The Next Frontier: Why Edge Changes Everything With Kris Beevers, Tony Craythorne , And Saimon Michelson
Cloud may be the new center of gravity, but the edge really does change everything: architecture, assumptions, workloads, and possibilities. You name it, and edge computing affects the end-to-end workflow of modern business. How can your company take advantage of this exciting new frontier?
Check out this episode of DM Radio to find out! Host @eric_kavanagh will interview several guests, including Kris Beevers of NS1, Tony Craythorne of Zadara, and Saimon Michelson of CTERA. They discuss how to deal with the constraints faced by customers out on the edge, the right way to handle moving data, and how AI technology transforms the current business landscape.
—
Transcript
[00:00:39] Eric: It’s a fun show, folks. It’s a very edgy show. That’s a pun. We’re going to talk all about the edge and the next frontier. Why edge changes everything? We’ve got an all-star cast. We’ll hear from Kris Beevers of NS1. We’ve got Saimon Michelson from CTERA, and we have Tony Craythorne from Zadara.
We’re going to talk all about what the edge is and why you should care about it. The edge refers to a variety of things. Your cell phone is part of the edge. Routers in a shopping mall are part of the edge. If you are in manufacturing, you could have some equipment way out at the edge. IoT is a big edge topic or The Internet of Things and all the different objects out there that are connecting to networks to let you know what’s going on in the real world.
The oil and gas industry, for example. A lot of edge computing use cases are out in oil and gas because that equipment is way out in the middle of nowhere. If you have these monitoring devices and these signals on there letting you know what’s going on and what’s changing, that’s a very useful thing. Edge does change a lot from an architectural perspective because we used to be on-prem. We knew what that was, and everything was on-prem.
It may have been complicated but at least we knew where it was, and then the cloud came along, the early cloud players. Salesforce was a big one. Now we have these hyperscale solutions, Microsoft and their cloud, Azure, Google Cloud Platform, and Amazon Web Services. We thought, “Now we have a hybrid cloud situation to worry about, how do we deal with security? How do we deal with moving data around? Where do the apps function? How much can we do in the data center versus in the cloud?”
Now, we have the edge. From an architectural perspective, you have a lot of things you can do. At the edge, you have to realize that sometimes there’s no great power or connectivity. These are pretty significant constraints to keep in mind. With that, we’re going to bring in our guests and go around the horn here. First is Kris Beevers from NS1. Tell us a bit about yourself and how you folks are dealing with the edge.
[00:02:37] Kris: Thank you, Eric. It’s great to be back again. This is a well-aligned topic for us. I am the Cofounder and CEO here at NS1. I’m an engineer. The edge topic for us starts way back long before we started this company, which is now almost ten years old. My backstory is building internet infrastructure even as early as the early 2000s. The business that I was engineering in before NS1 was a global cloud hosting content delivery and everything internet infrastructure company through that timeframe in the mid and late-2000s.
One of the things that we were building then was a global content delivery network. This is the kind of infrastructure that serves up cat pictures to you when you’re looking at them on the internet or serves the video to you that you’re watching on your streaming service or whatever. Even back then in 2007 and 2008, we needed to solve edge problems. We needed to put those pieces of content closer to audiences all over the world to solve issues around latency, the throughput of the content, and the reliability with which you could interact with it.
We also needed to solve a lot of problems around how you get the user to the right infrastructure close to them at the edge at the right time to interact with or engage with that content. We put a lot of work into that. The other thing that we were doing at that time was working with all of these up incoming web, SaaS, and software companies who all started to have the same challenge. They all started to realize, “My users are all over the world. They’re interacting with my code, data and content.”
All of it is sitting in Ashburn, Virginia, and some big fancy data center. I need to put the code, data, and content closer to those end users to have great engagement. That’s what led to NS1. One of the things that we do at NS1 is we are the DNS infrastructure for a huge chunk of the internet. If you type in LinkedIn.com to your browser, our job is to give you back the IP address and the right edge infrastructure for LinkedIn to engage and interact with to drive a great experience for you with that application.
We do that for a huge chunk of the internet. The reason they all work with us is all of them are operating now these edge footprints that are dynamic and very distributed. They need to solve this problem of getting users to the right code and data at the right time for those great experiences. That’s a little bit about what edge means to us.
[00:05:03] Eric: That’s fantastic. We’re going to pull on a lot of threads throughout the course of our show. Let’s get an opening statement from Saimon Michelson. Tell us a bit about yourself and what you’re doing in the world of edge computing.
[00:05:14] Saimon: Thank you, Eric. Also, thank you for touching on edge computing as an architecture because it really is. The way we think about edge computing is entirely different now with the cloud in play. A little bit about CTERA, we started back in 2008 and we anticipated that transition and new delivery of content and how we can improve how we deliver content across a widely distributed network.
Our background is also in the distributed network space. We dived into this data space and specifically solved the challenge of providing a ubiquitous file presentation that’s accessible through any number of locations. We’re identifying the gravity that data has. Data especially can be multi-petabytes of content managed by organizations. They’re dealing with the challenges of running out of capacity, providing highly available storage, and then providing that presentation across multiple continents, cities and states.
