Domain Specific – Why Data Mesh Works With Mike Ferguson, Bin Fan And Adrian Estala
Could there be a hotter topic than Data Mesh these days? Some say it’s still largely theoretical, but the promise of data mesh does make sense – give discrete groups within an organization dominion over their own data sets, which would be housed within a broader data platform that enables self-service development. A key aspect of the vision is to build data products that are designed and managed by focused departmental teams. Ideally, computational power (and cost) would be carved out according to business value. Sound intriguing? Check out this episode of DM Radio to learn more! Host Eric Kavanagh interviews legendary analyst Mike Ferguson of Intelligent Business Strategies, Bin Fan of Alluxio and Adrian Estala of Starburst.
—
Transcript
[00:00:40] Eric: We have a hot topic. We’re going to talk all about data mesh. I’m super excited about the panel we have put together here. My good friend, Mike Ferguson, who I’ve known for several years in the industry, is a very knowledgeable analyst, a consultant, and a practitioner. He goes out and does this stuff, but he doesn’t just talk about it.
We’ve also got a newbie, Adrian Estala, from Starburst. These folks are doing interesting work. A bunch of dedicated folks went out and created Starburst. They’re doing some work in the analytics space, helping with amounts to federated queries. This thing called Presto is out there. We will talk about that a bit on the show. He’s a former long-time practitioner and was at Shell. He’s CDO there. He said anytime he gets the warm and fuzzies to do something new, he gets excited, and that’s when he jumped over a Starburst.
My good buddy, Bin Fan, is from Alluxio. It’s an interesting technology company that spun out of the whole big data movement. We’ll find out some fun stuff from him. The topic is data mesh. What is data mesh? It’s an interesting concept. There are several key tenants to it. One of them is self-service. You want to enable self-service, but one of them is federated domain-specific data management. You let a department or a certain group manage their own environment, which is rather different from the old way of building a data warehouse and pulling everything into there.
We learned along the way somewhere that you couldn’t get everything into the warehouse. That’s when data lakes came into the picture and the big data, the NoSQL world, frankly. It was funny because we almost made the same mistake again. It’s like, “No, let’s put everything in the data lake.” That didn’t work out too well. For some people, it does, but it becomes a bit unwieldy, and there’s been a whole lot of innovation in this space.
Data mesh comes along as a way to divide a little bit to give the control to the departments to enable self-service but still have an underlying fabric. Some folks like our buddy, Bruno Aziza, will say, “You need a data fabric to do a data mesh.” We’ll talk all about that on the show. With that, I’ll hand it over to Mike Ferguson. Mike, tell us a bit about what you’re working on. You’re the co-chair of this big event in London, which is exciting. Tell us what do you think data mesh is? Why is it popular now?
[00:03:07] Mike: Thanks for the invitation to be on the show. Data mesh has come about because of a major problem. A lot of my clients are running with different standalone analytical systems. You talked about data lake and data warehouse. It may have other types of NoSQL databases using analytics like graph databases, streaming analytics, etc.
What was going on in all of those environments was separate data integration tools to be able to prepare and integrate data for each of those individual systems. They’re like a siloed approach. What happened was that you got different people often using different tools in different silos and repeating the whole approach of integrating data from the same data sources.
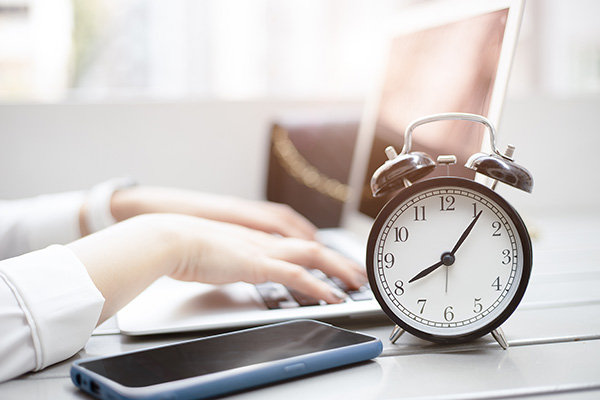
Data Mesh: We’re trying to eliminate far too high data integration costs. We’re trying to shorten the time to value by reusing. So build once and reuse everywhere, rather than everybody building for their silo.
In addition, the folks in the middle, who are doing this work, whether IT pros or even, in some cases, data scientists, are quite some way from where the source data is necessarily used. With more data sources coming on board and more business users around the business were demanding access to data for analytics and insights, the folks in the middle are becoming a bottleneck, in all honesty. Not only do we get siloed systems and repetition of tools in integrating the same data repeatedly, it allows time. There is a lot of redundancy in there. At the same time, we’ve got a bottle of that going on because we’ve got more data and not enough people to do the integration centrally.
It’s like, “Why can’t we decentralize the development of pipelines to produce data? Why do we have to build one huge data store each time? Why can’t we build a more component-based data development?” If you like data components and data by a state called Data Products, you can incrementally build up a list of different teams in different parts of the business doing that. It allows folks to pick the ones that they need for the analytical use case that they have.
