See What I Mean? The Power Of Visuals With Laurent Paris And Scott Masson
German Philosopher Ludwig Wittgenstein once wrote: “Some things cannot be said. They must be shown.” He was prophetically speaking about the power of visualization! These days, it’s not just data visualization that matters, but process viz as well.
To wit: the wildly popular orchestration tool, Airflow, which boasts 8 million downloads per month, is now being used by many organizations to map out a wide range of cloud and hybrid cloud scenarios. The broader trend line also shows classic Intranets and Web portals coming back, now serving as both process and analytics hubs. The goal is to synthesize views from across the business, even spanning multiple BI systems; and also enable front-line workers to take analytics-driven actions that help the business. Learn more on this episode of DM Radio as host Eric Kavanagh interviews Laurent Paris of Astronomer, and Scott Masson of Digital Hive.
—
Transcript
[00:00:41] Eric: I’m very excited to talk about a very hot topic. It’s always going to be hot and it’s expanding these days for good reason and to good effect. We’re going to talk about the power of visuals. We’re talking not just about visualizing data like in dashboards or in various graphs to understand patterns in relationships and things of this nature, but also the processes of being able to visualize the flow. Many of you know about Airflow, they’re up to something like nine million downloads a month. It’s one of the most popular open-source projects these days. We have a company here that sits on top of Airflow called Astronomer. We’ll have Laurent Paris and also my good buddy, Scott Masson from Digital Hive.
First, I’ll throw out a couple of things that are happening here in the marketplace that are very interesting. We’re coming up with new ways to leverage the assets that we already have. When you talk about Digital Hive, here’s a very interesting company where they’re able to consume assets from multiple BI tools like Tableau, Click or Power BI, and show them all on the same screen. It’s a very fast dashboard-like tool that you can build visualizations, but this way you can synthesize what’s happening in your organization.
You figure a dashboard is only ever a snapshot of time. You can have slider bars, for example, that show you over time how things change. I’ve always found those to be extremely valuable. Of course, time series databases are what enabled that thing, or some manifestation on top of data to allow you to see over time what happens.
It’s very useful to see things in motion and then to be able to stop at the inflection points. That’s cool stuff. Digital Hive does other things too. They allow you to bake in processes and functionality. We hear all the time about embedded analytics and data-driven or analytic-driven apps. Quite frankly, there’s a whole new space evolving around that right now, which is quite fascinating.
There’s a whole new space for analytics and AI-fueled/driven applications. We’ll talk about some of that too. There’s also this modern data stack. We have all these fascinating open source technologies that have come along and changed the game. Now we’re able to integrate across them in large part because of technologies like Airflow. With that, let’s bring in Scott Masson from Digital Hive. Scott, welcome back to the show.
I feel like we’ve come full circle in a lot of ways in the data world where we’re able to learn from the mistakes of the past and hopefully not repeat them in the future. A big part of that is being able to see the big picture. It’s not just one dashboard. You want to be able to see live data. You don’t want to be looking at the data necessarily from last month unless that’s the point. You want dashboards that are connected to live data, so you can see what’s happening right now in your organization. What do you think, Scott?
[00:03:31] Scott: I totally agree. Thank you for using time series as an example and not pie charts. There are way too many pie charts out there even to this day. I can’t guarantee you that we won’t repeat the sins of the past. Everything runs in cycles and we’re bound to run into errors with visualizations, the data stacks, and things like that over time. What’s interesting is that there are more and more data visualization tools and capabilities out there in the market.
We've come full circle in many ways in the data world, where we can learn from past mistakes and hopefully not repeat them in the future. A big part of that is being able to see the big picture. Share on XThe dream of standardization is gone. Every business unit seems to have its own tool. What really presents as a problem is trying to get that holistic view of your business. If you have operational data in something like IBM Cognos, your marketing data in Power BI, and your sales data in Tableau, that’s great for the business unit to function, get the visualization, and get a handle on their business. When you want to elevate the story a little higher to the management level or executive level, it’s very difficult for them to get that single synthesized view across all the business units without having to either have somebody screen scrape different images and create a PowerPoint deck for the management team or have the management team log into 7 or 8 different BI tools or portals.
It becomes unwieldy to use. That’s where products like Digital Hive serve a need because we actually take all the visualizations from these different platforms, bring them together into one cohesive view, and allow for that effective management of the organization.
[00:04:48] Eric: You bring up an excellent point here. We talk all about silos. Silos aren’t just information silos. It’s not just a data source, for example. There are process silos and organizational silos. The best businesses are those that enable the cross-team approach to solving problems. Meaning you want your finance people to talk to your marketing people, your salespeople, your ops people, and your administrative folks. It’s that cohesive view that’s going to let anyone not only understand what they should be doing next but figure out where they fit into the big picture.
If you think about the relay races that they do in the Olympics, that handoff is important because there’s one person running and everyone else is waiting, and then you’ve got to optimize that handoff. In the real world of business, it’s not so easy. You’re running on four different tracks all the time. You’ve got to be able to orchestrate that accordingly. Visualization is often the best way to do that, but it’s not a visualization of this view of the world or that view of the world. It’s this synthesis of views so that the human brain can understand that marketing leads up to revenue opportunities or it’s supposed to, for example.