We’re doing that in a way that provides performance to our downstream users, applications, and devices, but at the same time, not compromising on things like reliability, security and availability of our systems. We’re very passionate about that part, and providing that native data protection. We also see this movement towards the knowledge age or what we call data analytics. Once you’re able to provide an architecture that provides that performance at the edge, you also want to maybe look at the data that you’re collecting and try to drive some additional value from it. That’s a little bit about CTERA. I’m the North American CTO for CTERA based out of New York.
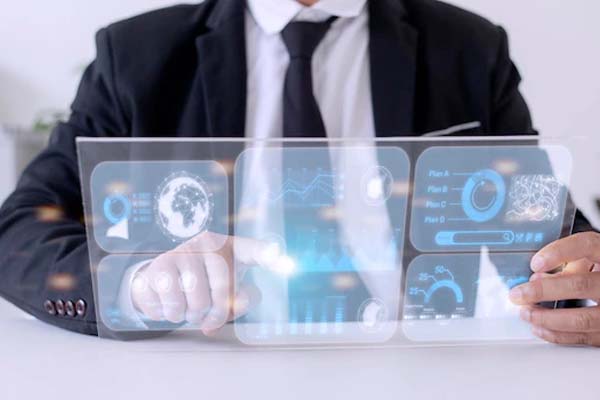
Edge Computing: Once you can provide a data analytics architecture that provides this kind of performance at the edge, you also have to look at the data you’re collecting to drive additional value from it.
[00:06:59] Eric: That means you’re an East Coast CTO. One of the funniest things I heard probably in 25 years of doing these shows was from George Corugedo from a company called RedPoint Global. He’s a super smart guy. He was getting all these calls from people trying to peel him away from his company. He was talking to one kid who was learning about him. He goes, “You’re an East Coast CTO.” He was like, “What does that mean?” He goes, “West Coast CTOs know one thing well. East Coast CTOs know lots of things.” That’s a funny observation about these CTOs. It’s pretty accurate too. You’re like, “I guess that actually makes sense.” Last but not least, Tony Craythorne is out there from Zadara. Tony tells us about yourself and the role you’re playing in edge computing.
[00:07:46] Tony: Thanks, Eric. It’s great to be with you. Edge computing is near and dear to our hearts because Zadara is the world’s largest edge cloud provider. We have over 400 locations worldwide. We deliver our network across a variety of MSPs and direct through our cloud. The company was originally formed around Storage as a Service.
In fact, the company was born as a cloud-native storage service, whereas everybody else has tried to shoehorn hardware to create a new cloud. Our business grew by actually designing a Storage as a Service and building our entire tech stack around that. For example, our file block and object are unified storage. You don’t need separate storage pools for file blocks and objects. You can use one storage pool and that gives you a lot of different advantages.
While COVID was happening, the company saw a need to shift towards a full stack. We acquired a very small company that had a fantastic EC2-compatible computing platform, and we integrated that. What was ours now is the world’s largest edge full stack EC2 S3 compatible edge cloud. We have 400 locations. We have hundreds of those interconnected. Our customers range from analytics companies to medical, state governments, and car manufacturers. Going third in this list means that you guys have already defined edge so I don’t need to do that or all the things that you talked about like latency security. One of the biggest things we offer is cost savings.
We are able to deliver a fully EC2 compatible full stack compute and storage without the cost of dealing with hyperscaler as ingress, egress fees, etc. We save our customers on average about 40%. We work with our customers in a multi-cloud environment. They’re on AWS. They have on-prem. We bridge the gap between the two. About me, I’ve been in service storage since time began. I’m the least smart of everybody on this call because you guys are all engineers and I’m a sales guy. I’m the Chief Revenue Officer, so I’ll do my best to keep up.
[00:09:45] Eric: Every company needs sales.
[00:09:48] Tony: I’m glad to hear you say that.
[00:09:56] Eric: I saw a hysterical meme. It was from John Daly. He is a big rough-and-tumble guy. It showed some regular golfing guy and then him. He’s dressed in all these colorful clothes. He’s smoking cigarettes and acting crazy. It said, “This is the sales guy.” That’s the guy all dressed up nice, and Daly was the sales engineer.
[00:10:21] Tony: They’re the ones that close the deal and do the selling. The sales guy is at the back, and then the SA and the SE do all the work.
[00:10:28] Eric: I love sales engineers because they can talk the talk, but they can also walk the walk, and they can usually go in multiple different directions. When you’re selling technology like this, and I’ll throw this over to Kris Beevers to comment on, there are so many factors to keep in mind. From a business person’s perspective, it can be pretty complex and bewildering to know where this one contract ends and this other contract begins, how we collaborate, and how we dovetail our security with your security. These are very serious questions, and everything is changing all the time. Kris, how do you future-proof your information architecture from a provisioning perspective and a security perspective? How do you guys handle that?
