’s always good to get a reality check, look at the real world and compare it to your numbers. What do you think?
[00:22:45] Marie: Having that frame of reference and evaluating data critically is part of the checks and balances, the feedback loop and the evaluation process of data quality. Not taking the data as gospel almost and saying, “This is the data and this must be true,” but critically evaluating where it came from, what happened to it up to the point where it was analyzed or used as part of the data quality journey.
[00:23:14] Eric: I’ll bring Kyle Kirwan back in from Bigeye to comment on that. What are your thoughts about the importance of this collective responsibility? Understand that 1 person needs to be responsible for 1 department but it is a group effort and you want to make sure to keep the lines of communication open across the organization and with your partners as well.
[00:23:35] Kyle: As you scale up, you need more of the organization to be able to help tackle the problem together and that comes down to, “Can you divide up responsibility of that scope?” That would mean being able to say, “Here’s raw data ingestion. Here’s the group of people that are responsible for an SLA around raw data ingestion. Is that landing in the warehouse on time?” Separately, you might have a different group of people who are responsible for the last mile of processing before that goes out. It’s used in marketing analytics or something like that.
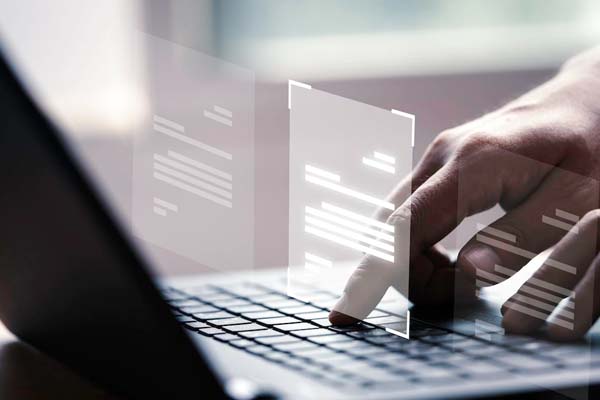
Quality Processes: Today, when you look around the industry, everybody’s trying to build a data product, satisfy customer needs faster, and get to the best possible recommendation for the next best action.
Being able to map that pipeline and split up the responsibility for problems that can occur in different stages of that pipeline and have that ownership get clear to those two different teams is what allows you to get that separation of responsibilities. You can route those responses to the correct team when you detect a problem in a different place in your pipeline.
[00:24:27] Eric: I’ll bring you in Rohit to comment on that as well. It is always important to nail down your processes and know who’s doing what. You all think a lot of that stuff is easier to manage in part because of observability. That’s one thing I love about the cloud. I feel like one reason why the cloud is so powerful is that we learned so many lessons through decades of on-prem and then along comes this interweb thing.
It took a while for big corporations to fully embrace it and partly thanks to Microsoft but the broader, big Fortune 500 or Fortune 2000 world is all on the cloud. Everybody knows the cloud is the future. In the infrastructure of how the cloud is built, you have all of this information baked perfectly so that you can grab it. That’s what observability is all about. It’s tapping into all these sources of signal to be able to see what’s going on. What do you think Rohit?
[00:25:21] Rohit: The key point from a quantity perspective, whether it’s cloud or on-premise is all about stewardship. Stewardship is essentially saying, “I own this data. I understand what the nuances are or whether this is going to make sense for the business or not.” This is not a generic field. This is very specific. Healthcare data steward is domain-intensive. They understand what’s going on with the data that is eventually going to be available for a pharmacist to take a call on medical trials or whatever it comes out.
It’s very similar in the finance domain. If you’re trying to look at the ratios of cash in the bank versus cash that should have gone out from an investor’s point of view, even the slightest change could leave $150 million to $200 million additional in the bank, which could have been very well in the money market. We’re talking about things that affect a lot of outcomes. The bigger the outcomes, the more stewardship is required.
The benefit of the cloud is that you can react faster. You asked Marie this question. Can the end user input or correct what they’re seeing? The biggest benefit that you have is when you start thinking about your data in terms of domains. That’s a domain that has its set of pipelines. It runs on infrastructure and here is the outcome of the reliability of data. Here’s another domain which is called financial cards. Many times, we ran the credit card fraud check and these are the outcomes that we got, whether this data is reliable or it’s not but it’s useful for new large corporations or small to start thinking about their data in terms of various domains.