There’s a bit of a lag time there. Marketing puts the message out, you’re gathering leads, then you’re handing those through your salespeople, and then they follow through. You should be able to look at what our marketing efforts were last month or what our sales efforts are this month. There should be some correlation between those two, but you have to look at it and understand it. That takes time. It takes human power to look at and absorb things. The easier you can make that for the decision maker, the better off they’re going to be.
[00:06:27] Scott: In your analogy of the relay race, there’s very little that goes wrong in the running part. If you think of that in the business world, that is your business unit operating on its own. That handoff is that collaboration between the different business units. In the business world, at least from what I’ve seen, that’s where things go wrong. Data is misinterpreted, people are questioning the numbers, and they don’t trust the data because they’re all working off of different data silos. When you actually put the data together, that helps to bridge some of that communication gap because now everybody is seeing everything almost in black and white, but the various colors of the visualizations are right in front of their faces.
One of the biggest challenges that we see is the bias when it comes to visualizations. It has been around forever. It’s nothing new by any means. There are three different types of bias when you’re looking at visualization. First off, at the tip of the spear is the leader bias. Every business unit or business leader is going to bring in their own bias based on where they sit in the organization and their personal experiences.
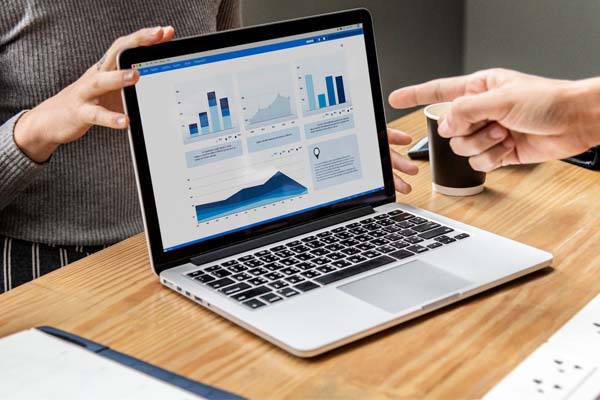
Power Of Visuals: There are more and more data visualization tools and capabilities in the market. The dream of standardization has gone. Every business unit seems to have its own tool.
There’s the author bias. The numbers are the numbers, but the author has the opportunity to skew the storytelling of a number based on how they create a visualization, the taglines they associate, the headlines or the captions. There’s author bias that can creep into there. One thing that is definitely harder to identify, especially from a business user perspective, is data bias. How is the data being gathered? How is it being treated? How is that ETL process happening? Do we have the same set of filters in the marketing group as being applied to the sales groups? When we bring those data together, do they actually mesh properly because all the underlying technologies have been synchronized?
The real challenge I find is that data bias or solving the data bias problem because the business user and the end user are essentially blind to that whole data capture process and the governance around that. You’re trusting your IT group or your CIO to make sure that data is properly governed and collected properly.
[00:08:13] Eric: The other thing I like here is that you’re facilitating cross-departmental communication. I think you made a great point there in the relay race. It’s almost always in the handoff that something goes wrong. This is why context is so important in every conversation. I think people forget how important context is until you’re out of context and someone throws a command at you and you have no idea what to do. You’re like, “What are you even talking about?” It’s because you didn’t have the context. This is the importance of training. This is the importance of buzzwords like empathy that people throw out there all the time.
A lot of times, people just need to be told. They just need a piece of information that they didn’t have because a lot of times people make assumptions about things. I think about when I used to do the layout for advertisements in my former life. One day my boss goes, “I thought you pushed the button and it did that.” I was like, “No. We were talking about logos that you have to size individually and put into the logo section of a big print ad.” That takes like 1 to 2 hours or even more if you’ve got twenty different logos to size, move around, adjust, pivot and all this stuff. It takes a lot of time. There are no tools that dynamically balance that stuff out. There was no such tool, at least not back then.
A lot of times people don’t know what the process is. If you have some visualization to look at to show where all the numbers are, then you can sit down with these people and say, “Let me explain how we get that data. Bob pulls it from this system. We cleanse it over here and here’s the pipeline. We deliver it over there.” Once people know, there’s a much greater appreciation for how much work goes into every different part, but you have to make sure and you have to ask questions and tell people stuff.
[00:09:52] Scott: Organizations have taken great pains and great financial efforts to solve some of these problems. You talk to me about collaboration. Especially post-pandemic, we’ve never had more collaboration tools in an organization as we currently do. Are they properly executed? Are they properly leveraged? I guess it’s TBD, but I don’t find the collaboration now between business units any better than it was pre-pandemic. We’ve invested in all these collaboration tools and I don’t think they’re solving that intercommunication gap.
That aside, you’re talking about quality data and trust in the data. I see organizations spending a lot of money on creating metadata catalogs and having metadata repositories to enhance and add value to the actual visualizations and the group reports to give the user that level of confidence and trust in the data. Where they fall short is the metadata repositories don’t sit side by side with the visualizations. Unless the user knows that they have to go leave Tableau and go to Collibra or Informatica to find that metadata, they’re not going to do it.