The idea of being is I can build it once and reuse it everywhere. It stops reinventing. There are a lot of challenges with it. One of the biggest challenges, in my opinion, is if we’re going to do this, how do you get everybody out there with the skills to be able to do this work? The challenge from a centralized IT environment is to admit that there’s no way they’re going to keep pace with demand. At the same time, coordinating all of this development going on across the enterprise, understanding who’s building what data products for what purpose, and how it’s going to contribute towards achieving a business goal.
If you’re trying to improve customer engagement, reduce fraud, or optimize the supply chain, what data products should we be building? Not only coordinating that but upskilling all of those folks. We do get a hell of a lot more people around the organization that are perfectly comfortable and capable of doing this work.
We’re trying to break a log jam here and get rid of far too high the costs of data integration. We’re trying to shorten the time to value by reusing. Build once reuse everywhere rather than everybody build for their silo. At the same time, we’re trying to get far more teams doing it. From most people’s perspectives, a lot will depend on the culture of your organization.
If you’re highly decentralized in a way, this fits to some extent with the way your organization may operate. I’ve got several clients that are highly decentralized organizations, but I think as well, companies are okay with this as long as they’re not fueling 20 different versions of the same thing coming out of 20 different parts of the business.
What they want is coordinated development of people, building stuff that’s going to, if you knit together to solve different analytical problems and shorten the overall time to value. It’s about a cost reduction, speeding up development, and reuse, but at the same time, in order to stop it from going off track, it’s also about coordination.
Data mesh is very much about cost reduction and speeding up development. Share on XI put a high priority on the whole organizational side of this. I’ve had a request from seven CDOs to say, “Could I tune in to a meeting with them and take them through the organizational implications of this?” All of these CDOs are recognizing we can go out, buy the technologies, and everything like that. They’re far more worried about the soft side of this than the technology side. They feel that organizing this and coordinating is going to be as important as anything else in order to let everybody know who’s building what. The whole thing ultimately stitches together a bit like a front of a jigsaw box.
That’s going on, and at the same time, there’s another trend here. If you look around, it’s already happened in software development. We went from monolithic applications to microservices and what we’re seeing now. In a way, we went to component-based development. We see the same thing, moving into data and analytics, whether it’s data model components or pipeline components that are orchestrated together in a data ops pipeline.
We’re trying to automate things like automated testing, automated deployments, infrastructure as code, and all of those things to shorten the time to build, get collaborative development going on, and get stuff out the door. Also, to find a way to publish what’s being created so that people can ultimately shop for data products that they need and equally then quickly assemble ready-made into what they need in order to deliver value. The last thing you’d want is if you produce data products to find that somebody done got to do even more work on top of that in order to use or what’s being created.
What people want is it’s almost like kin to Lego bricks. I want to grab these pieces, snap them together, and start analyzing and delivering value. We’ve got data product producers on the one side, which we’re trying to get more of with the skills needed to do it who are closer to the data sources. At the same time, we’re trying to encourage sharing without violating compliance and all those kinds of good things.
At the end of the day, can you shorten the time to value? Can you accelerate this and speed people up because 60%, 70%, or 80% of what they need is already made? Rather than what data I am going to use, I’ll have to go through all these sources. It’s like, “I got all these data products and it is ready-made. I don’t have to go and do this stuff. That’s related. I can grab that, snap it together, and start analyzing and delivering volumes.”
This decoupling of producers versus consumers is a good idea, but I do think from a corporate perspective, CDOs are concerned about not ending up in a Wild West environment. What they’re concerned about is, “How do we coordinate this? Make sure we’re delivering on strategic business goals to hit high priority targets and stuff from a business point of view.” Nobody is losing track of that.
One of the big things coming out of a lot of my consulting at the moment is CDOs are creating what I would call a program office. Nothing new there, but it’s like a nerve center because everybody knows which projects are going off for what purpose and which teams around the enterprise are building what. It also gives those teams around the enterprise a view of the bigger picture.
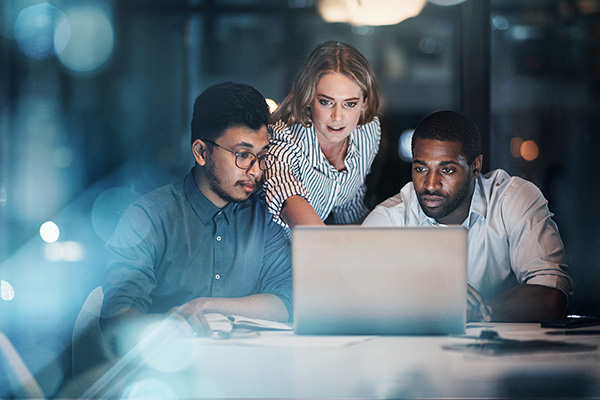
Data Mesh: We’re trying to automate things in order to shorten the time to build, get collaborative development going on, and get stuff out the door.
It works both ways. It works from a management perspective trying to keep track of all of this, but it also works from the individual team’s point of view to know which piece of this puzzle am I building. I’m finding in my consulting that the executives are worried about the organization. They are not worried about technology. They’re keen to up-skill.
CIO’s concern is realizing that there’s an enormous impact on IT in order to push expertise into those parts of the business to drive up those skills to get those folks delivering. There’s a lot more to this than the tech side of it or the architectural arguments. If you think about it, when you build software, how frequently do you see a vendor coming out with a new release? It’s almost on a daily basis. Sometimes even shorter than that because they added a new microservice or pop this one on and pop that one in.
