A Stitch In Time – How Analytics Optimize Operations With Nick Jewell, Navin Sharma, And Shruti Bhat
Host @eric_kavanagh welcomes Nick Jewell, Ph.D., of Incorta, Navin Sharma, VP of Product at Stardog, and Shruti Bhat of Rockset, to talk about how analytics optimize operations. The razor’s edge of innovation today reaches operations’ front lines. Companies use data-driven analytics to optimize key business processes at scale, improving performance, customer experience, and just about any other metric that can be measured. Of course, the devil’s always in the details somewhere. To learn from the experts, check out this episode of DM Radio!
—
Transcript
[00:00:35] Eric: Welcome back once again to the longest-running show in the world about data. I am here with an all-star cast. I’m excited about the lineup and the topic. The topic is operational analytics and how it helps fine-tune operations, quite frankly. We’re going to talk to several guests. We’ve got my good buddy, Nick Jewell on Incorta, my other friend, Shruti Bhat, from Rockset and a newbie in the show, Navin Sharma, from another interesting company called Stardog. They’re all doing things in the space of operational analytics. What does that mean? Analytics is analyzing data and trying to understand it.
There are many venerable players in the space of analytics like Teradata. We then had a whole big data splurge with Cloudera, HortonWorks and MapR and that shrunk down a little bit. There is a whole renaissance of new analytic database engines that are launching left and right. There must be seven companies in this space at least doing some very interesting things.
The whole point is trying to be able to do analytics at the speed of the business. Instead of an offline process where it’s at the end of the week or the end of the month where someone is analyzing, “What happened? How can we change things,” with operational analytics, you’re trying to understand what’s happening and how you can solve this problem right this second. Think of things like fraud detection or manufacturing. When there’s a problem on the manufacturing line, I can tell you it’s a very unpleasant experience.
I learned years ago in a past life in the print production world, talking to the pressmen who worked on the big machines that printed newsletters, magazines and so forth. One guy explained to me, “If those machines aren’t running, we’re not making money. That’s why we want those machines running all the time.” They button down their processes leading up to the actual print job. They have a press booming. You come down, take a look at it and make sure it’s all working. You give them the thumbs up, then go back and do your job. In the manufacturing world, that’s still the case. How can you get a view into the manufacturing world? Think about all the supply chain challenges that we have going on. It’s big money. It’s very important
With any customer-facing solution that goes through the Cloud, you got all these site reliability engineers who are working nonstop to solve issues. They’re like, “Why are things going slowly? Why aren’t these cards processing? Why aren’t the orders going out the door?” I can tell you that troubleshooting has always been a challenge. It’s especially challenging with what we call the modern data stack. Let’s bring in our guests. I’ve got Nick Jewell downing in from the UK. Tell us a bit about yourself, Incorta and what you are doing in the world of operational analytics.
[00:03:30] Nick: Thank you very much, Eric. I’ve been in the data and analytics space for many years. I started with a PhD in Computer-Aided Drug Design. I’ve been working in the industry both as a customer and as a vendor for analytics software. I’ve seen both sides of this journey. I’m heading up solutions marketing and Incorta’s product team so I get to spend a lot of time with customers and prospects and help shape the platform as it develops into the future.
[00:04:00] Eric: That’s a cool place to be because you’re the interface or the liaison between the product team and the customers. Since you’ve been in the industry for a long time, you understand development cycles, customer needs, prioritization and all that stuff. That sounds like a perfect job for you.
[00:04:17] Nick: It’s brilliant. You do get to hear customers’ dreams for the future. If we do it right, we get to serve some of those up for them, which is great. I’ll tell you a little bit about Incorta. We’re a bit of a disruptor in the analytics space. You could say we’re the only vendor to be recognized in the Gartner Magic Quadrant for BI and analytics and their market guide for analytic query accelerators. It tells you a lot about the role of delivering insights but we’re also about getting you there as quickly as possible.
We were talking in the pre-show about Cole and the different guests you’ve had in the past. The founders of Incorta have got this extensive, phenomenal experience in what’s called the ERP and the CRM space. They’ve been building business-critical applications for decades. For our audience who might not have come across those terms, we’re talking about software that runs some of the most important operational processes inside of your company.
Incorta’s mission is dedicated to solving the business application’s data problem where operational data gets captured in these transactional systems like Oracle’s E-Business Suite, NetSuite or SAP. That data struggles to get into the hands of data analysts, data scientists or even financial controllers or accountants so that they can use that data to make better decisions for the business. To your point, whether that’s optimizing a supply chain, targeting a customer segment or simply getting to close the financial books days faster than before when you hit that month or quarter end. I’ll pause there and let the other guests introduce themselves. Thanks very much for having me on the show.
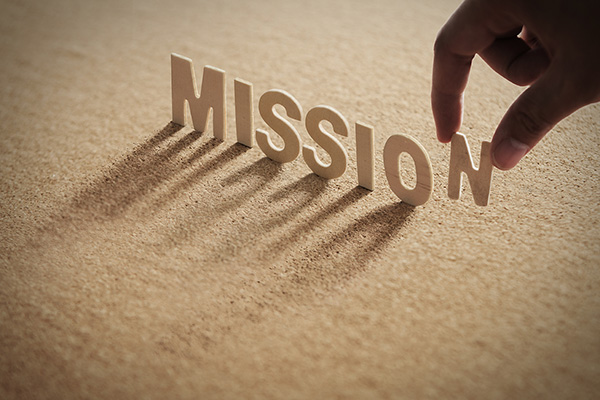
How Analytics Optimize Operations: Quarter’s mission is dedicated to solving the business applications data problem where operational data gets captured in transactional systems like Oracles e-Business Suite, NetSuite, or SAP.