Every organization talks about being data-driven, but in the absence of trustworthy data, a user will either make a gut call or resort to their own personal data sources. Share on XWhat we’re trying to do at least within Digital Hive is when you have a visualization there, there’s actually a way to launch into the metadata that’s associated with it. If there are questions about the data, you can pop open the information button. We’ll show the business glossary or the metadata values, whatever you deem as being necessary to help instill trust in your user community. Bringing together the metadata and the visualization is imperative for not only understanding the data but also making sure you have trust in the data.
Every organization talks about being data-driven. It might be out there but for users, in the absence of trustworthy data, they’re either going to make a gut call or they might resort to their own personal data sources. You’re taking Excel and munching the data. All of a sudden, your users have gone rogue because you’re not basing your decisions on any factual corporate information. It’s gut feel or personal data.
[00:11:42] Eric: It’s a great segue to bring in Laurent Paris from Astronomer. Astronomer sits on top of Airflow, which we were mentioning nine million downloads a month. It’s a very popular tool. Laurent, what I love about your approach is that you’re tapping into all of this observability technology these days. To Scott’s point, when you’re looking at a flow of data across some set of touchpoints, you can hover over that touch point and see the relevant metadata perhaps, or other reporting data that will tell you what is the latest thing that’s happened. That’s beautiful because it’s a multi-dimensional view of the world.
[00:12:23] Laurent: I like what Scott said because the problem is the dashboard is the tip of the iceberg. That’s what people see. Beyond that, there’s a whole pipeline that is policing the data. People are asking, “Can I trust how that data is being consented to?” Having that observability end-to-end from the ETL process down to the dashboard, understanding where all the steps are and where the data was being transformed, and understanding what was the quantity of the data that’s received is like an assembly line. It’s like process management. You want to understand from when they tell you to when you end up with the finished product. It is important to basically understand the end-to-end approach. I think there’s an interesting combination where Airflow is doing the orchestration needs. It’s the one that is orchestrating the assembly line, but we are starting to build visibility and transparency on the process itself. People gain that trust in how that was approached.
[00:13:21] Eric: This is interesting too because by also showing the flow from start to finish, you’re educating people about what actually happens to the data and where this information comes from. As you have suggested and as Scott suggested too, if there isn’t a readily apparent answer about how something gets done, people make up stuff in their head.
[00:13:44] Laurent: It’s so fascinating because in most cases, the reality is it’s a black box. Everybody builds a mental map in their brain, except everybody has a default position of what is the actual flow. It’s like Google Maps in my mind. You see their reaction like, “I didn’t realize I was also depending on these.” I’ve always been amazed by the reaction of the head when he sees it for the first time like Google Maps objectivity because he had that set of assumptions. Some are true and some are wrong. It’s that a-ha moment that you had when you see, “This is amazing.”
[00:14:32] Eric: That’s how you can also problem solve, optimize data pipelines, and optimize processes. You get multiple people at the table and get someone saying, “You’re doing all this work to cleanse that data. We already did that over here in this golden record. Why don’t you grab this instead of that?” Until you have something you can look at and move around chess pieces on the board, it’s hard to map all that stuff in your head.
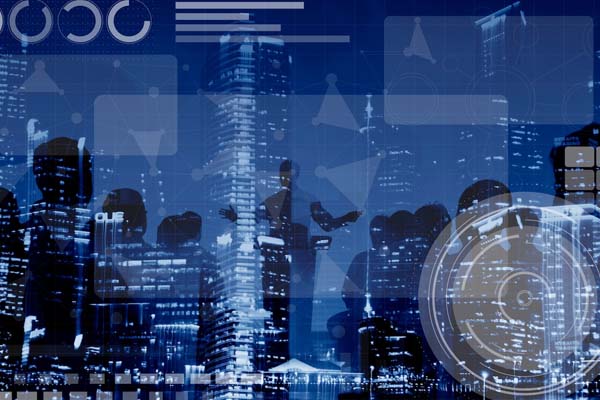
Power Of Visuals: Digital Hive serves a need because they take all the visualizations from different platforms, bring them together into one cohesive view, and allow for the effective management of the organization.
[00:14:59] Laurent: The failure to make it visible allows better understanding and optimization. Another thing that I was interested in your discussion with Scott was the collaboration between the team. Generally, a team are stealing their own domain, but the problem is in order of teams. For them, it’s a black box. That might be like a common language between teams and it helps collaboration. You can say, “I see my domain but I see also your domain and how you influence.” I’ve seen that as a tool that triggers collaboration across teams.
[00:15:44] Eric: I’m from the data world. I’ve seen ETL jobs and how you map them out. You pull it from this system, you do this transformation, and you load it into this system. Now we’re getting much deeper into the actual processes that run business. When you do the so-called digital transformation, what you’re doing is collapsing some multi-step process.