Their ability to continually deliver more functionality is there. The same thing is happening in data now, which is, “Can we break this thing apart and start building components?” Ultimately, it is going to end up with people getting faster in delivering because 60%, 70%, or 80% of what they need is ready-made, and they don’t have to go build it. That’s the way I look up on this.
[00:14:10] Eric: That’s a tremendous overview you got there from Mike Ferguson. We have a couple of great questions already from our virtual audience coming. If you want to be in the virtual audience, hop online to DMRadio.biz. You can register to watch these live and get your questions answered. Adrian Estala, I’ll bring you in from Starburst. Mike did a fantastic job there, giving a whole description of what this thing is, where it’s coming from, and what it is doing.
It’s exciting because we do have the component parts from a technological perspective to start creating these things. To Mike’s point, there is a huge impact on organizational hierarchies and how you do things. We see lots of examples of how we learn lessons from DevOps. I was at the Snowflake Summit. A company called DataOps.live gave a presentation with Roche, a pharmaceutical company, about how they modernized their whole data processing environment. They went from one new release every 2 to 3 months to 120 releases in a month.
[00:15:17] Adrian: I’m familiar with their model. It’s fantastic. With what pharma is doing, specifically in the R&D space, they have some of the most mature data meshes that I’ve seen, and we can learn a lot from them. Roche has a nice one.
[00:15:32] Eric: You’re a former CDO and you talk to CEOs all the time. This is front and center for you. What’s your take on it?
When you build software, you see a vendor coming out with a new release almost on a daily basis. The same thing is happening in data. Share on X[00:15:55] Adrian: What’s different about data mesh is we start thinking about the skill side. There is hunger in our business teams and in our analytic consumption teams to do more on their own. They want to deliver, build, and learn the skills. They want to do it, so there is an urgency, a hunger, and a want. I’m not forcing change on them. There’s a need there. It’s amazing what happens when you give somebody the keys and the perception of speed. When you control how fast you want to go, it is pretty incredible.
The skills required for data mesh are from a technical perspective, at least. I always talk about a data mesh as an ecosystem. There are a lot of tools. You can have a Starburst sitting right next to Snowflake. We see that quite a bit. It’s an ecosystem when you think of a data mesh. Certainly, at least with what we do, there isn’t a lot of new skill required. There are skills that you already have. You’re not building new skills. You’re leveraging existing skills, and even better, you’re teaching consumers to do it on their own.
On the skill side, Mike, I think it’s a great point, but if you think about how you implemented it, you realize, “I’m not teaching anybody anything too difficult. There are things they already know.” On the other side of it, when we start thinking about the broader organization pieces, I don’t know that you roll out a data mesh to everybody all at once. What you do is you focus. This domain focus model is your focus on the areas that are going to create the value and you can build in a pathfinder, a quick data mesh, or a team in creating immediate value. You don’t have to do it for the companies.
[00:17:37] Eric: You always want to get a quick win. You have the big picture in mind. You know where you want to go, what the desired outcome is, and use some point in the future, but you must start small. You must prove it out and work out the kinks in the process. According to your organizational culture, you’re going to find different roadblocks along the way. I’ll throw it back over to you, Adrian. Give us that 30,000-foot view you were talking about what data mesh means and how it’s for the business, not for the technical side.
[00:18:43] Adrian: It’s something Mike said earlier that resonates. You hit it right on, Mike. You talked about it’s about time to value. When you think about time to value, that’s what the business cares about. They don’t care about where the data came from and the pipeline. They don’t want the excuses about metadata KPIs. They want to get to their data, so that’s important.
Before we dig further into that, let’s start with that 30,000-foot view. If anybody wants a book, we offer a free digital copy of the inventor of data mesh, Zhamak. You can get a book and read the details. When you read the book, you’ll realize there’s a lot there. It’s a great vision, but nobody has implemented the book yet. Most of us are still in the early maturity phases. Even some of the most mature data meshes I’ve seen, they haven’t gone all the way to a fully federated computational governance, the way the book describes it. We’re not there yet.
I like to describe it in three steps. What I’m seeing is based on everybody that I’m talking to. The first step of any data mesh is the idea and principle that you’re leveraging the data where it is. If you’re building that data mesh and somebody is saying, “Let’s migrate your data here,” not sure that’s the right approach. There may be reasons to migrate data, but you should not be migrating your data as a design point for a data mesh. Data mesh, number one, leave the data where it is. You want to access the data where it is. That’s your first point.
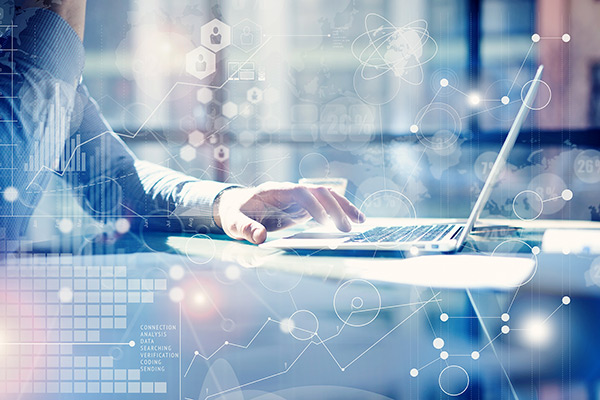
Data Mesh: Data mesh is an ecosystem. You’re not building new skills. You’re leveraging existing skills and, even better, teaching consumers to do it on the ground.