[00:27:06] Eric: As I think about the many challenges, we also have this whole reality of streaming data. A lot of the data quality processes and technologies that we built were all geared around data that has persisted somewhere in a database. We have all these different technologies to stream data in Kafka or any number of other technologies. Streaming is not tremendously new but there are a lot of different technologies that are changing how it’s done. How does that change the landscape, Kyle? Can you apply policies for persistent data that can be very easily ported over to streaming data?
It's got to be a combination. It is a mutually important world right now where if you don't apply skill sets and technology together, you're not going to get the right results. Share on X[00:27:44] Kyle: It’s an interesting challenge. Part of the lineage mapping work that we did at Uber was being able to tie data that was landing in HDFS. It’s a constant topic that was coming from originally. What was critical there was having a schema registry where the team wanted to generate a new topic and expected data from that topic. It would land in the data lake and would go to the schema registry to define its schema.
There’s their topic. They can start publishing it to it and then know that the data is going to arrive in a raw table in the lake. It can be used downstream from there. In that case, it might be okay to apply data observability initially to the persistent data and monitor it at rest but still, a step change above where a lot of organizations find themselves stay. They’re not doing observability at all.
Being able to monitor data at rest is still a huge benefit. If you can start to move upstream after you have that downstream piece covered and monitor things like how many messages are moving through the topic or do we have no values in fields that we don’t expect to have, then you can start to identify issues earlier before they landed. There’s still a tremendous amount of value in starting your monitoring downstream close to the application and then starting working backwards towards the upstream sources.
[00:29:07] Eric: As I think about all this stuff, there are so many ways you can glean information. The one key point is you’re always going to need people. All these new tools are great but you’re still going to need people to look at it, understand it, make comparisons, contrast, call people or email someone, “Is this right?”
You’re never going to get to a point where it’s lights out ever. The world is getting more complex by the day with new sources, models, schemes and so forth. Don’t fear losing your job. It’s going to be a better tool to help you stay on top of what’s happening but there will always be a need for people on the front end here, comparing, contrasting, emailing and communicating around this stuff.
[00:29:53] Rohit: We were one of the first companies to come and say, “Observability applies from your data at rest and data in motion.” Finally, data is ready for consumption. We give you an insight across all your systems, whether you’re streaming data from Kafka using a Spark’s team processor or whatever it is and whether you’re getting data from your relation data sources from CDC. We cover the whole spectrum.
To your point about humans in the loop, I can tell you that humans cannot deal with the complexity, especially at the volume. Therefore, they need tools but tools alone do not bring in the domain expertise that humans have in their heads. It’s got to be a combination. It is a mirror of the mutually important world. If you don’t apply skillsets and technology together, you’re not going to get the right results.
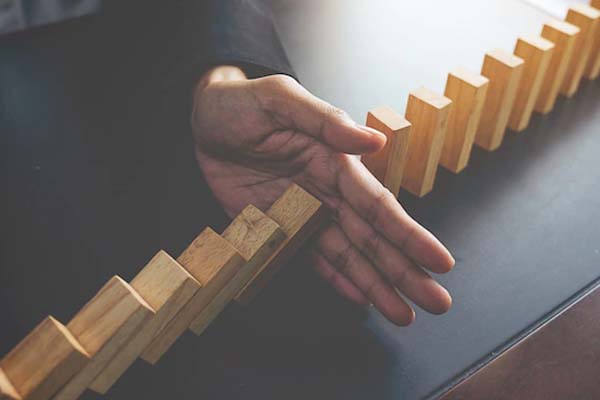
Quality Processes: Prevention is better, more efficient, and cheaper than remedy. It will probably be more accurate as well.
No matter how much technology you fly in and how much machine learning we apply to all of these different patterns and processing signals, you still miss out on some of the critical factors. It’s a combination of these two. The evolution of it could be very intense domain-centric observability patterns, which at least, we are already seeing in our customer base.
[00:31:08] Eric: Marie, I’ll bring you in quickly to comment. From your research and what you’ve seen in data quality, do you see organizations gaining this appreciation for the step change? When you scale out, the scale of architecture is a whole different environment from the old manual processes of yesteryear. Are you seeing in your research that companies seem to be figuring out what these gentlemen are saying which is to get some tools and the people to work with them but don’t try to hack through these forests with a sword? What do you think?
[00:31:42] Marie: What Rohit said rang quite true. That balance of people, tools and how it has to work together reminded me of how we were applying NLP algorithms to clean some of our unstructured data. One of the things that we check for is gibberish in unstructured open-ended text answers. Here’s the challenge though. A lot of Gen Z and younger folks write in a lot of abbreviations as we learned and as we were training our model.