Usually, you’re taking some input. You want an output over here. How are we going to get there? You map out the different parts of the story. Now with something like Airflow, you can see what’s going on and you can share it with people. You can talk about it and start making some decisions. This is part and parcel of changing your business.
Ladies and gentlemen, we’re talking about visualization of processes. We got Scott Masson from Digital Hive and Laurent Paris from Astronomer. I love watching things in motion. I’ll throw it over to Laurent first because you were commenting about something that you’ve seen in organizations. There’s so much collaboration that can happen, but you need a mechanism of action for enabling that. For tracking it over time and knowing who did what and where. The more tools you’re bringing to the mix, typically the more complex things get. What do you see out there when you talk to enterprise clients?
[00:17:49] Laurent: The larger the enterprise, the more diversity. You have a bunch of tools and a lot of teams, and then the connection between teams, and then the connection between tools. You have the handoff. That’s where things break. You follow the leads. How do you guarantee a smooth flow of data across teams and systems? To guarantee that, you need to understand. To understand, you need to have that visibility. I call that sometimes Google Maps of that ecosystem. You understand the data flowing from point A to point B. It crosses five teams and it goes from an ETL process.
If I’ve drawn to a warehouse like Snowflake and then an enrichment machine in our process where we stack it, you need to understand how that swings across all of that. That issue made that visible to everybody. It’s like everybody is stuck to having a common language and says, “I understand. I am this piece. The downstream consumer is this team, but I depend on that upstream team and everything.” That visibility is key if we want to avoid all the silos that happen naturally in a large enterprise.
[00:19:04] Eric: What I love about this again is that until you understand in your organization what is upstream from you and what is downstream from you, it’s hard to appreciate the importance of your job. Once that is put into context, then you can see, “If we don’t get this piece of information for you, our marketing campaign is going to be 50% as efficient because we’re going to be spamming people who are uninterested.” We’re not being clever enough to reach out to the people who were interested.
When you make data visible, you make it useful and understand it better. Share on XThere are so many things you can do, especially on the sales and marketing side, to optimize your net results. You won’t get there if people above and below or on either side of you don’t understand the end-to-end process. That’s why I love Airflow, especially in this modern data stack world where you’re going to have all these other systems. In the old days, you stitch them together behind the scenes. That’s the whole point. It was behind the scenes in the black box somewhere. Some consultants stitch it together for you. That’s okay as long as it works until it doesn’t work. In this case, it’s hard to change and fix, but not if you have this schematic that shows you where it’s going.
[00:20:11] Laurent: To your point, the visibility of the process is important. What’s interesting is when you layer your personality on top of an orchestration layer like Airflow, you have interesting additional capabilities. It’s not just because you control that flow and you also make it visible, but also because you make it useful and you understand it better. You can then reuse that in optimizing the orchestration and the layer. It’s like a human being. You act and you perceive there is an action, and then you work from that, and then you include your next action. That’s why I think it’s the magic of combining Airflow with the deep layer of observability.
[00:20:57] Eric: That’s what got me excited looking at the interface because you’re pulling any given stage along the way. Let’s say it’s the ETL stage. We’re pulling some information out, then you got an enrichment phase. You got a targeting phase where it’s like, “We only want these few people.” You have maybe one final check or something like that at any given point in time.
In the old days, you didn’t know which one of these things went down. You had to go guess and figure out like, “Let’s try it again. That doesn’t work.” Think about how much time people are spending in problem-solving or troubleshooting. It’s not a fun thing to do. What you want to do is try your idea and see how it works. I usually do things through a marketer’s lens, what gets people to engage, what gets people to click, ask good questions or whatever.
In the old days, it was a black box. You didn’t know and had to try again and change this or change that. Now that can know, you can figure out, “This is on the ingest. We have to talk to our team that’s out there on the front end, pulling this information.” “We changed the form. There was a new web form that went out. That’s why we’re not capturing this data anymore. They were doing a promotion,” or whatever. The point is it facilitates the conversations that get you to the answers.
[00:22:13] Laurent: The person who was looking at the system at a high level can observe everything and try to optimize the process. I would say even the team that is a part of that process are getting that understanding of the consequences of their action. You got the guy who’s controlling the ETL process and you have no clue how your data is being used downstream. If I fail that, the targeted marketing and ad campaign will stop walking. You feel like, “I am struggling to achieve success.”
[00:22:58] Eric: I’ll bring Scott Masson in from Digital Hive. I was actually on a show about open source, and I came up with a metaphor that was pretty fun. It shows how old I am. I remember I’m old enough to remember Christmas lights on your tree. There used to be one wire that ran all the way through, which means it was itself the whole circuit. What that meant was if one light went out, the whole thing stopped working. You would have to replace every single light to figure out how to get it all to work.
