Open The Pod Bay Doors, Please? With Luuk van Dijk And Avinash Misra
What is an intelligent system, and why might we want one? Good questions! Siri is an example of an intelligent system in that “she” can answer questions somewhat successfully. But what about in the enterprise? We’ll tackle this topic on today’s DM Radio, as Host Eric Kavanagh interviews Luuk van Dijk, Daedalean and Avinash Misra, Skan.AI.
—
Transcript
[00:00:43] Eric: Opened the pod bay doors, please. It was HAL in the movie. I’m sorry, I can’t do that. That was way back with Space Odyssey 2001 when they were imagining a future of intelligent systems. Systems that think. Are we there yet? Not really. We do have some great artificial intelligence technologies that are being applied. Probably the best way to describe it is what is called Narrow AI. It is when you are using some artificial intelligence algorithm to solve a particular challenge.
Typically, AI, from my research, boils down to a couple of different use cases. One is classification. That is a common one. We want to understand what is this versus that. Segmentation is another way to look at it. I got 10,000 customers. How can I break them into meaningful segments? These customers like bread products. These customers like orange products, for example. That is a segmentation to optimize marketing, operations and things like network traffic. Is that a glitch? Is that a bad guy? Is it a good guy? We are trying to classify what different things are. It is a common one.
We will talk about computer vision, which is a very hot and fast-evolving space for artificial intelligence. It is a wonderful thing. You have probably seen it folks on your phone when you point at some object and it will understand what that object is. It is quite remarkable how much progress we have made in the last few years.
We will also talk about the other side of AI, which is optimizing decisions. Should we allow this person onto our website or not? Should we authorize this transaction or not? Fraud is everywhere these days. I had a fraud email in my incredibly realistic inbox. It said, “PayPal has deducted $600 from your account for Walt Disney gift cards.” I’m looking at it and I’m thinking, “This looks like a real PayPal email. It is very accurate.” They had a phone number in there to call. I’m like, “I will call the phone number.” It didn’t go anywhere. No one answered the phone. It rang. I logged into my PayPal account. There is no such activity. It was a scam. There is fraud everywhere. It is quite unnerving.
We will talk about intelligent systems and what that means. Is AI ready for a prime time, depending upon the use case? We got Luuk van Dijk. He is from a company called Daedalean. Yes, that is referencing Daedalus. He built the wings. Icarus didn’t listen to his dad. He flew too close to the sun. The sun melted the wax. The wings fell apart and he fell. There was a great poem about that.
It goes about suffering. They were never wrong, the old masters. It references Icarus in the second stanza. It is a crazy story. We will also be hearing from Avinash Misra from a company called Skan.AI. They are also doing cool stuff. Let’s dive right in. Luuk, I will throw it out to you. Tell us a bit about yourself, your company and what you folks are working on in the area of intelligence systems.
[00:03:48] Luuk: Your anecdotes put it a bit and got us started on the sad notes but we are trying to make flying safer. A couple of opportunities to pick better names but I like this one. We try to make flying safer. We have all heard flying is safe and that is true. If you talk about the big airliners, which are incredibly safe given how inherently dangerous sitting in a metal pipe at 10 kilometers altitude going at the speed of sound with all this fuel around you. If you look at any small area, it becomes a lot more dangerous and there is a lot of headroom for safety.
In many spaces, we think that if you have machines that could do the things that humans are doing, you could make that safer. With that, you can make a couple of things and bring them within range. You can think about having denser operations. Everybody wants to do urban air mobility with those flying cars and it is nice, these visions of having 100 small aircraft flying over the city. If that is going to be done by humans piloting aircraft the way they pilot aircraft now, it is going to be an unmitigated disaster.
We are trying to mitigate that disaster by taking humans out of the depths of the control loop. We do that by applying modern robotics, computer vision and deep learning to the problem of flying. We started several years ago what we have to great maturity. It is a system that can use cameras that operate in the same range as the human eye and can see where you are, which is important. If you want to get from A to B, you have to know where you are and where others are flying.