[00:05:57] Eric: We’ll get right back to Nick in a second. Next up is Shruti Bhat from Rockset, one of these companies I’ve been talking about that’s taken a different way. You have an interesting approach where, if I recall correctly, you create three indices of data as it’s streaming in. The data is coming in and you’re automatically creating these indices to be able to query the data and find the data. Discovery is such a huge part of the process.
If you’re a business analyst in the real world and you’re trying to solve some problems, what do you do when you’re asking questions about people and systems? You’re asking questions about the data. You’re like, “What’s this? What’s that?” You piece it together in your mind. To have that immediacy of analytical capability strikes me as important and compelling. Tell us a bit about yourself and what Rockset is doing.
[00:06:41] Shruti: First of all, thank you for having me on the show. We take a very different approach. I’ve been at Rockset from the beginning. I’m part of the founding team here. A lot of our founding team came from Facebook. Those are different backgrounds saying, “If Facebook can build a personalized newsfeed for you in real-time based on who your friends are, what they’re commenting on or what they’re liking, why can’t your customer support operations team know in real-time exactly what your customers are doing? Why can’t your sales operations team know exactly which customer is having issues and needs proactive reach out versus which customer they should be reaching out to talk about a bigger discount or contract?” This is hard for the enterprise to do and this is where Rockset comes in.
Our approach is converged indexing. We build a converged index. The differentiator that we focus on is how you do this on streaming data. Change data capture is all the rage. If you have CDC streams coming from your Oracle, Postgres or MySQL, that’s great. How do you take that in real-time and join it across whatever other data sources you might have? The interesting part is the shift that we’re seeing. People are building applications on this.
Facebook is a great example of a real-time analytics application. Once it becomes real-time, you don’t have humans staring at dashboards anymore. You have an application that comes, taps you on your shoulder and says, “Something’s off here. Go take a look at this particular thing.” Our whole focus is on enabling these real-time data applications. A simple way to explain Rockset is to think of it as sub-second analytics. Those are sub-second search aggregations that join on real-time data. That’s CDC streams and event streams coming from your different sources.
[00:08:40] Eric: That also is another whole renaissance that I’m seeing, which is the next generation of apps. Snowflake talked about it at their event. Lots of other vendors are talking about this. The idea is that we need apps that are driven by analytics or AI, for example, which is a different kind of application. Traditionally, applications go to a static database to get the pieces and parts that they need to come back and show you some view of the world. Since you’re talking about this next generation, it’s a different approach. You need a different engine. The old engines aren’t going to do very well with that use case.
[00:09:14] Shruti: The two things that the old engines struggle with is one, taking that data in real-time. Anybody who’s trying to do this in a warehouse will tell you that the thing that breaks your warehouse is when you do too frequent updates. You don’t want to do it. It’s built for batch. Do your nightly batch uploads and it will do its best work. For this kind of use case, you can’t have your customer support team looking at yesterday’s data. You can’t have your ground crew operations for JetBlue looking at yesterday’s data. They need the most recent data. That’s one thing all systems don’t do.
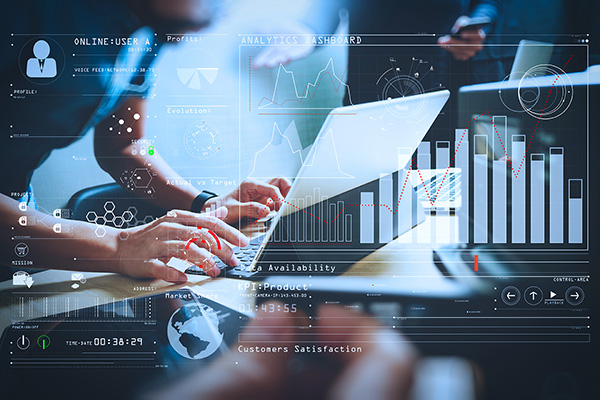
How Analytics Optimize Operations: The two things the old engines struggle with is taking that data in real-time. The second thing that all systems break very typically is query latency.
The second thing that all systems break very typically is query latency. If it’s an analyst looking at a dashboard doing quarter-end analytics, it can take a minute. That’s no problem. If it’s an application and you have a customer on hold trying to rebook their flight, that query better comes back fast. Otherwise, you’re going to have angry customers. The second thing that we differentiate from the older generation is these queries need to be fast. We like to say it is fast analytics on fresh data. That’s very different from slow queries and stale data.
[00:10:27] Eric: In those use cases, it makes complete sense to go down that road. That’s the key. It is to understand where you have this immediacy of the need for analytics-driven information. That’s your focus.
[00:10:39] Shruti: This is operational analytics. You mentioned fraud detection. We see a lot of anomaly detection. Talk about an application coming, tapping you on your shoulder and saying, “Something looks weird.” Anomaly detection is a classic use case for most operations teams. Think of a risk operations team. We have this major FinTech customer based out of Europe where they run risk operations. The problem with doing it in a warehouse was they would know six hours later that Apple Pay stopped working in West Africa. Think about how much revenue they’ve lost in that particular time window.