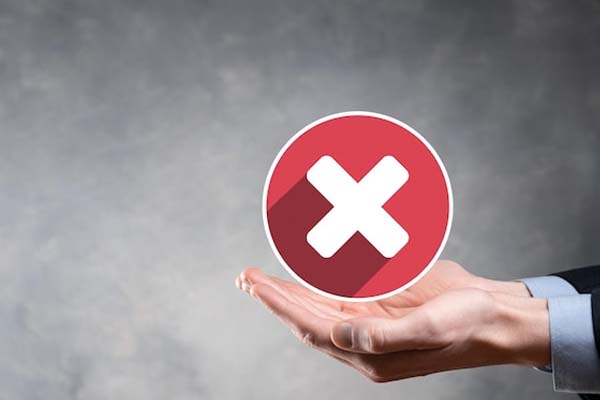
Power Of Visuals: Where things go wrong is when data is misinterpreted, and people question the numbers. There’s no trust in the data because they’re all working off different data silos.
They figured out, “Let’s have a separate circuit running through such that even if one goes down, the circuit still goes to the next one, and you replace the light that’s missing.” That’s a perfect analogy for observability. For being able to see, “That’s why this little widget here went down.” When you have that observability, the time you can save in troubleshooting is mind-blowing.
[00:23:54] Scott: I’m old enough to remember those light bulbs too. My dad used to say, “Screw it. Throw them out and buy new light bulbs.” He wouldn’t go through the pain of having to fix them all. I digress. The whole observability is huge. We hit on time savings quite often during the run section. What came to mind was that there is time sensitivity to all data. The fresher the data is, the more value it has. The value of data and time run on reverse curves.
Everybody strives to get real-time reporting. Real-time doesn’t exist because there’s too much transformation. Too many downstream activities have to happen. You want to get as near real-time as possible. Looking at that as the Google map of your orchestration, if that can actually save you time in troubleshooting or diagnosing problems or streamlining the actual availability of the data, that’s a huge benefit to the organization. Now they have a quicker turnaround time to get access to that fresh data.
[00:24:49] Eric: You solved lots of problems at that point. You almost don’t even notice until you sit down and think about it. You’re like, “That saved me half a day,” or something. This is what business people want these days. When they have technology conversations, what they want to know first and foremost is, “How will this change my day-to-day activity? What process is this going to streamline? How is it going to make my life better? Is it that I’m more informed when I’m making phone calls or is it I’m calling the right people?” These are all valuable because people sit around at their jobs. They do stuff all day. They take breaks or whatever, but as long as you’re on the right path, you’re going to be productive, until you don’t know and then you’re not productive at all.
[00:25:33] Scott: I go back to what I said before. If you don’t know either who to contact to get some assistance or you don’t know because the data is absent or not trustworthy, you’re left with your own devices. The results are going to vary. Most people aren’t going to sit there. They’re going to take some kind of action. In the absence of factual data there, I don’t know what they’re basing their decision on. As I said, they’re going rogue. They’re supplementing the gaps for themselves.
[00:25:57] Eric: I’ll bring Laurent back in. The other thing I think about a lot is the workspace. Where are you working when you’re doing your work? A lot of times you’re in your email, for example, or you’re in some operational system or with people who were designing these workflows. They’re working in Airflow or Astronomer or something like that. You want to be able to deliver the important information in that environment.
You don’t want to have to jump to some other tool to go add something up in your head. Even on simple stuff like sending up invites for these shows, I’m like, “I have to go over here. I grab the email address and go over there.” Google is making it a little bit easier. It’s very clever what’s going on under the covers at Google. This is basic machine learning. I’m not sure exactly how they’re doing it, but they’ve noticed that every time I email Lynn Moore, I’ll also email Scott Masson because they work at the same company.
When you create a visualization, a course of action should be formulated based on these numbers. Share on XThey recognize in the past when I emailed these two people, “Do you want to add these two people?” “Yes. I sure do.” That’s the value of AI and machine learning, but also automation. It’s baking in identifying processes that are repetitive and fueling the information to you. With Airflow, that can be the destination for all this observability information in the context of the flow, which is fantastic.
That’s where I go to understand, “It looks like this node crashed. That’s why we don’t have this information.” When it’s a black box, it’s anybody’s guess, but when you can see in there, you can get right to it. It’s almost like the new cars these days. The mechanic can plug right into your computer and look at it and go, “Okay, this is what happened.” It’s not even night and day. The difference is so great.
[00:27:40] Laurent: That actually is my dream and my vision for the future because you start with the orchestration layer. Because you are the one orchestrating, you have a deep understanding of what’s going on. The second step is the basic level of observability. You collect that information as you build that map and everything. These are not so complex that there are thousands of data sets with values.
There’s information from other people who would try to understand. The next stage is on top of that metadata over the machine learning or artificial intelligence, to start to extract signals from those. If you’re going to say, “I know all of that,” but as a human being, you should put your attention on that specific data set and that specific process because there’s an abnormal pattern. Something is wrong. I think that is the future of true observability. It’s focusing and showing that human beingness in a very complex ecosystem.
[00:28:48] Eric: It saves time and money. What’s great too is you can tell the person involved exactly what’s going on. It’s not a question of, “I think it’s you.” No, I know it’s you. I know that’s what the problem is. It’s an impetus on them. It helps you tell the story so you’re not having some argument about something. You can actually look at the data and say, “The feed is not coming through.” That’s what I love about computers. They either work or they don’t. It’s not like an opinion about some theory of how the world works. It’s a very specific practical thing that is functioning or not functioning.