For a lot of companies that are building data meshes, that’s the first big win. I’ve seen data meshes where they show it to me. That’s all they’ve done. All they’ve done is connected to a number of these centralized services. Somebody asked a question about data fabric. A data mentioned a data fabric is similar in that first step. We’re connecting the centralized sources. It’s a huge win for a lot of companies.
Companies that choose to take the next step realize, “Not that I’ve got all this data and not that I don’t care about the sources, what I care about is the consumer.” We’re taking a step forward. Data fabric and data mesh is about connecting. Data mesh takes the next step and says, “Let’s organize all this data in a way that my consumer understands it.” I like to say, “Get the architects out of the room and bring the consumers in.” Do you want everybody in the room, or do you want to listen to the consumer?
If you’re talking to a finance team, you’re talking about the finance domain. If you’re talking to a merchandising team, you’re talking about a merchandising team. Maybe you’re talking about anti-money laundry if you’re talking about an AML domain. You want to organize the data in a way that makes sense for the consumer domain. I’m not moving or migrating data into a domain. It’s a logical representation. You want that person to come into the room and say, “I’ve created a domain that makes the most sense for you, for the types of analytics and the types of business problems you’re solving.” That’s step two.
Step three is the most important piece because once you’ve got them working in a domain, you want to start to create data products. There are a lot of different types of data products. Mike, you brought up some good points about treating data products as a microservice. I had that same one that thought early on. What I see more and more is I do see some data products that are stable. You work with a team that they’re driving the same kinds of analytics all the time. We’ve created data products for them that are reusable and interoperable. There’s a consistency and a quality to them. It’s fantastic, beautiful.
I have other teams, and this is where I think the real magic or data meshes, that the teams that are driving the real value are the ones that are discovering. They don’t know what data products they need. I always say, “There’s no such thing as a perfect data product.” When you build it for someone and you show it to them, we’ve all been there from a data side. You show it to the business, and they say, “That’s not what I want. What’s missing?” The magic is when they say, “Can you add this table? Can you get rid of that table? Can you add this other source?” You do it right in front of them. Is that what you wanted? That’s magic.
[00:22:47] Eric: This is part and parcel of what we see in the DataOps space because we have DevOps. We learn a lot from that. A lot of the innovation we’re seeing in this regard came from the positive results we got from DevOps. First, you used to have this big IT business divide. The data was there, but it wasn’t a first-class citizen. With DevOps, what do you have? You’ve got developers working directly with the operations people to solve problems. What happened is, why do they get a lot of great stuff done? I’m like, “We forgot about the data side. Let’s focus on that.”
We’re going to be a maturity around how we manage data. The guy from a DataOps.live said, “Do you want anyone to be able to do a manual update in here?” The answer is no. “Do you do manual testing?” No, you’re not going to do manual testing in this environment. It’s all going to be automated. That’s one piece of the puzzle here.
It's amazing what happens when you give somebody the keys. The perception of speed when you control how fast you want to go is incredible. Share on XThe point being is you want to automate as much as possible to the point where you can drag and drop these things to your comment there to create the magic. Magic happens when someone realizes something. When someone goes, “We call the a-ha moment.” If you can work with your business leaders or even your frontline business folks, and right there in a session, make these changes, that’s a huge deal, Adrian.
[00:24:14] Adrian: All the CDOs and data governance people in the room or reading this are saying, “Adrian, you’re making a lot of assumptions. You’re assuming we have great metadata, data quality, and access to all the data.” Those problems don’t go away. As a CDO, I’ve been there. I’ve been trying to fix data quality across a lot of different data sets. It doesn’t matter how many contractors you bring in to fix that problem. You’re never going to get there.
Change the lens. If you’re now starting to focus on data products, and you start to focus on the quality, metadata, governance, and access to data products, you’re focusing your efforts. You’re applying the efforts to create the greatest value, and your business will notice the results. We still have to do all the things we’ve been talking about, but now we’re starting to focus on the areas that matter most on the forehand.
To your point earlier, Mike, once you create it and you get it right, now you can start to reuse it. In the discovery phase, you’re going to discover bad data. You’re going to discover data that no one understands. That’s part of discovery. The fact that you’re now able to access it and your data product owner should be tied to the consumer and business. I’m an IT person. I may talk bad about IT people, but when you bring a business person into the room to work with their own data, they understand. They’re like, “I know what that is.” They can help you with the metadata and fill in the blank. That’s pretty powerful.
[00:25:35] Eric: A whole lot of pieces have to come together correctly like Mike was saying that it’s like a jigsaw puzzle. There are some concerns around data silos. We’ve got a number of questions here from the audience like, “Aren’t we going back down the old world of data silos?” To a certain extent, you are, but nonetheless, you’re trying to change the role of the IT person. I heard someone say, “From gatekeeper to a shopkeeper.”