In the beginning, we were accidentally in the training stage removing a lot of those respondents because our gibberish model was flagging them for providing data that said wasn’t good enough but only human intervention was enabled to identify, “This is how these people talk these days. This is how this group of respondents talks. These are valid abbreviations.” Having that balance between the tools and humans is crucial.
It’s something that we recognize that without tools and the right technology, we’re not able to scale this mountain of data and data quality but it has to be done hand in hand. The tools can’t do it on their own because there is still some subjectivity when it comes to what is accurate or what isn’t. One of the things that we need to watch out for is to not over-clean the data where we remove data that was fine. We only thought it wasn’t.
[00:33:10] Eric: #SMH, #LMAO, #LOL. They’re all the clever little things where you have to be like, “What does that mean?” Don’t be afraid to google things. When your kid says something, I cry. “What was that?” I was shaking my head, laughing my A off. I got you. I was reminded in the break of my thesis back in college on deconstruction.
Jacques Derrida wrote a piece, Structure, Sign and Play in the Discourse of Human Sciences, where he broke down a structuralist view of the world. In the data world, this is very clear. We have tables and schemas. These are the structures that we’ve come to know and understand in the news but the world is a very complex place. It’s hard to take a human being and compartmentalize them into some three-dimensional construct
Without the right technology, we're not really able to scale this mountain of data. Share on XYou can do it for purposes of understanding things and trying to make the best recommendations for their next purchase but human beings are very complex creatures. Businesses are very complex, even plants are complex. There is something to be said for hyper-structural thinking, finding the way to dissolve that and get around it. What do you think about that, Rohit?
[00:36:59] Rohit: Going back a few years, I could be described as a person with a phone number, a street address, an email address and a mailing address. I could be a customer, which is an inviolable entity within an enterprise and I’m qualified. How are you using the information that you have about this person? You are not just using a flat. You’re going and adding, “What am I buying traits? What am I browsing traits? What are my location trends? Where have I been? What is my recency data?”
What ends up happening is that when marketing is approaching, I was approached very differently from how a lender would approach me because the lender knows my address. He only cares about whether he’s going to find me or not. Marketing is very influential. You’re trying to influence my behavior. Therefore, you’re trying to understand me better. You’re making big decisions.
For example, corporations are spending billions of dollars on advertising and they’re doing it. They are hyper-targeting people based on a better understanding of who this person is. The biggest change that’s happened in terms of data quality is that if you’re going to make a financial decision, which is to spend money on identifying the traits and the benefits that somebody like Rohit may get and to nudge them in a certain direction to purchase our service or product, those data points have to be very accurate. That is where the recording has changed.
It’s no longer about correcting phone numbers and ZIP codes. It’s about identifying those additional attributes that the business has collected over some time. Some of that may be very transactional and it’s coming accurately from your databases but some of it is coming from third-party sources. You’re only trying to acquire additional traits and identify us from my ZIP code but you’re also superimposing my being with the rest of the friends that exist in my ZIP code.
That is where the whole lack of structure is heading, the previous relation data quality companies, as I’m talking about the industry but every enterprise is dealing with quality. Who’s going to deal with this lack of structure when half of the video is still coming through streams and coming in with high velocity? Those are the things that have gone off the rails and deconstruction of life.
[00:39:18] Eric: I’ll bring in Kyle to comment on this. You have schema-on-read. It used to be in the old days that you’d have your schema after your data warehouse. If you want to load data, you had to match that schema perfectly when you loaded it in. They then started talking, “Don’t worry about that. Dump it in the data lake and we’ll do schema-on-read.” It does make sense. It’s a clever way of decoupling the structures so that you can get more stuff in but there are some pretty significant challenges around schema-on-read as well but that fits pretty nicely into this whole concept of structuralism and deconstruction.
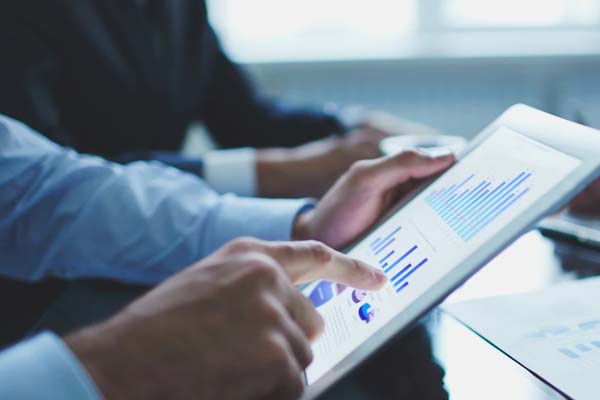
Quality Processes: Having that frame of reference and evaluating data critically is part of the checks and balances, the feedback loop, and the evaluation process of data quality.