As their scale got bigger, those 6 hours became 24 hours. It became a bigger window of time because processing everything and start finding the anomalies were taking too long. When they switched to Rockset, it is within a second. If something goes wrong, everybody gets paged. Until they get paged, they don’t have to worry about it.
[00:11:38] Eric: That sets people’s minds at rest. You can focus on your job and not worry about things. It doesn’t hang over your head. Everyone knows what that’s like. That’s no fun. We’ll come back to Shruti in a minute too. Let’s bring in Navin Sharma for his opening statement about being from Stardog. Tell us a bit about yourself. You got a knowledge graph going.
[00:11:56] Navin: Thanks for having me on the show. I’m the VP of Product. I’m also responsible for our strategic alliances with key technology partners in Stardog. In terms of background, I come from a similar stage as where Nick and others have been. I spend many years in the industry around data management. I grew up in the data quality, data integration and master data management domain. I get the idea of enabling organizations to gain insights from the data.
We’ve always found this challenging as either practitioners or creatures of habit. We tend to think about the world in terms of how we see things as business concepts. Unfortunately, in the way we model data and information, it is held hostage to the underlying data storage infrastructure. That problem tends to cause a lot of organizations to not get the analysis they need in time.
The way we model data and information today is held hostage by the underlying data storage infrastructure. Share on XMore importantly, they’re also looking at the challenge of working with data that sits across data silos. That’s a tendency we see many organizations continue to face even in this stage where a lot of the data is being brought into a data lake environment or even into a lake house construct. It’s still lots of data perhaps separated by domain, if not storage infrastructure. We all know that not all data will land up in a data lake eventually anyways. We talked about streaming data and certain applications like ERP operational systems. They’ll tend to have the best source of the freshest real-time information so you don’t want to work off of snapshots.
The way I describe Stardog is much like the way Shruti talked about getting fast data. We talk about faster queries across wide data. In this world where we’re constantly working with more data environments, especially in a multi-cloud or hybrid cloud environment, we’re going to find that the problem is starting to compound more. Even our best efforts in modernizing our data stack by bringing everything to a data lake still remove the actual users who need to consume this information in the context of what problems they’re trying to solve.
One of the benefits of what Stardog provides being an enterprise knowledge graph platform is that we’re able to attach meaning to the data itself through the semantic data model that comes at it from a consumer-consuming application perspective rather than the underlying data storage perspective. Do you know how we think about things as concepts? We think about suppliers, customers, products and inventory. That’s often how users across various industries or business functions think about the data. They don’t think about it in terms of rows and columns. That inflexibility where they have data trapped in these rows and columns makes it harder for them to make sense of the data and run it for the analytics purposes that need it.
That’s where Stardog comes in. We allow them to create this semantic data model that’s abstracted away from the underlying data storage. This can be meaningful to the analytics team that’s working in a manufacturing context. You talked about the manufacturing case of looking across the entire supply chain, being able to represent that information in the context of those business concepts and not worrying about how the data is stored, where it’s stored, what location and what structure. That’s the power of the knowledge graph. It’s being able to separate or abstract out the desire for information, tying it to the real-world concepts that are more meaningful to the consumers and coming at it from a consumer perspective rather than a data producer perspective.
The power of a knowledge graph is being able to separate, abstract out the desire for information, tying it to the fundamental world concepts that are more meaningful to the consumers, and coming at it from a consumer rather than a data producer… Share on XWe’re leaving the rest in terms of being able to connect to the systems regardless of where they are in this homogenized environment where we can, in turn, translate a query from a citizen data user to the source itself. Whether that source happens to be a delta table on Databricks or that happens to be a table in Snowflake or Postgres, our magic is taking that query and translating those to optimized queries back at the source itself.
We are a knowledge graph platform so not everything has to be virtualized in terms of access. We do what we can and we do materialize. We do have an underlying storage engine as well. The key aspect of what we’re delivering is the ability for the citizen data users to close that last mile and true data democratization effort and be able to work across these data silos with data that has meaning attached to It.
[00:16:29] Eric: That’s very cool. We’ll get into that in the next segment because you are talking about this abstraction layer, which solves the heterogeneity and the topographically challenging environment that we have with information systems. They’re all over the place and all different. What a fascinating episode already.
Nick Jewell of Incorta, Shruti Bhat from Rockset and Navin Sharma of Stardog are all doing very interesting things to enable rapid-fire analytics. Who doesn’t want to know? I did a show with a company called Swim. Software and motion is a series that we’ve done for them. He built a whole stack around real-time. He’s like, “We need to reinvent all the wheels from the bottom to the top to make this thing happen.” What are they tackling? It is real-time analytics at scale and scale is the hard part.
You’ll hear all the time in the AI world about someone coming up with a great algorithm on their Jupiter device or notebook, for example. When you put that thing in production, you hit what’s called scale, heterogeneity, all sorts of impedance mismatches and things that you didn’t expect. That’s why it’s hard to do. That’s why you see a lot of different companies coming up with different approaches to be able to facilitate that use case to be able to grab data from the fire hose. Think like Kafka from Confluent.