There are rules of the sky that mandate that you look out the window if conditions are visual because you might be on a radio or talking to air traffic control but other people may be flying around without any such guidance and you have to use what is called See and Avoid as the last resorts to not fly into each other. You have to be able to land in a safe place, either planned or unplanned. People usually do this with eyes because while you think, “Can planes land themselves?” A fully automated ILS instrument landing on the ground is a two-pilot operation where two pilots are watching if the computer is doing it right and if there is no glitch in the system and if HAL will let us land.
There are only a couple of hundred airfields in the United States that have the ground infrastructure to do that. People will land routinely on anything that looks remotely like a runway or helipad. We have built systems that can do that. These are the first elements of a drop-in replacement for pilots. By doing that, we let the machines take over to flying more to the point where we can hopefully move the pilot offboard more into a strategic role that looks into lower timelines.
[00:06:27] Eric: Let’s bring in Avanish Misra from Skan. Why don’t you give us an overview of what you are working on, what the technology does and how you’re enabling intelligence systems?
[00:06:37] Avinash: The notion of work in the enterprise has been looked at from various perspectives. There is the perspective of data, human perspective, transaction, systems and so on. We are trying to bring all of these together to give a holistic picture of how work truly gets done in an enterprise. The moment you apply for a loan or open up a new bank account, there is a cascading set of activities that happen in the front office, middle office and back office to make that happen. These are a combination of humans doing certain things on digital systems, back-end systems interacting and a lot of interactions between humans and systems that are going on.
Historically, we have assumed that if we knew the flow of data, we knew work. That is not true because many inefficiencies lie at the level of human interaction with application systems. Those, right until this point, are only understood if you bring in a business analyst to ask questions. How do you do this? What happens in this case? What happens in that case? When you ask people what is happening in a given business process, the answer is not what is happening.
The answer is what they are supposed to be doing. Humans find it difficult to describe what they do. We are trying to break that paradigm by bringing in the notion of observations. Instead of asking people to observe work at scale, we are using technology and specifically computer vision, to observe the onscreen actions of humans in a given business process and across the entire range of activities that you do. That gives us the most comprehensive granular view of how truly the business process or the operation runs in an enterprise as opposed to what the organization thinks, the way the business process runs. There are some intersections of this with process mining. I’m sure we will talk about that as we go on.
[00:08:24] Eric: It is an interesting space because usually, in companies, there are multiple different systems that you are using. You may hop from one application to another, even in any job you do. You hop from a Word document to an Excel spreadsheet, back over to something else and back over to somewhere else. I remember way back in the 1900 and 1990s. It was that long ago. It might have been McKenzie. I can’t remember who it was but one of the big consulting firms did some work with banks where they used cameras and watched where the tellers would walk around and all the people inside the bank.
They did some calculations on how much time was wasted walking from one place to another. They could come in and say, “If you would move the safe over here and move the controller over here, you are going to save about 20% of the time that you are spending every single day. You multiply that out by 5 days a week and 52 weeks a year. All of a sudden, you are starting to save some real money.” That optimization is not new. We have been doing it for a long time but you have to see what is happening to be able to adjust.
[00:09:32] Avinash: Telemetry has always been and it has evolved to do different kinds of telemetry. On a factory shop floor, you observe every single stage of the machining process. You could look at the input and output but if you had a view at every stage from those sensors, you would get a much richer picture of where what is happening. That allows you to intervene in meaningful ways. What applies there applies to human digital interactions to applies to the example that you gave. The science is not new. It is a technology that is new and the overall canvas on which we operate that has changed.
[00:10:05] Eric: Let’s go around the room here and dive into some of these subjects. Luuk, you were talking about using deep learning and computer vision. You want to be able to triangulate the truth. You mentioned how landing a plane is a two-person job. They are both watching to make sure that the computer does what it is supposed to do. You do want to have these checks and balances in these kinds of systems, especially when it is as serious as flying a plane. If you make a mistake, you are not going to make another mistake because you are dead.