[00:39:55] Kyle: You were talking about this idea of having the internal or mental structure that you apply to the world around you. I was thinking it’s like schema-on-read for the way you’re going about living your life. We were talking about testing and observability. This hits the nail on the head there. If the structure of the data is going to be in flux, then we can’t presuppose things or write rules anymore. We have to move on from that to say, “This is the state of it and how it’s changed.”
We see the shape of the array has changed. There are new fields that have appeared. Old fields are populated anymore. The types of values that we see in a column have changed. I agree with what Rohit was saying. We have to be able to handle that. That is the new normal and that means that with an observability approach to quality, you’re only looking at what is there in an un-opinionated way. You’re looking for signals that tell you that something might be impacting an application and you might need to do something about it but it’s a less opinionated and less structured approach.
[00:41:00] Eric: Marie, I’ll bring you back in with one of my observations from years ago in my philosophical discovery phase, which is that people notice differences. What I mean by that is if something is the same all the time, you don’t even notice it anymore because it’s the same all the time. Your brain is designed to capture, identify and then process differences. What is different now than it was yesterday or a moment ago? Whether that’s a predator coming towards you or some prey that’s available to you, whatever the case may be. The point is, if you do the same thing every day, your days are going to fly by and you’re not even going to notice them
To shake things up is a very useful practice to be able to gauge and regained because even if you’re not changing, the market or some dynamic is in your environment. Let’s face it. We all need to know what to focus on. I talked about this in a keynote. I said, “Priorities, biases and solutions.” What are your priorities? Always be mindful of your biases and what solutions are you trying to build. Data quality can be a real good indicator of what to focus on. If it’s getting worse, you better start doing something to fix it. What do you think about all that, Marie?
[00:42:23] Marie: Throwing back at your comment that if you do something every day, you don’t notice it changing. It’s like the frog in the pot. You turn up the heat. It’s maybe changing but you may not be noticing it because it’s changing so gradually. It feels the same as yesterday but it isn’t. That’s going back to the frame of reference. Let’s talk about data or data trends.
If you’re looking at your data and you see it looks the same as last week but last week looked a little different from the week before and that looked a little bit different from the week before, it’s important to take a step back. When looking at the data, we hold on to the data. We analyze to take that bigger picture view and have a look at how data compares, not only to the previous week or the previous two weeks but overall whether a certain data story has changed.
That can identify whether there’s anything that has changed in how that data was collected and cleaned and whether any of those processes are impacting the data and therefore the insights or the applications that we get out of that data. Is there any bias anywhere in the process of collecting the data and categorizing the data? Are there certain assumptions being made about who should be included or excluded from the data and may that be changing the outcome and the insights that are based on that information? That bigger picture view in my eyes is extremely important.
[00:43:56] Eric: We’re always at work in progress here. I’ll get maybe a final comment here from Rohit. You always have to start somewhere. I used to lay out newspapers. We get the dummies from the crew in the back and they would show me all the space I had to fill with my content. I would have to figure out which stories go where and which photos I use to use up the space because it wasn’t online. It was in print. You have to cover every little piece of print. I learned very early in the ballgame, you have to start somewhere, build out and handle those edge cases that you get towards the end. That’s what you have to do with data. What do you think, Rohit?
[00:44:32] Rohit: Look at your surface area. If you’re an enterprise data leader, you’ve got to take stock of how big your surface area is because in some cases, it truly is scary. Therefore, you start with very specific small initiatives. Finding out what are the financial implications of poor quality or unreliable data. What does it change? Is it going to affect you by $100 million or $50 million? What is the real impact of a lack of trustworthy data? That would be my first reaction.
Second, recognize that the word is multidimensional and so are data systems. Therefore, you pay attention to all the different layers that can go wrong, all the different problems that they go on whether accepting infrastructure level, which is then causing delays in your streams and therefore your data appearing unreliable.
The transmission is failing continuously or maybe the consumption layer is out of whack and out of sync with where it should be. Identify these multidimensional relationships between each of these and what is the final data part that you intend to cater to and what is the audience. Is it a business analyst community?
[00:45:41] Eric: Fantastic advice from all of our guests. Look these folks up online, Toluna, Acceldata and Bigeye. I had so much fun learning from the experts.
Important Links
data collection decision making information technology software engineering solutions structured approach