In the old days, you were the gatekeeper primarily because IT was worried about keeping the trains running on time. There’s this fear of like, “Don’t touch it, it’s going to break.” Now we have a much more robust infrastructure. You’ve got infrastructure as code, for example. All the underpinnings have matured to the point that we’re going to stop genuinely. It’s almost there, or it’s certainly possible. What do you think, Adrian?
[00:26:27] Adrian: Let me talk about how people are implementing it. That’s something that a lot of people are curious about. We think about big transformation exercises, how painful those are, and how difficult those are. We’ve been doing them for a long time. You mentioned DevOps. We have some tools that give us an advantage and agile working. We’re working in Sprints. We have a DevOps model. We’re building iteratively in terms of MVPs. Those give us an incredible benefit to start small and iterate quickly. The way that I see implementation succeed is I call it 90-day Pathfinder.
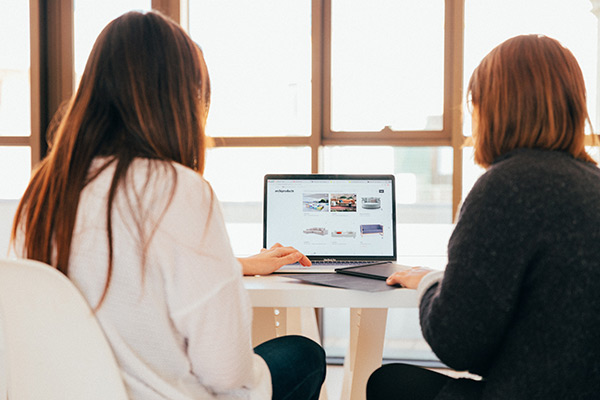
Data Mesh: There is no such thing as a perfect data product because when you build it for someone and show it to them, there’s always something missing.
In 90-day Pathfinder, you’re focused on three things, building a data strategy, implementing an MVP, and enablement. You’re training a small set of users. The train is important. You can’t build technology without training a data product owner, a domain owner, and a consumer. What we do in that middle phase and that implementation phase is two parts. One thing that I would recommend to everybody is to do a fast pilot.
It’s going to take you a little bit, depending on what you’re implementing or whether it’s Snowflake or Starburst. We’re back to say, “We’re implementing faster.” Depending on what you’re implementing, it’s going to take a while to get that implemented as you go through all the architecture reviews and InfoSec reviews. You don’t want to wait till the end of that to bring the people into the room.
What I like to do is do a quick pilot. Give me two weeks. Let’s get a light product installed. Let me ask you for a couple of data sources. Now we get that, and it’s all quickly. I bring the consumers into the room right away. By the second week, I’m working with consumers, and I’m showing them data products and a domain.
I’m getting them used to how this works because it’s not the technology that’s going to impress them. It’s the data that’s going to impress them. You want to understand how to use data. You want to understand where they get data from. We want to understand the questions they have, “Can you add this? Can you add that?” If I’m doing a 90-day Pathfinder, I want to spend 60 days with the consumer teaching them, working with them, and adding value.
The outcome of that is an MVP. It’s a production version of a data mesh with maybe 1 or 2 domains with 6 or 12 data products that other people would come in and say, “You demystified it. That’s how it works. That’s how you use it.” I’m not trying to shortcut all the other governance issues to forget the deal with, but once you create that momentum and people understand the value of beta products, then they’re more willing to come in and say, “Let’s work together on the governance and the other piece.”
[00:28:55] Mike: It’s not all sweetness and light here. Let’s be clear about this. You can’t say, “Let me have a data mesh deal here.” We’ve been through this before. If you go back to the 2014-2015 timeframe, we had this whole wave of self-service data prep. We had a whole ton of these self-service tools emerge, sold into the business, BI tool vendors out of that capability, and everybody was sitting in front of tools or they could connect to data and prep whatever they wanted to do. The problem that happened there was because of the Wild West.
If you thought you had a lot of silos after that, we ended up with even more because nobody knew what anyone else was creating. I can always remember a quote from one of my clients when we did a review, and the CDO said, “Everybody is blindly integrating data with no attempt to share what they create.” It was true.
Businesses don’t care about where the data come from. They care about time to value. Share on XPeople were using a multitude of tools and building their little world. There wasn’t any vehicle to publish this anywhere. There are an awful lot of assumptions out there. We’ve got lots of different tools. People can vote whatever tools they like, and we can share stuff. This isn’t about sharing data. It’s about sharing metadata. The problem that I see is still, in all my career, there is no industry standard to share metadata between any tool or anybody.
The assumption that you can put the metadata up here, it’s all self-discoverable, with any tool, you go in there and get it. It’s not true because there is no standard to share this stuff. There’s a big argument going on here at the moment, which does bring in that buzz phrase, data fabric, “Should I go and give tools to everybody? Let them all go and buy their own tools, build data products, and they’re part of the business. I put them all up there and share. It’s all going to snap together.”
[00:31:14] Eric: If you give him one tool, Mike, and I would say, “Build your data products here,” I would be very careful.