[00:10:33] Luuk: That example was specifically about a big airline doing an instrument landing in a two-pilot operation. What it shows is that instruments that you have in a plane are deliberately not integrated. You have a human sitting there who uses his or her eyes to fuse all these instruments, decides which ones to trust and which ones not to trust, looks out the window and puts it together to get a complete picture of the environment.
We worked through a couple of scenarios, flights and what it is that people do. You got to realize that what the pilot is there for is not to push the stick back and forth and push some buttons but it is to manage risk. The pilot is deep in a control loop. She tries to maintain the system state within the safe boundaries of the state space. For that, what you need is situational awareness.
Flying has evolved over 115 odd years from having a human deep in the control loop. In most small airplanes, there is a mechanical connection between your muscles and the applied control surfaces. The instruments are there to provide extra information and it is a cacophony of information. It is up to the pilot to make sense of that. It is a serious problem that there is information overload. Many instruments are clamoring for attention that, at some point, become detrimental.
What we want to do is take that actual role that the pilot has in putting together this whole situation, integrating all these instruments, deciding, “It looks like I can’t trust my GPS because I’m looking out the window. I still see the coastline that I thought I was following. Somebody must be jamming my GPS.” To decide if you can trust one instrument and not the other, you need to have an integrated picture and some form of AI to make sense of that.
To decide if you can trust one instrument and not the other, you need to have an integrated picture, and then you need some form of AI. Share on XWe firmly believe in narrowing it as much as possible because in aerospace, as you pointed out, mistakes are rather high and the cost of a mistake is in an average airplane incident. The median case is that everybody on board dies. That is serious stuff. In self-driving, it is a complicated problem. If you don’t know what you are doing anymore, you can try to gently apply the brakes, come to a standstill and say, “I don’t know what I’m doing anymore.” In flying, getting to a safe state is called landing and it is the hardest part of flying. If you could still do that, if you had a backup computer that could land, you might as well see the mission. That is a bit of a simplified view and I’m deliberately simplifying here.
What we wanna do is we want to create a machine that can make sense of all the central data and say, “I think I’m here. Everybody else is there. That is the ground. There are some moving things on the ground and static things on the ground I shouldn’t fly into.” The problem is much doable with a small team compared to driving. You need hundreds of people for a decade and millions of funding.
Flying, from a robotics perspective, is a lot simpler but the stakes are higher from the safety case. The way that is traditionally addressed in aerospace is the process called certification. You have to prove to the regulator, the FAA or EASA in Europe and a couple of other ones around the world, that your thing is safe. This is where we come in. Traditionally, people have thought of AI and black box. Nobody knows how that works. It is fundamentally uncertifiable.
Back in 2016, there was very much the state of thinking in this industry and my background. I had a couple of nuggets that made me put it together and think, “If I could make the safety case for a machine learning-based system in avionics and aerospace, there has to be value in that.” I admit I came somewhat naive with a build-and-this-will-come attitude. Several years later, we have some systems that do things that are only done by humans.
People say, “I already know where I am because I have GPS.” In Austin, GPS was jammed and a runway was closed. Maybe it was in Dallas, it was in one of the big Texas airfields and nobody knows who jammed. Normally, it is the military who jams GPS and they send out a notice. They were like, “GPS is jammed.”
GPS is a miracle. It is one of the most impressive bits of technology ever created by mankind and it is truly a marvel but it is not sufficiently safe to land an airplane full of people, even if it is a few people. The same with TechnoVoids. You have an instrument called the ADS-B, which is a little radio that is laying here and flying in that direction. If you have a receiver, you can see there are planes but you can’t see planes that don’t have that. You can’t see clouds of birds or flocks of birds. You can’t see big weather balloons. There are all kinds of things that, if you don’t see them, it is not a valid reason to fly into them and you are supposed to keep an eye on them.
[00:15:12] Eric: Hold your thought. We are talking with a couple of experts here in artificial intelligence. If you want that plane to be flown correctly, you want it to land nice and safe, get off and have your trip be successful.