[00:11:15] Kris: A couple of immediate gut reactions to that question. I’m a vendor and I care about my customers and how they have to solve these problems. Step one for me is I talk to my customers. What do they care about? What are they trying to optimize for? What problems do my customers have that they’re trying to solve? The reason our customers are moving to the edge, and to capture it very simply, is they’re trying to provide great experiences to the people they care about like end users, their customers, and those audiences out there.
People are moving to the edge to provide great experiences to individuals they care about. Share on XFor context, our customers primarily are applications that you use all day every day. Streaming media, news, SaaS applications, those kinds of web and other properties, that’s who we’re working with. They’ve become more global and learned that their audiences, wherever their audiences are, have high expectations of any interactive engagement as we all do. We all hate it when the photo of the shirt that you’re looking at on a shopping website doesn’t load, the buffering icon comes up when you’re trying to watch a piece of video or something like that.
That’s what those customers ultimately care about. They’re always thinking about how are we going to progress those ultimate priorities in our business. Great user experience equals for them their conversion, continued engagement with their application, and so on. Also, they’re always thinking about how we are going to continue to increase the quality of the experience. Put yourself in a streaming media organization’s shoes. How are we going to support it next year? How are we going to support the next thing after that?
The volume of data is multiplying. The expectations of latency are always going down. Those are things that are pretty predictable for all these organizations. When they’re thinking about the future of their architectures, they’re thinking about the roadmaps with respect to increasing volumes of data, decreasing goals on latency or response time, and increasing expectations on the rate of change in the systems and on the expectations for the experience. Also, something that Tony touched on, how the heck am I going to pay for all that because that’s what isn’t going on?
[00:13:28] Tony: Cost is a major factor.
[00:13:32] Eric: It’s hard to know where the costs are going to be until you start doing this stuff. You have to rely on your consulting partners and the vendors when you’re talking to them to figure it out. One of the things that fascinate me most, and I’ll throw this up to you, Kris, is that out of the edge is like the early days because you have to be much more lean with your code. You have to be much more strategic. There are more constraints to worry about. I mentioned low–power usage or bad connectivity. You have to normalize this data and understand what it means if we don’t hear back, for example. The edge is a good proving ground for technology. If it can work on the edge, it can work in the data center and the cloud. What do you think?
[00:14:16] Kris: I generally agree with that. Start to paint a practical picture of some of this. In service of meeting the modern latency expectations and the increasingly connected world that we all live in, you talked about IoT. It’s an explosion of devices. I have 50-something connected devices in my house. If I go down to my local coffee shop, it’s full of connected infrastructure, from the point of sale to the IP cameras and the local retail analytics.
Tons of data are flying around more than it has ever flown around before, but the exact conditions and problems you described exist. There are 30,000 global coffee shops in some global coffee chains and they need to give you a connected experience, but guess what they can’t count on. They can’t count on deep computing and storage infrastructure in every one of those stores. They can’t count on consistent connectivity.
What do you end up needing to do? You end up needing to start to move smarts out to where the action is happening, where the data is being generated, and where it needs to be processed in real-time to provide you with that great experience. Many problems start to emerge when you distribute the code and the data more widely with less consistent connectivity and limited computing.
Those are all the problems that are shaking out now. The term I use is Wild West. The edge is the Wild West right now to the extent that we can’t even define the term. We used the word fog earlier when we were chatting. It’s a foggy term in and of itself. I tend to think of what’s happening. Edge is maybe not a great label. All computing, storage, and bandwidth are becoming more distributed and now need to be orchestrated to provide outcomes with these kinds of experiences we’re trying to generate.
Many problems emerge when code and data are distributed more widely with less consistent connectivity or limited computing. The edge is becoming the Wild West right now. Share on X[00:17:40] Eric: Saimon, I wanted to get you back into the conversation here talking about the constraints of the edge. It’s a very topic graphically diverse. There are lots of different things out there at the edge. Lots of different processors, boxes, machines and protocols. It’s being able to wrangle all that and understand the tapestry you’re working with before you start painting your workflows and your apps. Tell us how you guys help customers deal with the constraints out on the edge.
[00:18:08] Saimon: This is a great topic, Eric. Some of the things we also notice are different energy powers. There are different energy levels in different apps and different edge sites. You have to account for those different types of architecture. As we stay very close to our customers and understand what their roadmap is, what the landscape is comprised of? We see any number of things from different hypervisors, bare metal systems, and sensors that would have different limitations associated with them. They require an edge that’s tailored for their use. It’s very optimal and it’s able to harness the power that’s provided to them. As a vendor, we have to provide that unified platform through software that could be installed in any number of locations or any architecture.
Providing that type of abstraction layer is critical. Another point is about how we handle content caching. As a concept that Kris mentioned, how do you make data accessible in a way that is stored on a very limited device? One of the things we do at CTERA is using intelligent data caching that studies user behavioral patterns. Based on those behavioral patterns, we’re able to stage the data. It’s close to that end application or user so they enjoy fast response times. That’s one topic that helps address the limited bandwidth and circuits you have from the edge all the way back to the data center, and latency when optimization is top of mind for a lot of these widely distributed organizations.