The engine that drives LinkedIn, they open source that. It’s a huge thing. Lots of companies are exploring streaming-first architectures. It’s the same idea. You want to have fast answers to solve problems in the business. I’ll throw it back over to Nick Jewell from Incorta. As I recall correctly, some of the magic of Incorta comes in the ability to unload that data very quickly. You pointed at Target. You suck that data in and could do your analysis quickly. That’s half the challenge right there of getting the data into your environment and allowing you to do the correlation, causation and all that stuff.
[00:19:39] Nick: To align with Navin and Shruti, their definitions were great around fast queries. I’d say Incorta is all about fast queries across complex business data. These are environments that have often been underserved when it comes to analytics in the past. Something one of our founders loves to talk about is the fact that one size doesn’t fit all with data as well. You might have the Facebook or the Netflix of the world dealing with hundreds of petabytes of data but that data is structured in a specific way. It’s often capturing click streams, impressions or social likes. That can be held in simple table formats and can be sharded out across large clusters of computing.
It’s very scalable when you get into those hundreds of petabytes. However, when you’re dealing with complex business data, it can only take 1 billion or 2 rows in this incredibly complex format where you have thousands of database tables or often, hundreds of thousands of relationships between those tables to bring these queries down. If you try querying directly against one of these applications, it’s a non-starter. Even if you could work out where the data lives, you run the risk of a query that brings your system down.
Even if you could work out where the data lives, you run the risk of a query that brings your system down. Share on X[00:20:49] Eric: You go back in time to remember where data warehousing came from. It came from the awareness that these enterprise resource planning systems or the systems that run your business were not designed to be queried. They were designed for a transaction and to be able to pull in information and make sure that Bob got his box and Susan got her box. Those are complex challenges.
Building out ERP systems is very complex. We talked about that. One size does not fit all. That’s why an SAP will have all these different modules that they bring in there because the business models are different. You’re dealing with time series issues like, “When will this get there? When will that get there?” Think of manufacturing. They’re not designed for that so what do you have to do? You have to pull the data out and then do your analysis in some environment. When you discover things, then you go back and change something in the systems.
[00:21:42] Nick: This is the same data warehousing playbook we’ve been running for three decades. It causes enormous problems. The real problem is the plumbing itself or the architecture. It’s what needs to happen behind the scenes. It’s incredibly complex and expensive. The modern data stack has hundreds of different tools potentially to lift, shift and most crucially, transform that data. There’s the time needed to code and test the business logic associated with the data movement. There are months to build reports and models off of the transformed data itself. Honestly, in short, it’s pretty much the worst car you ever owned. It’s always breaking down. It’s never getting you to where you need to be. It’s always costing serious bucks for maintenance.
[00:22:26] Eric: I’m trying to wrap my head around how to explain this to folks. It is like fixing a car. Not a lot of people can fix the car. We have to remember that we have all this what some people call technical debt. It’s extant systems that are running operations. For big companies, especially, you have this long arc of very old systems, rather old systems, pretty old systems, old systems or relatively new systems. You get this whole spectrum of system technologies that run at different speeds.
You have networks to network that stuff altogether but even the networking is changing. You’ve got software-defined networking. There are all these different ways you can solve problems but sooner or later, you have to get down to the source and change either the architecture or the system itself. You’re going to learn something but you can’t continue to try to add bigger, fatter servers and things like that. Sooner or later, you have to change something fundamental about the business process itself.
[00:23:24] Nick: What attracts customers to Incorta is that it does have this fundamentally different take on the whole end-to-end process. We pose the question that if you could run real-time analytics on raw business data, what if you could do that without pre-shaping or pre-aggregation, eliminating all of these overly complex data pipelines which are full of choke points and delays?
You appeal to a CFO in a company. What if they want to put analytics on top of the company’s general ledger across hundreds of these different tables? They need to drill down into operating cycles or cashflow or take them to the FP&A team, the Financial Planning and Analytics team to understand why expenses are over budget for a particular division.
Shruti, you touched on this a little, the diagnostic analysis. It could be the fact that these data-driven applications run themselves and they flag up anomalies. Maybe in HR, you have people leaders understanding whether or not they’re hiring people enough to get over current attrition rates. It’s these areas of applied analytics on top of these operational data sources and operational processes that customers see as a major differentiating factor when they come to choose Incorta as an analytics vendor.
[00:24:34] Eric: That’s a good set of points you made right there. It’s a good segue to bring Shruti into it too. What Nick is trying to say here is that there are these areas in the organization where to do your job properly, you need information from multiple sources and it’s relatively unwieldy information in a lot of cases. In all these examples, whether it’s the knowledge graph, Incorta’s approach or you with the indices, there are different ways that you’re trying to open up a window into the world of what’s happening. You let people do either troubleshooting or scenario modeling to be able to come up with ideas and test those ideas before you push them into production. What do you think, Shruti?
[00:25:15] Shruti: The fact that data is in so many different places and formats make life hard for everyone. Nick touched on this when he said operational data. Operational databases have been around. Everybody thinks of the system of record as your operational database. That is your Oracle, Postgres, MySQL or whatever’s behind your ERP CRM system. Those are not built for analytics. Yet, if you want any real-time queries, your only option is to try and hit your production database or create a read replica.
If you want any real-time queries, your only option is to try and hit your production database or create a read replica. Share on XEverybody knows how expensive it is to create Oracle read replicas. You have people creating a lot of different read replicas because you don’t want to put that analytical query onto your primary production database. This is where the new pattern is emerging. Why can’t you do that in a warehouse? We talked about this but then, what’s the other option? You don’t want to hit your production database. You don’t want to go to your warehouse because it’s too batchy. That’s where this new world of operational analytics and real-time analytics databases come into play.