—
[00:16:45] Eric: Yours truly is on the road. I’m in Boston, packed the kayak and have a yard. I’m checking out the Ataccama Conference and they’re doing some interesting stuff with self-driving data. They are an interesting company out of the Czech Republic but they are doing their data people summit here in Boston. Hats off to those folks. We are talking about artificial intelligence and systems that learn.
Avinash, you are using computer vision and that is an interesting way. Are you able to model specific applications and capture what would be described as the defacto business logic by looking at those systems? What I mean is most applications, even in the cloud, you have your menu items and you can scroll around and do things. When you leverage your technology or deploy, are these systems able to figure out, “That’s SAP version 1.7 or Oracle version XYZ?” Are you slowly building out the matrix of functionality that is in these different applications?
[00:17:53] Avinash: Everything is correct except that it is not slow. It is fast. It gets built almost instantaneously as these things come and get categorized into various buckets of functionality that people are using. The bigger problem is not recognizing on a given screen what an object is and whether the save button was clicked and something else was done.
The bigger problem where the challenge lies and where the value also lies is that given a certain set of activities that were done on one screen, what is a corresponding set of activities that were done on another screen by another person for the same person’s process? Where is the deviation? What is the most optimal path? How many exceptions, nuances and permutations are happening at scale? It is a problem of one recognizing individual traces but also being able to put these traces together to see the totality of work. Within that totality, find optimal paths and answer questions that historically have never been answered. For example, where does this process have a choke point? You might find that the choke point is in the handovers.
We are following traces of work on the screen so we can say if this work was active on the screen for these many minutes and an hour, it was un inactive until it popped out in some other application on some of the desktops twenty minutes later. This gives you an unheard-of precision in terms of understanding the floor of work, who is acting on that work, where applications are being used and all of this without ever touching any form of back-end by observing what is happening on the screens.
[00:19:22] Eric: A long time ago, I did some computer programming when I was fourteen years old on a Commodore 64. I built a version of Asteroids, the game Asteroids, where you shoot at the asteroids. The ship comes around and shoots at you and all that stuff. I wrote it in BASIC. Way back then, we were talking about 1982 or 83. I recall that by using BASIC, you could track every object in the environment in 1 of 2 ways. You could track it mathematically from the code. Meaning it was here and it is at this trajectory. Now it must be there or you could look at the screen and say, “What does the screen tell you?” You could map it that way.
I wasn’t sophisticated enough to use both of them as a check and balance. I went ahead and used the screen version because that would be more realistic from my perspective. That is exactly what you are talking about. You are using screen rep and saying, “This is where they are moving. It must be in this app. Now they are in that app.” You get people who have 32 different windows open in their browsers. I’m guilty of that too. You are able to glean some remarkable insights about where the gaps are, where things slow down and where they go down wormholes or choke points. That is pretty cool. Do your clients need to install different software? What is the remedy from your perspective?
[00:20:43] Avinash: Our customers are banks, insurance companies and large organizations that are running processes at scale. The ultimate question that they are solving is that they have a set of KPIs. A contact center and a business process that you are running have a set of KPIs. You want to influence those KPIs, cost productivity and customer satisfaction in a certain direction. The challenge in the bugbear has always been that what you know at an aggregate level, you have never been able to disaggregate in the individual actions that people take in that business process.
We give you, for the first time, a disaggregation of these large high-level KPIs into individual actions back and forth. You can zero in on a given business process, saying, “My customer satisfaction is low because of this particular step in this particular cohort happening here.” It is observability and visibility into actions which is never possible. If you go into the back-end systems, you will be hampered by the amount of integration that you need to do but also, back-end systems, by definition, are only committed states of work. They are not work itself. Work is what happens before and after that commitment state. That is on the screen.