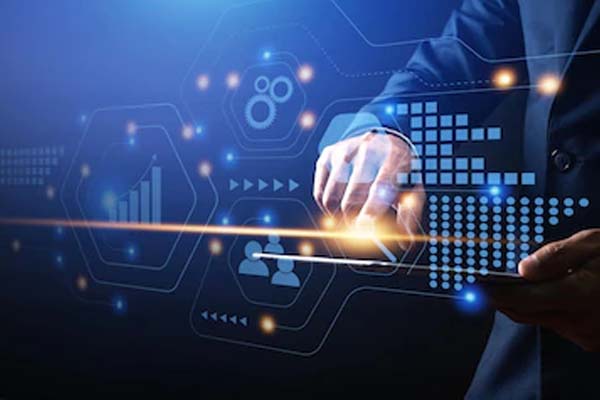
Edge Computing: Providing any type of abstraction layer is critical for vendors who have to provide a unified platform through software that could be installed in any location or architecture.
Another topic that ties into that, as you have this highly distributed architecture, is consistency. The other topic is consistency and speed. We manage so many of these different codes. How do we then propagate security calls, software updates, and any configuration management at a very large scale? We want to be able to do that across any number of ed sites. Providing that central place where you can provide automated change management and control is also highly critical for a lot of these organizations we find.
[00:20:09] Eric: I’m guessing, and maybe I’ll throw this one over to Kris, and then we’ll get Tony back into the conversation. Understanding and being able to quickly ascertain what are the objects out there on the edge? What are the protocols out here? Being able to sense what they are and then understand the interdependencies and how they work together on certain workflows. That’s pretty important stuff to know that we have XYZ devices over here and ABC devices over there. Being able to very quickly understand what is the footprint of this thing. What is this thing that we’re dealing with? That’s a big part of the equation. You have to know that before you start managing and designing your workflows. Right, Kris?
[00:20:45] Kris: It’s a huge piece of the puzzle and one that we think a lot about. Saimon referred to the term fleet management. You’ve got a highly-distributed set of whatever it is out there, and how you going to manage and orchestrate your data, your configuration, your code or whatever it is with respect to that fleet. That is very heterogeneous and complex. One of the starting points of this is the edge is representative of this explosion and complexity.
It’s something we’ve seen in our customers from the start of our business. One of the ways we manage complexity is automation. Humans don’t scale. With the scale, complexity, and distribution of the edge, we need to start to automate. One of the linchpins that we’ve found in automation is step one, to know what the heck is out there in my fleet. Understand what is on these networks and what all the connected devices and systems are.
One of the ways to manage complexity is automation. Humans don't scale with the distribution of the edge. Share on XOne of our big areas of investment is in what we call network source of truth. It’s the system of record for what is out there. One of the first steps in an automation strategy is to get that source of truth, maintain it, and manage it so that you can drive your automation from that. One of the important linchpins to doing that is being able to observe what is out there at the edge. Being able to go and inspect your edge sites, your edge systems, your edge devices, whatever it is, and understand the nature of them, the traffic that is flowing around, and so on.
This is another big area of investment. Back to the theme of hard problems that emerge at the edge, how do you observe what is happening across these incredibly distributed footprints? That has the side effect of generating a lot of data. It’s the same problem we’ve been talking about. There are these circular problems that start to emerge at the edge, and a lot of our investment is figuring out also how you move some of the processing of that data out to the edge, and bring it back to your source of truth or your batch processing systems or whatever it is that you need. Not everything but what you need. There are a whole lot of problems that start to emerge around this one little concept of wrangling, what is out there and starting to orchestrate it.
[00:22:54] Eric: You bring up this great topic of observability, and you’re right. It’s more data, but the observability is fundamentally changing a lot of the game in very positive ways. Maybe I’ll throw this one over to Tony to comment on. Once you can see what’s happening, then you can problem-solve much easier. We’ve gone through all these cycles over the last 30 years of trying to figure out what can we know, then what can we do about what we know, and then you know more. You start to level up, but to Kris’s point, the complexity curve is blown out these days. You must have automation and observability to get somewhere, or you’re going to get blown over by a tsunami of data that will make no sense to you and people will go back to gut instinct. What do you think, Tony?
[00:23:42] Tony: Being heritage storage, we got hundreds and hundreds of petabytes out there, both file block and objects. Everybody now, it’s no secret. The file data is the one that’s exploding like crazy. There is a multitude of tools out there that now can analyze data, construct data, move data, etc. From our perspective, I’m probably coming at it slightly definitely from the other two guys given what we are. We are an edge cloud provider.
What we offer is the ability for us to take those down into smaller chunks while still connecting them across what we call our federated edge, which is about hundreds of our sites that are connected, and then enable them to get better visibility into what their data has. Analytics has come a long way to be able to provide actual real-time information back into what the heck is out there. I don’t think the challenge is ever going to go away because data continues to explode exponentially and it’s going to get less and less.