[00:29:26] Laurent: You have a specific use case you promise to your customer. They don’t want to get that reactive when a process is failing, the data set that is wrong, or something like that. What happens generally is you have a root cause. Let’s say some data feeds starts that to be wrong. You start to have a bunch of downstream processing and all the downstream data sets start to get wrong there. The thing is you want basically the root cause being fixed on the front end than being told, “We are all aware there’s a problem. Don’t worry. We know who’s the team in charge. They are already walking on the front end. You have to wait until it gets repaired.” That’s the intelligence that you can deal with collecting all that metadata.
[00:30:18] Eric: It also helps you understand workflows and understand where the value is coming from. It helps you identify how to future-proof your architecture. If you see one time and this part is falling, maybe that’s where we put some investment and get a more robust solution for that piece of the puzzle.
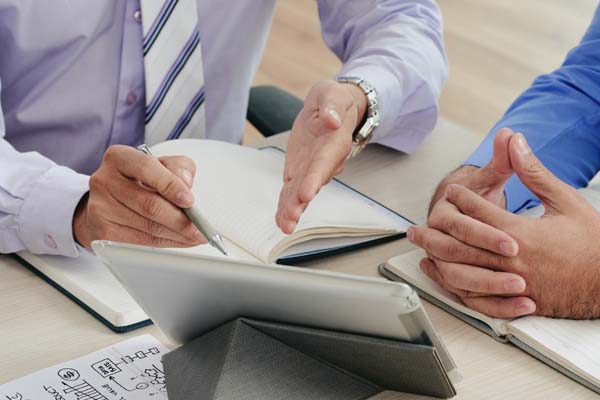
Power Of Visuals: Three different types of bias when you’re looking at visualization: reader bias, author bias, and data bias.
[00:30:42] Laurent: It’s providing an end-to-end use and extracting the information from it. I keep coming back to the same thing. Also, all of these processes help reduce tension between teams. One other thing we heard from customers is the analyst consumes that asset for use by the IT team or by the engineers. They have no clue who it was produced for. When something goes wrong, it’s a black box, and then you have that friction between the analyst and IT. The IT team is capable of saying, “Yes, we know what the root cause is. We’ll look at that flow and it’s impacting you. We are working on it.” It’s a common language.
[00:31:27] That’s a good point. We want to reduce tension and friction points between teams and keep people happy. That’s what we’re learning about. We are talking to Scott Masson from Digital Hive and also Laurent Paris from a company called Astronomer. I love that name. I’m big in astronomy. I love the visibility. It’s weird. I’m a digital marketer. I’ve been using email marketing for many years. I can tell you once you can see who opens and clicks, it’s almost impossible to go back in time and live in the dark.
Because you learn more and more about what’s working, now you have to optimize your processes. At least, you know what to do. I think that’s one of the keys here. I came up with a methodology, a simple one. It’s go APE on people. Check your Assumptions, align your Priorities, and then Execute. Do that again and again. There’s like the Odoo loops or these other things that are not new, but the key is you have this set of assumptions you walk into work every day. With technology like Airflow via Astronomer where you get all the access to the visibility and the observability feeds, it helps you know what to do.
I spoke at a conference and off the cuff, I realized there were two volunteer firefighters in the audience. That’s a lot in a fairly small audience. A firefighter is a great job because you know exactly what to do. You put out that fire. That’s what you’re doing all day long. Now short of that, there are all sorts of evaluations. You have to know what needs to be done now from a management perspective, what to ask people to do, and what reasonable amount of work to request from someone. All of this can be ascertained if you have observability and if you can work cross-functionally across teams to solve problems. I’ll throw it over to Laurent first. What do you think?
[00:34:06] Laurent: At the risk of failing, the understanding of the processes and the flow is the first condition in basically improving and optimizing your processes. Definitely, that’s harder. The business derives value by first understanding what are the areas of optimization. It’s a continuous improvement loop.
[00:34:32] Eric: I’ll throw it over to Scott to comment on that. What do you think?
[00:34:35] Scott: I totally agree, but I’d actually like to go back to a point you made. You talked about the marketing metrics and being able to see who open the email, being able to visualize that and report on that. You can’t go backwards once you have that data. You’ve hit the nail on the head with the challenge that a lot of organizations face around visualizations. They’ve created the dashboards. They’ve created visualizations with a certain expectation, and the expectations aren’t being met.
Collecting the data is one thing; knowing what to do with the data is another. If you don't know how to take action on the data you're collecting, that data is worthless. Share on XWhat I mean by that is when you create a visualization, there should be a course of action that is formulated based on these numbers. If the number goes up, what do I do? If the number goes down, what do I do? If the number stays the same. What do we do? What course of action do we take based on the movement of the numbers? If you don’t have an action plan on those metrics or on those visualizations, essentially, they’re just vanity metrics.