[00:31:20] Mike: A common data fabric approach, a common platform to do this on at the moment, is a more practical option because of the fact that if everybody is on this, they can share the metadata. Whereas if everybody is off buying their own tools, we’re going to end up with the inability to see what everybody is creating.
[00:31:50] Eric: At the end of the day, in your ecosystem, we’re getting to where they are. They may create data products that are a part of a journey. The challenge we have right now is that the businesses are not willing to wait. You can’t say, “Give me two years to build this right.” We need to go in now.
[00:32:06] Mike: The problem for me is what do you provide to the business side? On the technical side, what you can be offering. It’s always a negotiation. Who can need who? If you can get some pilot project to show the demo like, “This is what you can do and convince the business sides.” We can have some chart pack of knowledge to show. This way, we can virtualize this data or provide data availability.
I feel like we are at a different age right now. This is perhaps the opportunity for us to enable a better data fabric or data mesh. I do believe also we’re not mature enough on the technology side to implement the full feature of data mesh, but I think we’re getting there. I want to share is a little bit of story here.
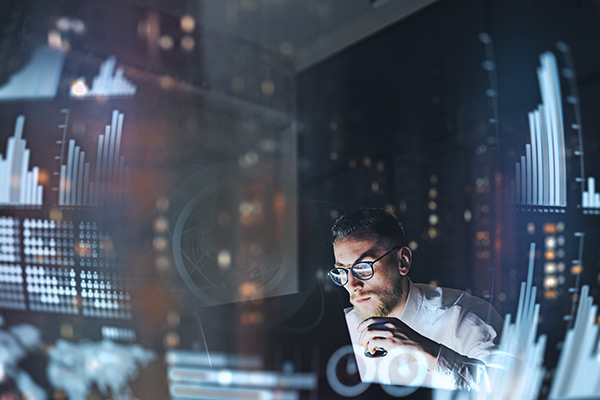
Data Mesh: You can’t build technology without training the data product owner, domain owner, and consumer.
I’m talking to a user who is using Datablast. Nowadays, you can create a data product easily on public cloud providers. It’s easy for different teams to create data silos without company-wise rules or some enforcements. It gets many silos easily. Previously, you perhaps bought a device with five different services. Now it’s like, “I’ve got to get a credential.” It creates a service there. You naturally get in the US and maybe some other states.
[00:34:07] Eric: We’re having a lively discussion about data mesh, and for a good reason because it is trying to weave together all these practices that we’ve learned. Mike Ferguson made a great point. I’ll throw this over to Bin Fan of Alluxio. If you want it to work, you have to reduce the complexity of the business, but you have to solve that. Simple is hard. If you want to create something that looks simple, it’s complicated underneath, and almost always. Bin, tell us a bit about your take on this and your role from Alluxio. These folks did something interesting. Tell us what you’re working on, Bin.
[00:34:46] Bin: It is like what you mentioned, “Making simple is hard.” That’s what we’re working on right now in Alluxio. I want to continue my example. It is a little story I was sharing. The user is telling me to find the actual. Different teams’ complaint is creating different data silos even in all one single cloud. Different teams are buying different speedup products in different regions. There’s also an acquisition from the big corporation, and they will see integrated data products from other teams.
In that case, it becomes a messy situation. You have all kinds of different tables in different locations, and there’s a lot of data duplication. It’s hard for them to discover who can carry what. At the end of the day, they come up with a solution or at least a proposal. This is the central data lake or central region. I should move all my tables there.
This simplifies the architecture to some degree but also creates other problems. You have to make the pipelines to create data. They migrate data from one place to another, and this creates latency costs and inconsistency. You may have seen a delay before you fully migrated the latest copy of data from the original data products to the central data lake.
In this way, how can you make sure you see all these benefits? You have all the data in different places. We’re working with them, and we’re saying, “How about let’s create a virtualization layer here for your data in different places, regions, or data products.” You can assume that this virtualization layer creates this housed metadata, but it’s more on the file system level or on the object level.
With this virtual layer, you can access data in different places. I assume it’s fresh or updated enough depending on if you are willing to pay to maintain consistency. This then creates a lot of flexibility and ease for the maintainers in that central because they can assume, “This is the view I have. I can go to different places.” You can have some surgeon machinery there to guarantee the data is updated enough, or maybe there are certain tables that are hot. Oftentimes, this is the case.
Everybody's blindly integrating data with no attempt to share what they create. People are using a multitude of tools and building their little world. Share on XWe have 20% of data that takes more than 50% of the traffic. For example, this is a residence or close enough to the applications. You don’t have to repeatedly query the data and move the data again. After the discussion, they are decided to go this route, and they are implementing this in production. They told me that this gives them good flexibility but also a reduction in their costs. More importantly, it simplifies their minds. Having this simplification is important for them to reason next to the staff and what we can do more based on this action.
[00:38:39] Eric: The concept itself of a data product signifies maturation in this space because we’re moving beyond old databases or things of this nature to a more refined consumable product. That’s what Adrian was talking about. If you talk to your consumers, “What do they want? How can you help them solve problems?” The mobile paradigm changed a lot of our perspectives about how to design user interfaces and workflow.