Analytics has come a long way to provide real-time information back into the open world. This challenge will never go away just because data continues to explode an exponential. Share on X[00:24:36] Eric: I think that’s exactly right. I’ll bring Saimon back in. You mentioned analytics earlier. One of the more interesting use cases I heard in the early days of edge computing was geared around Cisco’s routers, which one of our guests told me were built to have two processors. The thought process back in the day was, “In case one of the processors dies, the other one kicks in and gets the job done.” Some clever person figured out that for edge use cases, you can use one processor to do what it is normally doing. The routing and the other processor can be focused on data crunching. You figure facial recognition at casinos for example. You’re pulling in information and then one of those little processors is just running algorithms all day looking for the bad guys. That’s a pretty clever use case. Saimon, what do you think about that?
[00:25:23] Saimon: I do, and we all have those on our phones now. We all have dedicated chips for machine learning that optimize the images we take. I don’t know how many know this, but whenever we take an image, our phones know how to automatically filter and maybe make them look a little bit better. We also have auto-correction which studies the language we use and what those common words are, and they proposed it ahead of time.
Eric, what you mentioned is this is the opportunity of leveraging additional processing power to not only deliver the service but also use another processor for additional capabilities, whether that be in real-time analytics or replication. We use that concept also in CTERA quite often. We have multiple processors. One may be responsible for immediate file serving and the other is responsible for the replication of data. The third is responsible for machine learning and determining those patterns of how users access the data so we can stage it at the right location based on that intelligence.
I love that approach. This is a unique, interesting option for providing value close to where the entity resides, where the user and application are. It does not mean that we defer all of our analytics to the edge. At the same time, we realize that cloud computing is terrific for big data analytics. As Kris mentioned, we want to create that single source of truth or our authoritative copy. That’s the master data set that we rely on and we can always recover from, but we can also use that full of storage to run big data analytics so we can further improve as an organization, identify new opportunities that provide better care for our patients or whatever field that organization is practicing in.
[00:27:00] Tony: We actually have a real-time example of that going on right now with Seagate. Seagate launched live cloud and we are the compute engine for it. We’ve literally spun up there. We’re spinning up our second cloud for them running one of their largest analytics applications. We had to design a whole brand-new system for them to do it. It’s a huge application. They’re going to be taking it to market as well. To your point, we have a level of server that’s doing one thing, processing one level, then filtering it down to the next level and the next level, etc. We’ve got it deployed on the East Coast and the West Coast. We’re about to go to Singapore with it, and then we’re going to go to Europe with it and continue expanding. They’re doing exactly that analytics crunching at the edge. That’s huge for ours.
[00:27:39] Kris: To chime in very briefly on this one, analytics crunching at the edge has been a big theme for us too. We are swimming all the time in network data. What are all these packets flowing across the network? One of the problems we have operationally and also our customers all have is understanding the nature of that stuff, and then you start to run into trade-offs. The more widely distributed, the edge footprint becomes, the more you have to ask yourself, do I want to crunch all that stuff at the edge? Do I want to slurp it up into some batch-processing system? What are my real-time requirements? What is my data transfer cost is going to look like?
A different concept that we’ve found emerging in our own architecture and that of our customers is this idea of what we call small data. We all talk about big data all the time. We love small data. We distribute the analytics all the way out to the edge. We crunch all this stuff. We bring back only the tidbits that matter for us to look at. It enables us to distribute the processing cost. We simplify or reduce the data transfer costs that drive real-time value. Depending on the kinds of applications, this notion of analytics at the edge is powerful and can maybe change the way we think about big data or even give us new concepts about this small data concept.
[00:28:54] Eric: I was talking to another vendor in this space. I remembered the whole concept of asynchronous java on XML or AJAX when they came out with that. It’s a similar concept to what we’re talking about here because what you want to do is understand at the edge what are we trying to understand. You don’t want to be throwing tons of data back over the network to get back to the data center or even to the cloud. I’ll throw it to Saimon for quick commentary. That’s why architecture is such a big issue here. It is figuring out what we are trying to do. When do we want to send the signal back over the wire? Is it only at important times? What do we want to accomplish at the edge? What do we want to accomplish in the data center? There are lots of different options here and it takes some real thought to figure out how to juggle all that. Right, Saimon?
[00:29:40] Saimon: Right. One of the infrastructure considerations to this also is the fact that as we’re highly distributed, our connections back to that central facility, whether it be a core data center or the cloud, could be limited. It could be very high distances or very low bandwidth. We got to mitigate that. This is part of the reason that it forces us to redefine what are the roles and responsibilities of the cloud and what are the roles and responsibilities of the edge.
It’s looking at all those aspects of data analytics, reliability, monitoring, logging, and figuring out placing those responsibilities where they should be. Part of that is as we collect a certain payload at an edge, we have to extract data set that matters for maybe long-term retention, for compliance regulation reasons, or for certain values that we want to run some downstream analytics in the cloud.