Nobody is taking any action. You sit there. In previous lives, I’ve been in board meetings. You’re sitting there with lots of visualizations and data. The numbers are going up and down, but no actions are being taken on them. What’s the purpose of us sitting here looking at these visualizations if no actions are being taken to either correct or improve on them? One of the things that we haven’t talked about in visualizations is the outcome and the actions that people take on top of their data.
[00:35:48] Eric: That’s an excellent point. What am I supposed to do next? As a marketer and as an ops person, where should I be focusing my attention? The more visibility you have and the more aware you are aware in the process this sits, the better off you’re going to be at determining either what to do yourself or what to have someone else do.
[00:36:11] Laurent: To complement what Scott says, sometimes you show data or visualization, but it’s only half of the problem. The other half is, how do I interpret the data? What does it mean? How do you derive insight into the raw information? What actions do I take based on these insights? Sometimes people stop to say, “I showed them a bunch of data. I visualized a bunch of things. I have the feeling that I‘m in control,” but if you don’t derive valuable insight and if you don’t base action on these insights, it’s useless in my mind.
[00:36:49] Eric: To get back to Digital Hive and your view of the world, that’s why it’s nice to be able to consume multiple analytic assets in the same window, but then also bring in some process information, some textual information, and maybe a video that talks about the parts of what’s happening. People learn it in a variety of different ways. There are certain things you can learn by reading the instructions. There are certain things you can learn by someone showing you what gets done.
The disparity in time between watching someone change a car part, for example, versus trying to read step-by-step instructions for changing the car part. Let me tell you, it’s a lot easier to watch someone do that than it is to type out prescriptive directions. Some little videos could be all you need to complete the picture to help the person understand. That’s why the marketing campaigns aren’t working. It’s because we can see from these numbers over here, etc. That’s the storytelling.
I was all excited about storytelling, but storytelling has to be connected to the data, which has to be connected to the skeleton of the process in order to figure out what gets done. To your point, Scott, take action, “Bob, we now know you have to fix this pipeline. Fred, we know you have to do this. Susie, you have to come up with some new marketing campaigns,” or whatever, then people know what to do. When you know what to do and you’re part of the process, that’s a very cool feeling. It’s good for morale. It’s good for the energy and the organization. That’s what makes good changes.
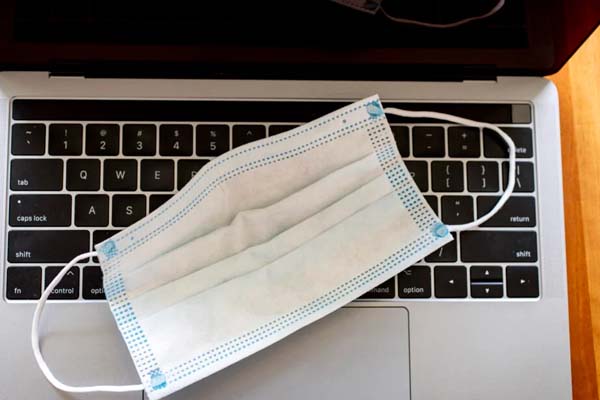
Power Of Visuals: Post-pandemic, we’ve never had more collaboration tools in an organization as we currently do, but are they properly executed and leveraged?
[00:38:21] Scott: Thanks for making the connection to the context of the data. That’s where I was driving, but you brought it around full circle there. That’s perfect. Being able to put the right context to the data is key when you’re trying to couple that with taking action on the insight you gained from the reports. A perfect example is in an executive management meeting, you might make a decision in Q1 that has an impact or won’t have an impact on Q4.
Marketing, driving leads and sales pipelines take a while. The decisions you make in Q1 aren’t going to automatically be seen in Q2. Come to the Q4 management meeting having the ability to have your visualizations there. Pop up the PowerPoint or the PDF or wherever your summary notes from the Q1 are. You say, “We decided to do A, B and C. Here’s the net effect six months later.”
Making that context is great, otherwise, you’re relying on people’s memories from three quarters ago. I barely know what we did last week. Trying to take the memories and the decisions that were made 2 or 3 quarters beforehand and make sure that you understand or you’re correlating that to your results in Q4 is near to impossible. Adding the context of the data is a key takeaway in all this.
[00:39:24] Eric: The combination of what you folks bring to the table is the key. Laurent, I’ll throw it over to you. Knowing what to do next and knowing what big undertaking to do depends a lot on the information on what you’re able to gather, and just the whole TCO side of the equation. What value are we getting from these assets if we’re not able to plug them into some business process?
[00:39:48] Laurent: I liked what Scott said. That process of extracting and interpreting the data, and the context is so important. I agree that context is a basic key in that process. To your point, part of the context is, is it even worth collecting that data? Does it lead to a decision that brings value to the business? Especially in the early days of data, we hear people say, “Collect the data and then we’ll figure out what to do with it.”
When you start to say, “Pick that data that I accumulated,” you say, “Maybe we need to be a little smarter about which data matters and which data doesn’t matter.” I think it leads to a notion sometimes of what the SLA s are or what the business is. You associate it with a piece of data that is being stored, processed, collected and everything. Part of the context in the metadata that is on the rise is actually maybe decorating a piece of data with, “Is this business critical?” “Yes, this is nice to have for research,” and have a different treatment of this piece of data.