That was the key, in my opinion, because when you have this small device now, you don’t know a lot of real estate and you have to think carefully about what are the possible options at any step along the way. That’s the flow of screens or whatever. To make it consumable, you want to make it as simple as possible from a UI or UX perspective. That’s where I think data mesh shines when you get that UX right. What do you think, Bin?
[00:39:42] Bin: To make this more consumable is important for the users and for us. If we can provide this virtualization that is not only on the UX or UI level, it’s on the metadata level, at least for the file system level metadata. This is a logical space you’re accessing. This can make this thing easy to port to different environments or to different data products.
For example, I worked at Google. In Google, there are all the data, tables and articles. You have one single unique logical space for that. Assume this is a global database and you always go there. This can be in different data centers across continents. It can be in different media. Some of the data is on tape. Some of our data is in memory. As long as this is in thing space, you have a single and logical space address to access that, and this simplifies everything from writing the pipeline perspective or writing a business launch perspective.
[00:41:10] Eric: Alluxio has done some fascinating work in that space. I would recommend you guys look them up online, specifically with namespaces, and be able to initially leverage the power of cloud computing on your on-prem data. You create a fast connection of the virtualization layer to be able to get all that data accessible to cloud compute, which is a big part of the story these days. Cloud, you have scalable compute. Scalability is huge these days. You’re helping create a bridge between these two worlds and focus on the value to the business.
[00:41:51] Bin: We’re building this virtualization layer. We’re building this logical space on the file system. The admins, regardless this is in the cloud or your only own data warehouse, as long as you can put them into this logical space, your applications can go do something like, “I’m accessing this logical file and logical directory.”
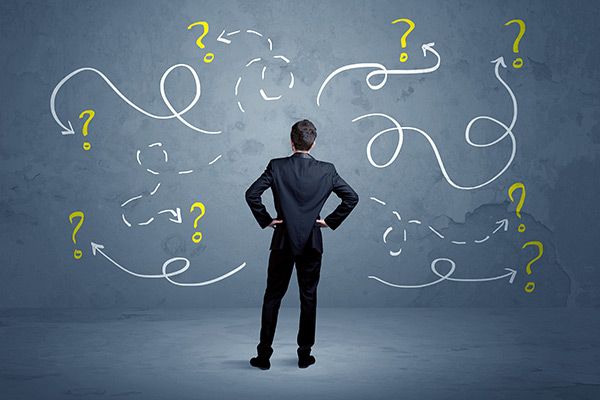
Data Mesh: Don’t try to solve everything yet because the data mesh model will mature. Your needs will evolve, and there are lessons you need to learn from the people and process.
In the upper layer, if this is a metadata service or something like high metadata, this can be made up as a table, a database, or something like that. Data product becomes easier to maintain and involve because what we see is decoupling the data of consumers, which is important for both sides to evolve faster, quicker, and more scalable.
They are for sizing on different parts of the scalability part. To me, that is interesting. I have my degree in Computer Science. Having another indirection, or sometimes you can use probably the virtualization of abstraction, is always the most interesting part because, with abstraction, you can do more stuff behind reconstruction.
[00:43:19] Eric: There’s an old adage in the IT world, “One new layer of abstraction can solve all our problems,” at least for now. We learn something else, and it goes around. It is exciting stuff. It does represent the maturation of our industry. We’re at the beginning of being able to pull off this data mesh, and it is all about the business value.
It’s all about opening up the channels between the business people and the data. You’re constantly working with the information and using it as leverage to make better decisions. We’re not lost in the conversation around the intricacies of the warehouse or how to fine-tune the indices and all this stuff. You’ll still have that depending upon your environment and need TVAs or IT people to manage things a lot more.
They’re going to be spending their time understanding cloud providers, what they do, and how does this impact our bottom line and performance? TVAs, IT, and admins, don’t worry. Your jobs aren’t going to completely disappear, but you will have to change what you’re doing and focus more on this new architecture and environment, which is a reality. It is not going away. Anytime soon, it’s going to have very long tails in that stuff. I would love to get a pitch on what to do next and why. From your perspective, let’s say you’ve got a client who clearly can use this technology. What should you do next, and why? I’ll throw it over to you, Adrian, with the pitfalls to avoid and stuff like that. What are your thoughts?
[00:45:10] Adrian: First, thank you to Bin and Mike for that awesome conversation. It’s super useful. It’s nice when you get real conversation. That was something real, so thank you for that. You have to make sure you have a need. Anyone out there is saying, “Data mesh is for everybody.” I like to say, “Start small.” What I mean by start small is focusing on an analytics team or a specific business function that truly has a need for it. There’s an urgency for them to start using and accessing data. I can get data all over the place. Perfect use case, I want to do more of it myself. Self-service is perfect use cases. Start small with a specific team, and move fast.
I certainly agree that we can’t solve the enterprise quickly. There are too many other data governance ponds around the enterprise, but you can move fast, solve and create an impact for one team. My advice there would be not to try to solve everything yet because the data mesh model is going to mature. Your needs are going to evolve.
Don't build a use case; build a showcase. Share on XAll the lessons you need to learn from a people and a process, you need a government perspective. You’re going to learn in that initial exercise at Pathfinder, but don’t build a use case, build a showcase, build something that’s real that people can say, “I’m using a production data product.” The magic of a data product isn’t building and walking away. It’s building it and changing it because the discovery is a real value that a lot of companies are driving for.