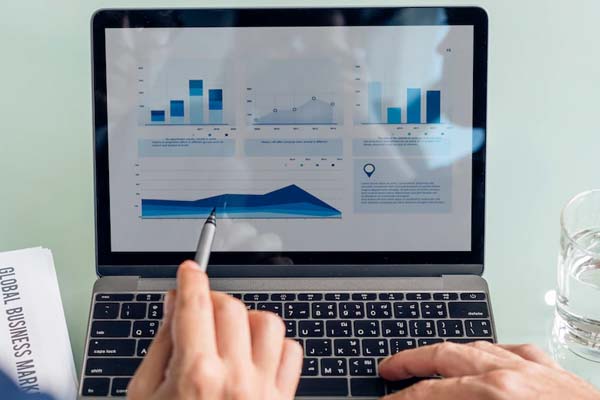
Edge Computing: As we collect a certain payload at an edge, we have to extract data set that matters for long-term retention for compliance regulations reasons.
It’s a very fine balance. It’s something that requires that continuous cycle of improvement. As we gather new data, we learn a lot more, and then we can keep redefining what workloads we want to have running at the edge as well as at the core. As infrastructure becomes better, processing power and networking, we might make some modifications down the road.
[00:30:56] Eric: That’s a good point. Folks, we’re talking all about edge computing and how you can come up with new architecture to solve your problems cost-effectively. This is the key. You can only solve anything by throwing lots and lots of money and hardware at it but that’s not going to solve your CFOs of budgetary problem.
[00:32:30] Eric: Edge computing affects everything, architecture, assumptions, application performance, and of course this whole issue of security. It is a never-ending cat-and-mouse game that will never ever go away because there are so many ways that the bad guys can penetrate your environment. We’re talking with Kris Beevers of NS1. We got Tony Craythorne of Zadara and Saimon Michelson of CTERA. Tony, I’ll throw it out to you. Next step, we’ll go to Kris with this blast radius concept that I want to talk about. Tony, tell me a bit about security and how your approach helps with security and simplifies all that.
[00:33:06] Tony: Most of our customers are currently with hyperscalers like AWS, Azure and GCP. They continue to run many of their large applications there. I’ve seen risk and done risk analysis on the fact that they’re part of a massive data center that is a big fat target to anybody out there. If AWS has only got a few locations across North America, for example, I’ve got hundreds. That becomes a very easy target. Just look at the various credit cards they’ve got and things like that. Over and above that, people are getting slightly miffed about outages, etc. Most of our customers come to us from the likes of AWS because we’re fully EC2-compatible.
One of the things that they are thinking about is security. When they’re working within a large data center with one of the hyperscalers, that’s one-to-one. It’s targeting one big data center with multi parts. Whereas a distributed edge cloud, as we provide, gives far more security, resilience, and a smaller target. It is harder to find those than it is one huge data center. Cost is a major issue as well. We generally save customers about 40% against the hyperscaler. Bringing it to CTERA edge cloud does provide a lower cost but more reliable and secure experience purely because of where the data is located.
[00:34:26] Eric: I remember learning a few years ago about this shared responsibility model with security and the big vendors, which basically means you are responsible. It’s some of that news speak stuff going on. Kris Beevers, tell me a bit about the blast radius concept, which is interesting.
[00:34:43] Kris: It’s very aligned with what Tony has told us about what his customers are after. If you put yourself in the customer problem lens and you are building an application that is meant to be used by people all over the world or you’re processing data in a distributed way that is critical and important somehow, you have concentration risk if you are bringing most of that data, web traffic or whatever it is that you’re servicing to a single place.
If you are building an application to be used by people all over the world or processing data in a distributed way, it is critical to have concentration risk. Share on XWe’ve all known that for a long time. We’ve all built these application architectures that are somehow redundant or highly available. One of the promises of a very distributed edge is that you’ve got a lot of these little nodes or sites all over the internet and all over the world, in some cases, that can handle the workload with respect to whatever your application is. If you lose one of them or you’ve lost a few percent of your capacity software deployment or code operational capacity, I’m going to reroute that traffic to the next site, which is probably pretty nearby, and contrast that with the more traditional architecture of a couple of big highly available data centers.
One of the ways we think about this at NS1, as operators of a big edge infrastructure to serve our own applications, is the nuclear survivability test. This is where we use the term blast radius. What is the blast radius of a data center outage for us? It needs to be pretty small. A small percentage of our end users are impacted if our data center in Chicago and New Jersey is nuked. You also have this notion of blast resiliency. How many of my data centers can be nuked before I have a real problem? One of the drivers of this push to the edge and push to more distributed architectures is the idea that you’re decreasing the blast radius and you’re increasing your blast resiliency.
[00:36:38] Eric: That’s pretty cool stuff. I’ll throw it over to Saimon to comment on. If you think about the hackers that are taking people down, taking data centers down, losing any time at all, especially for some of these big companies, it is very disruptive financially. You better have some plan. This makes a lot of sense to be as federated as you can while still realistically pulling off what you need to do to minimize that blast radius and minimize the impact of some bad actor. Right, Saimon?