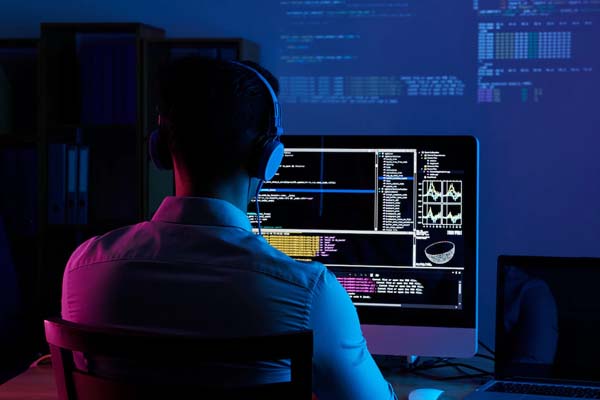
Ai For Business: Backend systems, by definition, are only committed states of work. They’re not work itself. Work is what happens before and after that committed state.
[00:21:51] Eric: You are making a good point and back-end systems are not designed with a good UI. The whole point is it is a back-end system. It is the database or the system of record. You get views that the information that is in there is what happened or what is going to happen, not what is happening now.
[00:22:10] Avinash: If a person is making an entry journal entry or anything of that kind, with the set of systems that they are referencing, they are doing some design work. Referencing and looking at a pricing table or system of record somewhere else is never recorded but there is time spent on it. It has quality implications for the work that you are doing.
If you do not know that your business process involves all these systems which have no implication on the back end, you are working blind. This is not to in any way say that the core technology of process mining has to be done away with. Some things are happening in the back end that is useful. What we are saying is that there is a much more elegant way and much faster way of figuring out deeper insights that relate to human digital interactions and the inefficiencies hidden there. For that, you don’t have to go around back-end integration and log files. Put a small piece of software not unlike this application software using, like Zoom, to capture the screen and use that to understand and add scale to your business process while it is happening.
[00:23:10] Eric: I will bring Luuk back in because you both are talking about computer vision as part of the package. Luuk, you got some deep learning in the background. Can you walk us through how these algorithms work? I’m guessing the data points here are all sorts of sensors. If you look in the cockpit of a plane, you see all of these different monitors and sensors giving you bits of information, wind speed, friction, temperature and all that stuff. Walk us through how the deep learning modules work and what they do.
[00:23:42] Luuk: These existing simple systems are remarkably simple. In a small airplane, you have the mandatory set of instruments, a six-pack of which in a fixed-wing airplane is important. That is the indicator of airspeed, which tells you if you are about to fall out of the sky because you are flying too slowly. As a human, you are supposed to keep an eye on that yourself.
Everything else is optional except having eyes and a brain. In 1939, you can legally and safely fly a small aircraft using no instruments whatsoever, just using your eyes. Conversely, none of the existing instruments are set enough to do that for you safely and legally. You can fly automated but you are blind. You cannot emergency land and avoid other people flying.
From that, it follows that the vision is both sufficient and necessary. What we built are computer vision systems to do exactly that. If you have GPS, you are going to use GPS but you want something that can decide, “I don’t trust my GPS.” It has to be a more reliable system than your GPS. You want to be able to say, “It doesn’t show up on the radar but it is there.”
The computer vision algorithms themselves are pretty simple to decide where you are. We have set up algorithms called SLAM. It is technically not deep learning. It is some finished location of mapping. It uses how the apparent motion from one frame to the next to reconstruct your motion in space. I can talk about it separately but you are interested in the deep learning ones. The two most important ones are, does this little blob of 10×10 pixels in my 12-megapixel image look like an airplane that might be flying toward me?
Object recognition is fairly narrow and well-defined. Look at things that look like they might be aircraft. We have to detect them with high enough precision and recall. In the first recall, you want to see everything that is there. It could be an aircraft and you want to discount the ones that are not a threat. They are funny shapes of clouds or things that are trees and not aircraft.
For that, it is very classical, offline supervised learning, collecting data which is expensive and having it labeled by human annotators who go and look at all the 12 megapixels and draw little boxes around it. The other system for finding the runway is pretty similar. You have to look at lots of pictures that have runways in them, draw a box around the runway and teach a computer to do that. It is sufficiently reliable.