What we’re finding is this pattern is around real-time change data capture. DynamoDB, MongoDB and other modern databases got this right. They have this thing called Streams API. They’ll give you CDC streams. Anybody can consume a change data capture stream. Every insert, update and delete is streamed to another database. Rockset consumes those streams natively but then what happens when you have Postgres, Oracle or some other database? You still need some way to capture the CDC streams. Once that comes into Rockset, what we are doing is giving you search aggregations and joins on top of that.
The interesting part is these are showing up as JSON streams. We were talking about JSON over the break. The CDC stream is coming from Debezium Kafka as a JSON stream. You have an event stream that’s also coming as a JSON stream or an Avro stream through Kafka. Certainly, you have all the structured data in your traditional operational databases in the form of structured SQL tables but they’re emitting change data capture streams in the form of JSON streams.
On the destination side, you have to be able to take that in and somehow give your end users SQL search aggregations and joins on top of these streams. This is how we are approaching it with the converged index. We take all these JSON streams, even if it’s deeply nested JSON and give you a fully typed and fully indexed SQL table. You can join against S3 and join your Postgres database. Suddenly, you’re making sense of your world but you’re doing that using standard SQL in real-time.
It was Navin who talked about the citizen analyst. That’s the crux of it. How do you give the power of this analytics insight to every person in every operations team? They shouldn’t have to go, “Wait for your batch nightly job to run.” They should be able to ask the question as they think of it. Most of the time, in operations teams, they’re not doing quarter over quarter or year over year. They’re doing a lot of drill-downs like, “What happened to that shipment? I didn’t see it come through when I was expecting. Let me go look up that. What happened to that?” There are a lot of drill-downs and joins that you have to do in real-time. How do you empower people?
[00:28:51] Eric: If you’re thinking about these use cases where something is being shipped, if you don’t have a solution like this, you have to wait for some batch process to come into place before you’re even going to have visibility to that. People know this. You’re talking to someone in customer service. You’re like, “I don’t see that yet. We don’t see that in the system yet.” Why not? It’s because you have an older system that doesn’t have this capability.
It was a good segue to bring Navin back in too. To the point of having some sort of abstraction layer where you’re pulling in all this information in real-time and what Shruti does with Rockset to create this converged index where you’ve got multiple different indices, you converge them and then you can query on that as it happens.
Think about when Google will go and update its indices of websites. If you’re a news organization and you’re constantly throwing content out there, they will dynamically search you more often. They’ll figure out, “What is the rate of change on this website?” You’ll get penalized if you do it a bunch and then stop doing it and then start doing it again. You can climb back up but I promise you, some algorithms are tracking that all the time. if you have to wait 1, 2 or 3 days until something shows up, that’s not as exciting, especially if you’re trying to solve some problem in real-time. Go quickly, Shruti, then I’ll bring in Navin. Go ahead.
[00:30:03] Shruti: Indexing is such a great example for both Google and Facebook. It’s the same thing. Believe it or not, many years ago, Facebook’s newsfeed was batched. At some point, you would have to log in and look at it in the morning. It would stay the same until the end of the day. The minute they went to real-time is when the engagement started happening.
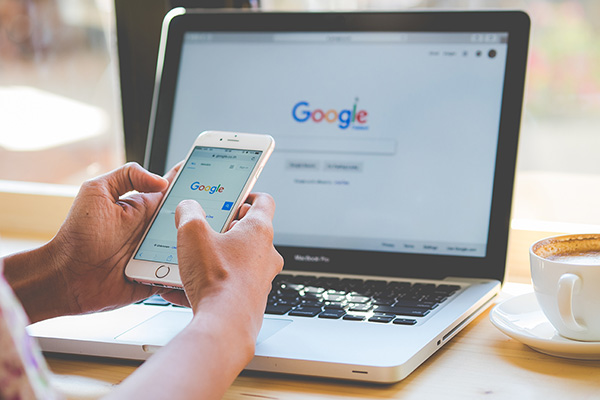
How Analytics Optimize Operations: Indexing is such a great example for both Google and Facebook.
It’s a good analogy when we think of Netflix and Facebook and what they’re doing at the petabyte scale. If they can manage that, why can’t we bring that to everybody but without the cost and complexity and without having to hire thousands of people to run your data systems? That’s not going to be affordable for everybody. How do you make it accessible to every citizen out there but without the cost and complexity? That’s the real challenge for all of us that we’re trying to solve here.
[00:30:52] Eric: Cost and complexity are real things. Everyone has a budget. We were talking before the show about resource groups, data mesh and all that fun stuff. We’re getting there but historically, it has not been easy to understand how much this thing costs me. I get my Snowflake bill and it’s like, “Holy Christmas.” They’re getting closer and it’s because of observability and lots of different things that are happening. Navin, I’ll bring you back in to comment on that. Everyone has a budget and needs. They need to understand how this is going to fit into their environment. What I like about your approach is that you are enabling analytics in a defacto-federated world.
[00:31:33] Navin: You talk about cost and complexity. Every source that you need to bring in requires some level of data pipeline build. Every data pipeline build requires ongoing transformations, maintenance of pipelines and specialty in terms of data engineering teams that need to be in place. That becomes that much more challenging.