[00:37:07] Saimon: As Kris calls it, it’s the Wild West. We also see it very much the same. There are almost multiple layers to this onion. We have our headquarters or our core sites. We can implement state-of-the-art security or we have more control over what we do. As we get to the far edge and the user’s home, we lose control over those types of environments. We still want to provide some access, but those degrees of freedom come at to cost. We have to do whatever we can to at least have those protections in place.
The way we look at it at CTERA, there are a number of elements that are incorporated to improve your security posture, starting by implementing a zero-trust architecture. I know this is a topic that’s receiving a lot of publicity right now. Traditionally, we looked at IT as if you authenticate to a system, you get in through the fort, and then you can walk around everywhere. As opposed to a modern security architecture that implements zero trust. Any relationship between any number of nodes requires authorization and authentication. Access is not assumed. You have to have the proper credentials and context to be able to access a certain resource or take certain actions like checking out a card online. That’s one thing.
The second is delivering on the fundamentals. What I consider to be the fundamentals are application security, network security, and hypervisor security. Those are things that are within our control. As application developers, those are elements we can harden our systems to provide the best possible security and forensics for our customers. They have this big pool of logs where they can also have all the information of what occurred in the system.
The unique part, which we’re seeing more of now, is what else can we do that’s far beyond those two elements. That touches on machine learning, AI, and looking a lot at anomaly detection. It’s understanding what is clearly to be normal behavior and what is an anomaly. Based on that anomaly, taking action, denying access to a certain resource or system, and deleting a user that is malicious from your database if needed. What we typically find is as we use to step into the far edge, that’s when your attack vector increases and you’re more vulnerable to threats that could be exploited because your surface expands. There are more users and easier points of entry into your environment.
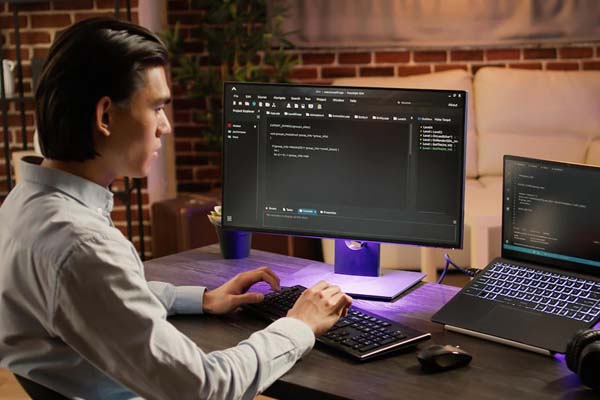
Edge Computing: As people step into the far edge, their attack vector increases. They become more vulnerable to threats that could be exploited because their surface expands.
[00:39:29] Eric: These are all important topics. Also, there’s this whole concept of DevSecOps. We have DevOps where developers are working with the operations people. That solved all kinds of problems. It was cool development in the industry, and it saved us from the age-old business-IT divide. In the data center, you had this business IT divide where the business people didn’t know the tech. The tech people sometimes didn’t know the business. You have this battle going back and forth of what’s possible. Sometimes tech is hiding from doing new things because they didn’t want the added trouble. DevOps then comes along and now you got developers working with it.
It balanced everything out because now the developers are in a different character than most business people or even traditional IT people are. It created a nice dynamic where it wasn’t so much of a battlefield anymore. What is DevSecOps? I know some developers who work for some of the very large companies these days and cloud providers, it’s almost bewildering the stuff that they do. They build artifacts and deploy them. You deploy it only in 1% of the instances to see what happens, and then in 5% and 10%, you roll it out companywide.
There are all these cool things that you can do, and relying on getting it as your repo to be able to do branches and things. It’s fascinating what is possible now if you can understand it all, manage all of that, and wrap your head around it. That approach theoretically should limit the blast radius. It should allow you to isolate the problems, quarantine them, and then deal with them. I remember the old days of security. If you get a virus, you’d quarantine it and stop it.
Saimon made a great point there about machine learning and using this. I’m telling you folks, in these bigger environments, if you do not have some form of machine learning, automation, or artificial intelligence, you will absolutely lose. There’s no way you’re going to be able to navigate through the fields. It would be like in the agricultural context trying to go out there and compete with your shovel, hoes, yaks, and moving your stuff around compared to this industrial equipment that was so much more powerful. Folks, the bonus segment is coming up next. Send me an email at [email protected].
Time for the bonus segment here on DM Radio. We’re talking about all things edge computing. We’ve got Saimon Michelson, Tony Craythorne, and Kris Beevers. Tony, I’ll throw it over to you first. On this topic of AI and where to deploy, you made an interesting comment that it works better on the edge than it does in a hyperscaler somewhere. Explain to us what you mean by that and why.