The actual thing of making it work is not the hard part. You get a couple of students fresh out of university, put them in a room, give them chocolates and coffee and they can make that work. The hard part is that in aerospace, you have to guarantee performance. It does not only mean guaranteed performance. In our case, that also meant developing the theory of how to guarantee performance for a machine learning component to the satisfaction of the FAA. That is where we brought value.
In the last couple of years, we worked with EASA, the European version of the FAA and the FAA to sit together because you already think, “It is a mold-determined system. You can never say what it is going to do. We all know that machine learning has affiliates.” How do you design a system that is good enough to land a plane?
There are a couple of tricks to that. The basic system is simple enough. You draw a box around a runway and extract some geometry. To make it safe enough, you first have to make an architecture of your whole system that can deal with the finite fill probability of the neural network itself. Suppose you have a classification task within a neural network like the EMNIST Dataset, the hello world of all classification, neural network introductory classes. The human record is 97% but the current best algorithm could do 98% or 99.8% something.
Suppose you had an aircraft that looked at one picture. I had to take a decision. It got it wrong 1 in 50 times and would kill people. That would be pretty bad. That is not how these systems work. These handwritten character recognitions that is applied in delivering the meal but less than 1 in 50 pieces of meal get delivered wrongly. That is because it doesn’t depend on the single shops looking at a single image.
What you have to do is carefully construct your system so that this 2% gets raised to sufficiently high power and it becomes 10(-7) or 10(-9), which means you have to have a couple of independent shops. What we do concretely is when we approach the runway, we have to see the runway and our confidence. Seeing the runway where it is and our positions should increase. Otherwise, like a human, we conclude we are not sure what we are looking at here and we can’t use this information to land. A human pilot would then abort the landing and go somewhere else where visibility is better.
If you architect the whole system, it turns out that in the cases that we are talking about. I’m not claiming that this is more than Narrow AI that is doable. The second ingredient and this is important, is that suppose we make a system that can deal with a 2% failure rate of the component. We look at multiple images, do the approach and get this sufficiently high power.
The very important thing is that this 2% holds when we go out in the wild and fly in real conditions. That means that the test sets that we evaluated have to be sufficiently big and drawn from the same distribution to be an adequate representation of reality so that the informants we measure generalize from the wild to real life. This concept of generalization as the fundamentals of certifying critical application is the concept we have developed together with the regulator in the last several years.
There are other schools that thought. I’m not going to claim the monopoly on people trying to solve it here. There is a school of thought that says if only we had explainable AI, we could explain everything. Therefore, if it was wrong, we could fix it and we would be done. That is not how it works because the reason you use the machine learning component, to begin with, is because you have a problem that has a large amount of inherent uncertainty.
If only we had explainable AI, then we could explain everything. Share on XIf there was a crisp yes or no, this is the runway. This is not the runway. This is an aircraft. This is not an aircraft. You wouldn’t use a machine learning system because you would provide some codes for this. To take that step from the probabilistic nature of the problem and system that we make to do it and come to a safety guarantee that is sufficient for aerospace, that is where we put in all the work.
[00:29:54] Eric: You are working with the right people. I’m sure they have their skin in the game because it is their responsibility to make sure that everything works perfectly. We are in a very interconnected world. I’m in the broad of the top of the hour. Some people are trying to do bad things all the time and people are doing bad things at airports. Some lasers were being pointed at the pilot’s eyes in some of these airports not too long ago. This is a big meaty problem.
—
[00:32:02] Eric: Avinash, I will throw it over to you first. I would have to think that you could have a whole separate service offering around working with software companies to better design their apps and their user interfaces. Is that something you do?
[00:32:14] Avinash: No, we do not do that but you are right. Our focus is more on helping large organizations optimize the way they work and understand where the nuances and deviations are, historically and even leading up to the state. They are going by second-order data to be able to ascertain how the business process is running.