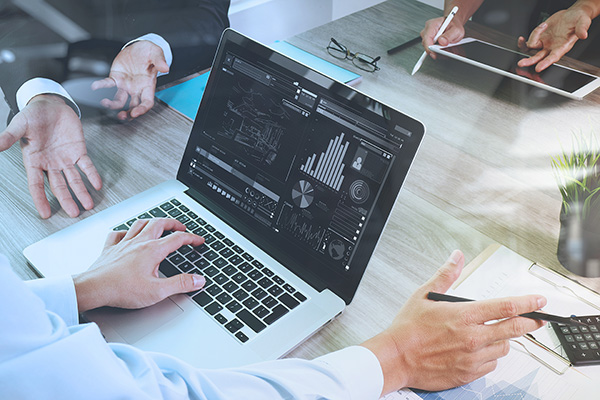
How Analytics Optimize Operations: Every source you need to bring in requires some data and pipeline build. Every data pipeline build requires ongoing transformations, maintenance of pipelines, and specialty in data engineering teams that need to be in place, which becomes that much more challenging.
More importantly, at the end of the day, you build the data infrastructure. What you’re still lacking is the ability to address the needs of the larger business. We talk about this notion of democratizing data for more users, except we are still having the users rely on the IT teams and data engineers to feed pipelines into their BI reports, dashboards or applications. It’s based on questions they know to ask but what we are trying to enable for users oftentimes is they don’t know what questions to ask. They want to be in a position to do discoveries over the data landscape that exists and do so in a way that’s more meaningful in the context that they’re looking at.
[00:33:33] Eric: I’m talking to three experts all about real-time analytics, operational analytics, different technologies and different approaches. We all have the same end goal. We’re trying to figure out what’s going on. I’ll throw it back over to Navin Sharma from Stardog. You made such a good point about knowing which questions someone’s going to ask. In the modern world, especially with this modern data stack with the cloud and all these different applications that are still on-prem, you don’t know which questions you’re going to want to ask.
You want to have versatility. I call it discovery. Discovery is one of the most important parts of this equation because if you can’t have some interaction with the data or the environment, you’re going to have a hard time answering questions. I’ve seen some graph databases where you have to go to the command prompt to click. I’m like, “That’s fine for the power user. It’s not so good for everybody else.” What do you think?
[00:34:32] Navin: I agree. We’ve always talked about enabling people to be data-informed and improving data literacy in the organization. Yet, we still find that we go about it traditionally. We got to bring data into some relational data structure. We know in the relational database context, it is a misnomer. Relationships are not first-class citizens in a relational database. In the real world, the way you model information is many-to-many. It is not one-to-many. It is not one-to-one. Those are optimizations we’ve built for the underlying way we store data in a relational data structure. In the way we think about things and concepts, there’s a high degree of connectedness in that data.
Google coined the term original term knowledge graphs. The idea was that when you search for things in their search engine, they present related ideas or concepts on the right-hand side of the panel. You may not know to ask the question until you see there’s more information that you can glean from this to get additional context. That’s what we’re trying to do. We’re trying to enable that experience in a larger enterprise.
If you’re a life sciences company and you’re doing clinical R&D research and you want to be able to pull data from your trials, from your understanding of the composition of the drugs, the molecules, the proteins and the make-up of that from molecule to market, adverse effects that are being reported and bringing that back to the specific molecule or compound in the related drugs that might also get impacted is a tough, rough cycle to go through painfully in a relational database construct.
[00:36:19] Eric: I often think about how I recollect things. Everyone’s brain is like this. There are some people more than others maybe but it’s very associative. If I’m trying to think, “What is the name of that movie,” I’ll think, “Which actor was in the movie? This other actor was in the movie.” I then have two pieces of information that I can use. I’d be like, “What movie were these two people in?” I’ve got it.
It’s so important for analysis and discovery to have that flexibility. The brain works like that all the time. You have all these associations. You don’t even know all the background there but they do facilitate and get you to the meat. You’ll have an idea like, “What is it I know that I’m almost there?” You have to do a couple more queries and then you figure it out.
[00:37:01] Navin: That’s the power of putting data in context for business users to do that and enable that discovery. That’s critical for an organization, the size of a life sciences organization or even financial services or operational risk. You look at fraud and AML. You’re talking about transactions, events, counterparties and even derivatives of securities. Securities have their derivatives. The collapse of 2008 was the fact that these securities had these derivatives hidden under them. They were exposed and no one knew how big that exposure was.
It’s being able to trace the lineage of that. I’m not even talking about lineage in the context of the metadata but the lineage of those assets is an important and big problem. To be able to address that, you need to work with data that’s in that model in a way that has those many-to-many connections. Your relational data structures are not designed for that.
[00:38:03] Eric: You gave me a thought here. We have a good question from an audience member too which we can get to in a second. Maybe I’ll weave it into Nick here. There is this question about business processes. You think about the supply chain, manufacturing and planning group. You talked about this earlier. If you have something like COVID come along, you recognize, “We’re not going to be able to get these parts from China, Japan or some other place, what impact is that going to have on my bottom line? How do I need to reorganize my company in terms of personnel or technologies to be able to absorb that blow and pivot?”