[00:42:07] Tony: It’s simple. We are in the process of building GPU instances at Zadara. Nvidia now is the most powerful company in terms of IT, especially in terms of processors, but it’s straightforward. AIML needs high-speed, low latency, reliability and scalability. If you are a small customer building an application around AI or requiring AI and you are in New York and accessing the data center in San Francisco, there is inherent latency across that.
If you are able to deploy that from our perspective on a cloud that is local to the offices where you are running or utilizing those applications, we know countless examples of where it runs so much faster but is also scalable. If you want to spin up a new application, I’ll go extreme. We’ve got a cloud in Angola in Africa, for example. The benefits in terms of latency, reliability, and being able to scale far outweigh, in my opinion anyway, putting it all in one place and relying on one place.
[00:43:11] Eric: That’s a good point. Saimon, I’ll throw it over to you to comment on them and then Kris. AI is incredibly powerful, machine learning. Where do you deploy it? Where is it in your workflow? It makes a lot of sense to think that through because of performance and timing. What is the latency that we can handle? Is this for the call center? Is this for security at a casino? You have to walk through the use case to understand what those changes are, but seconds matter.
[00:43:41] Saimon: Depending on the type of AI workload, some constraints are related to how fast you need to make a decision. For instance, in a lot of security applications, you would tend to put that close to where you would want that decision to be made. We see a lot of applicability for AI in the ransomware space. We’re all worried about our datasets getting encrypted. As soon as we can identify that there’s this abnormal behavior, we want to turn it off immediately. That will be a great application for deploying AI at an edge location.
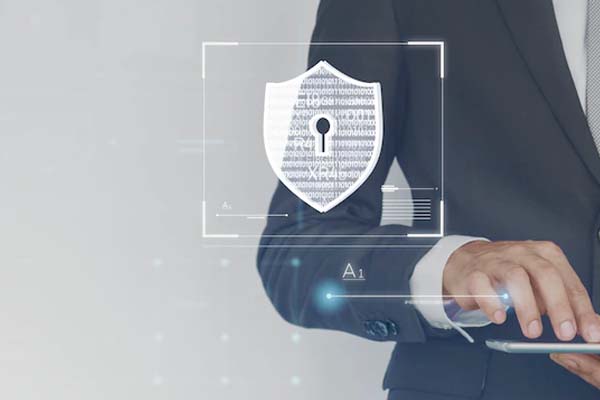
Edge Computing: Many security applications are put close to where important decisions are made. This is why there is a lot of applicability for AI in the ransomware space, with datasets all getting encrypted.
Another instance would be intelligent caching. In our space specifically, we have that need to anticipate where you would require data, whether that’s in a site in Hawaii or another location in Europe. In this scenario, we have a little bit more time until users or applications would require that, but we can start transitioning it. AI could help us again determine where and when you need that data so we make it available for you.
[00:44:38]: That’s good stuff. Kris, I’ll bring you back in. I love machine learning for lots of reasons. One of which is because it can handle such tedious horrifyingly boring tasks and do so at scale. This is what I remind people about because the narrative in the media tends to be all about AI and machine learning taking jobs away and all this nonsense. I’m like, “Give me a break. It’s not going to take any jobs away.” The best thing I have heard was the only negative impact of AI is that people who use it will keep their jobs, and people who don’t, won’t. Tell us a bit about your thoughts on that perspective. Being able to churn massive amounts of data to ascertain meaningful patterns fairly quickly and then inform your decision about what to do. Right, Kris?
[00:45:22] Kris: That’s spot on in general. The other dimension of this that you didn’t quite touch on there that is implied is these machine learning models and AI capabilities, one of the big trends that we’re going to start to see is that these are becoming for us. They’re becoming personalized and specific to particular applications or particular localities. If you bring it back to this topic of the edge and what all that means, what all AI really are, aside from big buzzwords, are models that are large. They’re driven by volumetric data sets that are crunched through these models like neural networks and so on. It results in a machine-learning model that can act for us and make our lives better.
All of us are going to have our own models. All the applications around us are going to have models for them. My house is going to have models for the things that are happening in it. They are going to be personalized. If you think about what we’ve been talking about in this in this whole session around the edge, machine learning and AI are driving a further explosion and distribution of that data because I need a different data set than you do in Pittsburgh, Eric, for example. That’s going to have to happen at the edge.
That data is going to need to be deployed at the edge. We’re going to need to process it at the edge. It’s going to make sense for my model to reside in Ashburn, Virginia. It’s the same as your model. We’re going to want it near us to provide those low latency and great experiences. Machine learning or AI is another application. All of this ties back to this concept of the edge that’s emerged as a result of the increasing complexity and data-driven nature of the world around us. That’s what’s happening.
[00:47:08] Kris: It’s ideally a virtuous circle and in very bad situations, a vicious circle. Folks, look these guys up online, Saimon Michelson, Tony Craythorne, and Kris Beevers. What a fantastic show. Edge computing is the real deal. Get into it and figure it out. We’ll talk to you next time.
Important Links
artificial intelligence automated process blast radius concept cloud computing network data technological innovation