We are looking at KPIs, which are post-fact. We are looking at overall aggregate cost metrics, which come to be because after things have happened. We are giving them a real-time way to observe how things are happening. As we were talking off-air, you said you couldn’t go back to not knowing. Similarly, once you begin to lighten up these dark processes, put a light on how this business process is running. You can’t go back to running the business process without knowing what are the nuances, exceptions and permutations. How much time is being spent on a given business process? What is the breakup of that time?
Questions such as where are there certain cohorts that are doing certain things differently, leading to better or worse outcomes? These are insights that are possible and the disaggregation of large KPIs into individual actions that humans and software take is the traffic on our stage. We are trying to solve that problem. Yes, a coronary of that may well be that you might want to redesign software, which is a step that our customers often take. We realize that we got change not the process but also the tooling behind the process.
Before Skan comes in, this is dark that people are making certain entries based on certain research. What is that research they do? Do they go to Google or look at a training document that your organization has created? Once you begin to know that, you can put it into the workflow. This is akin to redesigning the application flow but you can bring that into the workflow that every time this entry has to be made, we will pop up this information for the agent to use. This is a simple example of what can be turned into a much more comprehensive exercise. Do I redesign the entire workflow?
[00:34:14] Eric: You are reminding me of the real power of AI and machine learning. In my opinion, it is going to boil down to one word. It is a suggestion. The AI will suggest to you, “Why don’t you do this? I see you are doing that. Why don’t you do this?” If you look at Amazon, it has basic stuff like, “People who bought this also bought that.” That is a different thing but it is still giving you some context from the crowd.
Where I see the value of AI and I will throw it up to you first, Avinash and over to Luuk to comment on, is making these suggestions. I mentioned I’m at this Ataccama Conference. That is what they do too. They will notice when Susie loads data, it is usually marketing data into these databases with these fields. She logs in, opens up a document and it will say, “Do you want to put it into this system like you always do?” You say, “Yes, I sure do.”
There is another example I saw and this went away. It probably changed some settings. One of the challenges in the world is that everything is changing so fast. Staying on top of the settings in your browser on your phone is a bit of a challenge. I noticed that Google and Gmail were doing something interesting, where they were dynamically choosing which high-priority emails probably are for you. I was impressed at what they had done. They would notice that this appears to be a sales thread and you haven’t responded in the last three days. Maybe you should get on that. That is some interesting sophistication from an analytics perspective or an AI in a suggestions perspective. What do you think about this mantra of AI as the power of suggestion?
[00:35:52] Avinash: The area that we have chosen to work on is understanding human digital work in business processes. This has an important implication and that implication has to do with compliance and control over business processes. Not just a suggestion but being able to figure out that if certain steps are taken, it is necessary that certain other steps not be missed or done. You can treat this as a suggestion or as a report that certain steps were done or not done and loop back into popping up the exact things that follow one after another.
Organizations, banks and insurance companies have everything to gain or lose both by way of regulation, as well as by way of the SLAs that they signed with their customers to be able to run the processes in a compliant manner. The power of suggestion is not merely that it is suggestive that you put this next best action but it is the action and any deviation from that action may lead your organization to be either out of compliance from a regulatory perspective or an SLA perspective. It is an important aspect of all the data that you are coming together. You are able to predict and prescribe based on that data.
[00:37:00] Eric: Luuk, I will throw it over to you on the power of suggestion. Is that one of the best ways to describe what AI can do for you?
[00:37:06] Luuk: I hadn’t thought of it and while you were chatting, I was thinking that it is interesting that there is such an analogy between flying a plane and these business processes because, like in self-driving space, EASA has suggested that our levels of autonomy for flying. The first one is where the machine learning component or the AI as we tend to call it, augments or assists the pilot, in a sense, our visual landing guidance. You can control it directly to the outer pilot. It may be safer in the first incarnation if it shows the little cross to the pilot and if he puts the big cross on the small cross, you hit the runway where you want it.
In a way, that is like suggesting, “How about you go a little bit to the right? How about you go a little bit to the left?” The pilot may have other information that makes her decide that wait a bit until following up with this suggestion. As you move to the higher levels of autonomy, where you don’t want to lose it, the final say is with humans.