Especially when you talk about manufacturing or any big box retailer, these are real problems that occur. With COVID, we did have some leading indicators here. There were tariffs in the last administration. That should have given some people cause to re-think their processes and get better awareness as Navin is talking about. That takes a view across information systems with data that does not fit into rows and columns very neatly. That requires you to be able to do scenario modeling around, “What if we stop this production on that line? What if we spin up this production?” Those questions can only be meaningfully answered if you can see across systems and understand those processes.
[00:39:23] Nick: To Navin’s point as well, known questions are one thing. You can go through a huge data modeling exercise to get things in the right shape but it’s the unknown questions that are going to kill you. When COVID came along and started to disrupt the supply chains, a lot of people were left hanging because they simply didn’t have access to all of their operational data across these different fragmented silos.
You can go through a huge data modeling exercise to get things in the right shape, but the unknown questions are going to kill you. Share on XBeyond finance in Incorta, we see a lot of operational data coming from the supply chain process. We have a lot of customers that use us for inventory management and order management and bridging the gap between the two. At a glance, you can determine whether your demand is at risk or when items are likely to be back in stock. You then understand which inventory items are likely to impact that revenue forecast. It’s not only the ability to do basic SQL querying or analytics but it’s also getting into those more advanced forms of analytics, predictive and maybe prescriptive analytics as well. It is being able to apply machine learning across these multiple silos of data without the effort of having to pre-model and think of every possible question.
[00:40:26] Eric: Shruti, I’ll throw that over to you to comment on. The world is changing constantly. It always has been but the rate of change is so much faster for lots of different reasons. That means businesses need to be able to react. You need to be able to collect the data that you want and need that runs your business, analyze that and then make changes.
Think about budgets. Who’s done an annual budget? Do you do a whole annual budget for the whole year? You do it for a quarter or even a month. I’ve seen very short cycles because you don’t know what the future’s going to bring, whether it’s the revenue side or how to better manage your expenses. It’s a very fluid dynamic environment. You need real-time or close to real-time analytics in many use cases.
[00:41:13] Shruti: You used the word absorbability in the context of SREs before. I would go so far as to say you need business absorbability. You need to do whatever they’re doing on the machine data of your business. You need to be able to react within seconds and say, “This is what’s going on in my business. That big deal that I thought was going to come in is not coming in. I need to go and see what else is in the pipeline and act faster. That big shipment that I was expecting, for whatever reason, whether it is a hurricane or COVID, it’s not coming in on time. I need to be able to track that in real-time.”
Eight percent of the cement mixers in the US are tracked on Rockset. When it’s raining and your contractors are waiting, how do you reroute your cement mixers in real-time? All of this, I call business observability. We’re looking at a pretty tough time ahead of us as an industry. Everybody is worried about what’s going to happen with the markets. Are we looking at a downturn? Is it officially a recession? You got to be even more adaptive. The flexibility you need is on two sides.
Eric, you talked about data modeling. With the data coming in, suddenly somebody adds another column or another type. You can’t be sitting here asking your database, “What happened?” You can’t be doing all the tables and adding columns every single time. It needs to pick up the changes, react and not break your pipelines. That’s the data flexibility. The query flexibility is you need to be able to ask whatever questions you want without having to go change your pipeline. That’s how we think of it in the converged index wall. Both data flexibility and creative flexibility are important for real-time analytics.
[00:42:56] Eric: It is the business observability. That’s the real bottom line. That gets very complicated at large organizations. That’s why we need these technologies. Look these guys up online. It is time for the bonus segment here on a fantastic show. Maybe I’ll go in reverse order. Navin, I’ll throw it over to you. You are doing such interesting stuff. I like the way this knowledge graph facilitates new questions and discoveries. To me, that discovery side is so important.
There are some cool vendors in discovery. ThoughtSpot does a good job of allowing you to throw questions at data and get some information back. There are a few others that do a pretty good job. The beginning and the end of the process is the discovery. Talk about why that’s important and how your technology facilitates that.
[00:43:57] Navin: The underpinning of our technology is an enterprise knowledge graph platform. We have our roots in the semantic web with open standards described by W3C, which was all meant to describe the way we talk about things in the World Wide Web arena. We have distilled that down to the problem of enterprise data silos.
The idea there being that you have to work with information silos is never going to go away. Rather than trying to constantly work at centralizing all the information before you can do something with it, let’s start with the idea. What is it that you want to do? Describe your set of concepts that are meaningful to you as a business or for your use case in your context and then let’s get to the information regardless of where it is, how it’s stored and where it’s structured. You can then annotate that with rules, your definition of quality and access policies.
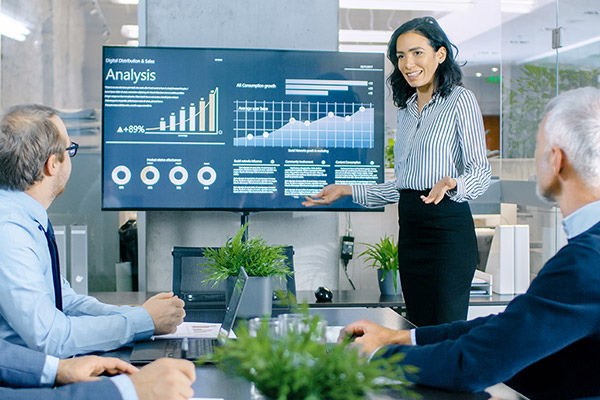
How Analytics Optimize Operations: Describe the concepts that mean to you as a business or for your use case in your context.