[00:40:56] Scott: Just to add to that, Eric, I’m probably a bit of a data hoarder as well because I believe we’ll eventually have a use for it. To add to what Laurent was saying, collecting the data, having value, and knowing what to do with the data is one thing. As I said before on the vanity metrics, if you don’t know how to take action on the data you’re collecting, I’d almost argue that data is worthless. The face value is worthless as well if you don’t know how to act on that data.
The major factor for agility is the size of the business and the willingness of the business to accept risk. Share on X[00:41:20] Laurent: I’ve seen the evolution. I’ve seen some of the big data and data stacks. Before, “Let’s collect all the data and figure out what to do with it.” As Scott said, we need to start to give those away. What is the digital value, what are all the metrics, and which data do we need?
[00:41:46] Eric: I love what you’re both able to bring to the table here to help people stay on track, do the right thing, and make sure we know where we’re going. I used to have a manager. I love this. He would say, “Let’s achieve what we’re trying to achieve.” I was like, “That’s a good point,” because you do have to be open-minded about what you’re doing and where it’s going. At the same time, let’s not be random happen chance bouncing around out there and hope good things happen. There is a management-side to this as well, which is humility but also sternness. You have to be able to take complaints and deal with multiple people being upset about something for different reasons and say, “I hear you. Now let’s do this,” and come up with a plan of action.
I’d be curious to know what are the cycles these days. It depends on the company, but is it two weeks at a time? Is it a month at a time? Is it a whole quarter that you’ve mapped out? Any thoughts from either of you on like the sprints? In development, you have sprints. It’s like a week-long sprint or a two-week-long sprint. Where are we? What do we figure out? Where are the bugs? Where are the problems? Any thoughts, Laurent, on what’s a good sprint these days for business?
[00:42:58] Laurent: I would say it depends on the business context. Some businesses are operating quickly. Some businesses are operating a little slower for a good reason. I think that also values thought processes. Typically, a sprint has to be every two weeks. I was in companies where it was even every week. We start on Monday and we shift by the end of the week. That’s the evolution of the product. If you look at a bigger cycle, every six months, we revisit the strategy and the budget and everything. Twice a year, we try to have somebody figure out, are we on the right track as a whole company? We have monthly checking out the progress of the roadmap. You have different processes with different incomes is my analysis.
[00:43:53] Eric: That’s a good answer. Scott, I’ll throw it over to you. I don’t know even what our cycles are. We have annual cycles here, we have quarterly cycles, and then we have weekly cycles because we’re constantly trying to stay on top. We’re in the media business. It’s a bit harder for us because we have to be edgy and interesting all the time. That gets to be hard to do. For Digital Hive, what kind of cycles do you see and what kind of sprint length?
[00:44:17] Scott: If you’re talking about sprints for pure development, I think everybody works at 1 or 2 weeks. If you’re talking about sprints for the whole business and the direction strategy for business, it’s obviously going to vary based on the size of the business and the market or the industry they’re in. I look at a company like Microsoft or IBM, these things are ocean liners. It takes them a long time to course correct. Whereas as a startup, if you told me next week that we got to change your whole technology stack, I’ll have it done by next week. If we need to change our whole marketing, move off of Salesforce, and go somewhere else, we can do that. We can react quickly like that.
That’s the benefit of being a startup. You have that agility. When it comes to a company the size of Microsoft and you tell them that you’re changing your whole marketing process and sales process, that’s going to have to go through a lot of checks and balances to make sure it actually gets approved, then implementing that is going to be months, if not year-long process. The major factor for agility is the size of the business, the willingness of the business to accept risk, and what their tolerance for risk and missing the mark would be.
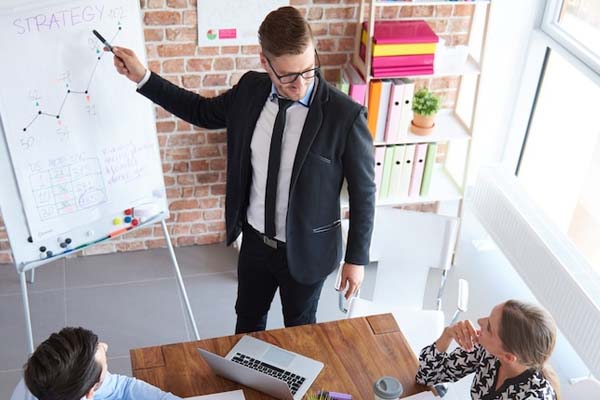
Power Of Visuals: Everybody strives to get real-time reporting. Real-time doesn’t exist because there’s too much transformation. Too many downstream activities have to happen, so you want to get as near real-time as possible.
[00:45:14] Eric: It has been fun talking to you, gentlemen. To our audience, look up these guys online, Laurent Paris from Astronomer and Scott Masson from Digital Hive.
Important Links
Apache Airflow Astronomer data visualization Digital Hive process visualization real-time reporting