The time scales might get bigger. We may want to move from tactical things to strategic things but there are also these systems that will not replace and take humans out of the loop for the overall responsibility of the system. They will make it easier to do the right things. Sometimes by automating things away. The difference between automation and autonomy is I see more in where you want to have the final say with some conscious person if something is a good idea or not. That is an interesting observation.
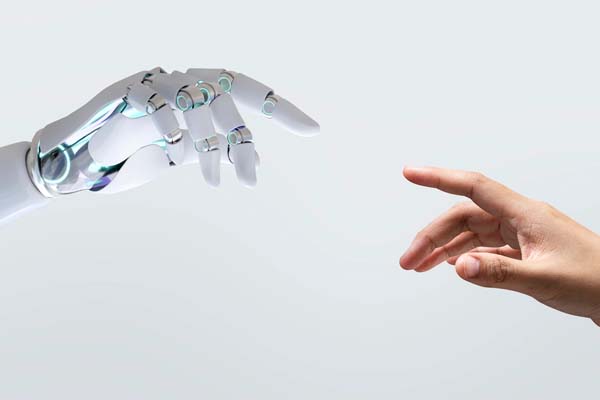
Ai For Business: We may want to move from tactical to strategic things, but these systems will not completely replace and take humans out of the loop for the overall responsibility of the system.
[00:38:29] Eric: I always refer to it as the manual override. Any time you automate something, you want to have that manual override in case it goes so fast.
[00:38:38] Luuk: It depends. The manual override might not be a joystick that supposes you are in control of a rocket with a joystick. You are a primate that has volunteered to fly on Elon’s rockets to the ISS, the moon or Mars. Seriously, what are you going to do with a joystick? You want the computer to steer that rocket because there are not a lot of useful things you can do. It holds up to a point but whether we are going to launch at all or things that are on the time scale of a second, 10 seconds or 100 seconds, you want to give the human in control all the information and the time to think of it. Putting information forward is one of the tasks where the machine may help.
[00:39:17] Eric: I will throw it over to Avinash to comment on very quickly. If you can, do a show bonus segment too. What are your thoughts on jobs? What jobs are you looking for? What people are you looking for to join your company? What would their roles be?
[00:39:30] Avinash: The number one job that is important at Skan is people who can figure out data. We are generating huge amounts of data and algorithms are crunching that data and finding patterns. Once you find a pattern, how do you link those patterns back into business decisions? What can we serve for businesses to figure out?
Case in point, for example, we have a customer who has all the data in terms of what is the most expensive step in the process and where they spend time on until someone got the bright idea that we can use this data to be able to differentiate price our services to our customers. Now we know for whom we are spending how much time.
You called a lawyer. You have many paralegals working on different cases. If you could figure out that a certain step that there is more expensive for a given case, you price that case differently. We are looking for people who can come up with all of this insight and intelligence that has been created for bringing it back into the business.
[00:40:31] Eric: This was a fascinating conversation. Look for these guys online, Luuk van Dijk of Dandelean and Avinash Misra of Skan.AI.
Important Links
About Avinash Misra and Dr. Luuk van Dijk
I am an intellectually curious entrepreneur and CEO with a mission to solve the problems that hide in plain sight. My current company Skan.ai, which I co-founded with Manish Garg, helps organizations understand the truth about their business processes, so they can make more informed decisions.
Looking back, the majority of my career has been spent as an entrepreneur and what has been driving me in that pursuit is the combination of building something from scratch and making a personal impact. My approach has always been expository. I love to unpick a problem, explore where the paradoxes exist and work with my team to provide a new solution.
Luuk is the CEO of Daedalean AG, where he leads a team in the development of autonomous flight software for the electric personal VTOL aircraft of the near future. Daedalean innovates through combining cutting-edge robotics with avionics and safety-critical standards. Luuk advises a number of international tech start-ups and previously held Senior Software Engineering positions at Google Zürich and SpaceX, where he worked on infrastructure, flight software, and machine learning projects, among others. He holds a PhD in theoretical Physics.