More importantly, this makes it easier for you to then reuse and inter-operate because it’s based on open standards. You can work on cross-organizational boundaries. If you’re working in a larger ecosystem with other partners and suppliers, there is the notion of ontology. Those ontology concepts are the basis of how industries collaborate. In the context of financial services, there is FiBL and financial instrument ontology for financial services. There are industry ontologies specific to drug discovery. Believe it or not, there are industry ontologies specific to superheroes. The Marvel Universe, what Disney shares and what Warren Brothers have from a media perspective build ontologies.
The autonomous vehicle is an interesting use case we’re seeing a lot more of. They’re beginning to think about transportation and mobility. They’re building industry-standard ontologies because they’re going to share that with their partners. You can’t cooperate in a silo, especially when you talk about autonomous vehicles.
More industries are beginning to participate and collaborate. Working with domain-centered ontologies that allow for that collaboration makes it that much easier for you to work across cross-organizational boundaries, more importantly. Lastly, you can reuse it. There’s a lot of reuse and sharing of what you have without worrying about what the underlying data storage is. If you want to move to a new disruptive technology, that’s great. Everything is still contained within the model you’ve already defined.
[00:46:19] Eric: That works perfectly for our TV show, which is called Future Proof. The concept was in five years, you’ll look around and see proof of what the future was five years ago when they were designing it. That was the whole concept of the show. Shruti, I’ll throw a question over at you. It’s an interesting question from one of our attendees saying, “What if you simplified what you need to know by understanding the biz process?” Let’s say you want to track a few hundred interactions in a process in real-time. You don’t need to until you get at the interactions of what people do in the process.
In the risk management world, we call those control points. At a certain point of the process, either a human or a technology can step in and make a decision, refine a decision or do something. A control point is a point at which something can change. That is something you do need to understand if you’re going to change your business.
[00:47:10] Shruti: Those processes are all evolving. We are talking about what we are going to see in the future. This is where we are headed. Everything is getting more automated and data-driven. I’ll give you a real-world example. We are working with one of the largest insurance providers in Europe. They are all about digital codes for your insurance. You don’t have to go submit your data and then wait for somebody to come back to you with a code. You can go shop around in real-time and get competitive codes from multiple insurance providers.
They found that they were making a classic mistake with that real-time data, which is they were making certain assumptions and not giving the best possible code. It was either 1 of 2 things. They lose out on that customer or they’ve given a code that’s not accurate. That is a classic business operation. The control point there is how you provide the code at that particular moment.
What they’ve moved on to is real-time analytics digitize that process. How Rockset steps into this is they take the data from the customer that’s coming through Kafka streams. They go join it with their operational database, which is an Oracle and they are able to give you a real-time code that is very accurate based on your data and their business and make the most competitive offer for you.
I see this happening everywhere. Every industry is getting more digitized and has more process automation. I only see these empowering operations people. Those are the same people who are still running the insurance operations but they’re able to do a lot more efficiently and accurately because they have real-time data.
[00:48:54] Eric: Nick Jewell, I’ll throw this over to you because this question came in around process awareness. I remember there was a guy named Dean Stoecker who started a company way back when. He talked about process awareness and process intelligence. They are a company called Alteryx. What are your closing thoughts, Nick?
[00:49:11] Nick: I enjoyed the conversation. The idea of real-time is still quite nebulous for many companies. I’ll define it and say it’s almost an ever-decreasing micro-batch. We’re getting closer to zero but against complex ERP systems. With the status quo for most companies where they have underserved analytics teams, they do their extraction and transformation. They’re loading and answering known questions in a known way they’ve done for three decades. If you’re lucky, you get your reports by 9:00 the next morning.
Within Incorta, we see excitement from customers. They get to move from that legacy starting point. They get to load their data more than once every 24 hours. They get to move to have refreshed data every 15 minutes or 5 minutes. It is an ever-decreasing micro-batch. The culture that changes from the ability to have 96 times more frequent refreshes every single day, they’re no longer waiting for that batch load to fail overnight. They can make intra-day decisions with increasing regularity. I’d also say that if you can take a four-hour report and turn it into something that’s sub-second, you can start to ask tens of thousands of exploratory questions on that data. That moves the needle on an analytics culture.
[00:50:21] Eric: I love it. We’ll talk to you next time.
Important Links
About Nick Jewell
My passion is building communities of fiercely-enthusiastic individuals who become more than the sum of their parts. I love how technology can bridge the gaps in large, siloed organisations in unexpected ways. I thrive on the network effect and my role in making change happen.
About Navin Sharma
I’m a self-described intrapreneur, a seasoned Product Management Executive, who thrives at the intersection of technology innovation and business challenge to create value for both the employer and the customer.
About Shruti Bhat
I lead product management, product design, product marketing and demand generation at Rockset. Prior to Rockset, I led product management for Oracle Cloud, with a focus on AI, IoT and Blockchain. Previously, I was VP marketing at Ravello Systems where I drove the start-up’s rapid growth from pre-launch to hundreds of customers and a successful acquisition. Prior to that I was responsible for launching VMware’s VSAN and I’ve also led engineering teams at HP and IBM.
analytics Data Storage Infrastructure Knowledge Graph Optimize Operations Production Database Query Latency