[00:46:38] Eric: That’s a great line, “Don’t build a use case, build a showcase.” I love these statements because that’s the elevator pitch. You get the point across in the elevator or walking down the hallway when you see the seniors. Bin, I’ll throw it over to you. You have a client, and you know what makes sense. What’s your advice on moving forward and quickly getting things done?
[00:47:07] Bin: To me, it’s important. I will always talk to my users and clients. I would like to emphasize a few things. This includes being an end venture of technology, being able to virtualize your data sources, and being able to bridge your data and also the data sources. This is important to think about and how you can achieve there. There can be different technologies, for example, in Alluxio technology. We are building a virtualization layer to help users and data products to achieve this. This is a complete feature, but at data mesh, it’s far from that. At least, to me, this is one key to making data mesh easier to implement.
[00:48:01] Eric: These virtualization layers, and these days, we’re getting good about knowing what data is hot. You see certain tables keep getting hit again. Maybe it’s a timely thing or a seasonal thing or it’s once a month or once a day. Whatever the case may be, you can watch those patterns, and that gives you clues as to what people are consuming. You use that activity and that behavior to optimize your design.
[00:48:26] Bin: I want to share a surprising data. This is one of the giants. They told me they are processing deployments. Fifty percent goes to a small set of data. In total, it’s tens of terabytes. This is one of the largest that’s internally good.
[00:48:57] Eric: That also helps in the governance process, with strategic planning, and understanding of the business. There’s so much that could be learned from paying attention to which questions people are asking, which data is hot, and which data is getting hit all the time. That’s what you work on. That’s what you prioritize because you can tell it’s of use to the business. I love this observability that we’re getting in the marketplace in all kinds of different ways.
Observability is the latest and hottest thing out there. It comes in lots of shapes, sizes, and different ways of doing it, lots of different types of visibility that we’re getting. It’s all useful for the information architect to understand what’s being built and how can I optimize the collaboration between all these different teams? Closing up for the show here, Mike Ferguson, take it away. What do we miss? What do we not miss? What are your next steps?
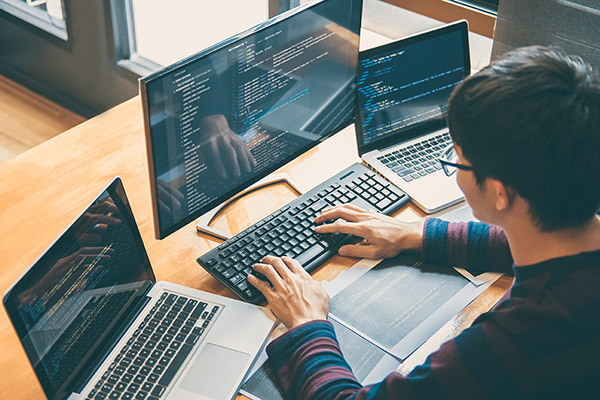
Data Mesh: The magic of a data product is in building and walking away. It’s building it and changing it because the discovery is a real value that many companies are driving for.
[00:49:53] Mike: You’ve got to think about some of the soft things. Organize this. This isn’t centralized. It’s not decentralized. It’s federated, in my opinion. I need a program office. I need to support business teams to help them upskill and get them fluent. I need a common platform to do this because the fact that there’s no standard for metadata interchange between tools, and you can’t exchange easily unless you have interfaces built between tools.
I need important technologies like data catalogs in order to discover what data I got out there. Even if I’m in a domain and I’m a specialist, what happens if my data sources are 40,000 tables in it. I need to know what’s under the hood. What’s in there? I need to know where my sensitive data is because if I’m going to do federated computational data governance, the domain people will have to take responsibility for sorting out sensitive data. They are not going to know where it is.
I need these kinds of technologies, and I need to take complexity away from business people. There are standard ways to do things and standard ways to publish data products. There are templates to get my pipeline’s going to produce data products. There are reusable components. They don’t have to build a whole pipeline from scratch. All of these things to help people be more productive and take the complexity away, automate the testing or CI/CD, all of that stuff.
For me, there are some critical pieces here of technology on the one side and on the organizational side. We need to allow multiple teams to get involved. We’ve got to harness it so everybody knows what everybody’s doing and align it with business to get real business value out of this. People will know who’s building what and for what purpose. You can prioritize who is doing what and in what order and people can see why you’re doing it in order to drive the value from it.
Don’t make assumptions. Think about industrialization. We’ve been through a decade of frenzy in development, and everybody is doing all kinds of bits and pieces, but execs now want this tie-dyed up, and they want to industrialize. They want to speed up the ability to produce stuff. If you’re going to speed things up in a production line or manufacturing, what are you going to do? You’re going to automate stuff. You want to make it as easy as possible and move stuff down the line.
If people are producing a data product for somebody else to consume further down the line, make it as easy as possible, do not say, “Let’s have agility. Everybody buys whatever tools they want.” We have a Wild West situation. Organize this. Make it a strategic project and take the complexity away, and maybe we will get industrialization.
[00:52:49] Eric: I love it.
Important Links
analytics Big Data Data integration Data Management data mesh data strategy