Strike Up The Band: Data Orchestration Takes The Stage With Divyansh Saini, Chris Sachs, And Taylor McGrath
If you haven’t looked into data orchestration to streamline your data analysis, listen up. Joining Eric Kavanagh in today’s episode are esteemed guests here to discuss a new spin on data orchestration. “The whole world is up for grabs right now with respect to data!” notes Houseware Co-Founder Divyansh Saini, whose technology gives business users direct access to data-driven metrics in Snowflake. And when they say metrics, they’re referring to the actual, live data of the business, thus (finally) empowering business people to quickly and easily cobble together meaningful views of what’s really happening. Check out this episode of #DMRadio to learn more about it with Saini and two special guests: Chris Sachs, Co-Founder of Swim, whose company is reinventing the entire data supply chain, opening the door to actual real-time in ways never before seen in this industry; and Taylor McGrath, VP Data Labs at Rivery.io, whose company is simplifying data orchestration for business users across the board!
—
Transcript
[00:01:01] Eric: The topic is a very interesting one. It’s one I’ve been tracking for years. It’s a new concept but it’s more of a culmination of concepts and the topic is called Data Orchestration. What does that mean? It implies lots of instruments. We have lots of kinds of data and systems moving data around.
The way that people have moved data around historically has been largely ETL, although sometimes you drop it on a disc and ship it across the country. You can do that too. You can do ELT, which is a newer version of ETL. It stands for Extract Transform Load and then Extract Load Transform came around. Years ago is when you first started seeing that.
That happened in part because you can preserve all the context of the data. A lot of people don’t realize that in the data world, if you look at data warehousing and business intelligence, in the old days, we had to strip out all that context to get the data through the thin pipes to get processed by this relatively small processors. Storage was expensive and you didn’t want to keep everything.
Most of that has changed. The cloud is a big part of it. If you talk to some experts about Snowflake, they’ll play DB. The cloud data warehouse is taking off. It’s amazing how much traction those folks have gotten in a fairly short period. We did a webinar with them around 2015 or ‘16 when they were starting and how things have changed. They’re not the only ones. There are lots of other data warehouses in the cloud. On-prem is here to stay.
The bottom line is people are going to want to move data from one place to another or at least give access to data where it is. I’m going to talk all about that with our guests. We have Taylor McGrath joining us, Divyansh Saini and also Chris Sachs. We’re going to learn about their different companies. Taylor is with Rivery, Divyansh is from Houseware and Chris is with a company called Swim. All of them are doing interesting things. Ladies first, Taylor, tell us a bit about yourself and what you folks are doing in the space of data orchestration.
[00:03:04] Taylor: I’m Taylor McGrath. I lead our data labs group at Rivery. That’s our internal data stack as well as a lot of our product evangelism and data advisory. At Rivery, we are a SaaS ELT platform. That covers areas of the data stack like ingestion and transformation, whether that’s SQL, Python or transforming the source of the files in a data lake itself as well as being able to activate your data and data viewer CTL back into source systems. Data orchestration is a huge part of what we do in terms of simplifying. We can orchestrate things, as well as being flexible to orchestrate easily consumable APIs with other best-of-breed arts of the data stack.
A metric we like to use is ‘time to new use case’. How scalable is your stack in terms of getting to the point where you can onboard a new use case that provides business value? Share on X[00:03:57] Eric: You folks came up along with a pretty clear vision to help harmonize and align these environments. Especially if you start working with bigger companies, they’re going to have 3 to 7 ETL tools, 2 to 7 data warehouses, lots of data marks and code that they write Python. Perhaps, they’re going to have lots of different things going on. That’s a real mess for anyone who wants to govern what’s happening, data quality and organizational change but it’s the reality of most scenarios in the real world. Could you talk about how you can help with your technology to make sense of all that and start to reign in the cats?
[00:04:40] Taylor: A big piece, especially bigger companies, is connecting the dots. A metric we like to use is the time to new use case and how scalable your stack is in terms of getting to the point where you can onboard a new use case that provides business value. It’s all of the pieces there in terms of if you need data, enrich and transform your data, make it operational by sending it back to some other system and then orchestrate that whole piece.
What is the simplicity of that? Flexibility is huge, especially with these bigger companies that have lots of incumbent systems where they’re truly integrated and part of their day-to-day operation. It’s not necessarily something that you sunset in 1 month and 2 months, even 1 year or 2. It’s being able to integrate and flexible enough to work with existing incumbent systems and also providing value quickly.
[00:05:43] Eric: You brought up a couple of good points here. I love this time for the next use case as a metric. That’s brilliant because that’s what matters to the business. It’s like, “You come up with an idea. You want to solve some problems. Let’s get the team together and find the data. What algorithms can we throw at it to ascertain what’s happening, make some prediction, for example, or classify organize or whatever it is?” You then set about doing it. How long does it take you to get that done and get onto the next use case? That’s a pretty clear metric to me. Who came up with that one?
[00:06:17] Taylor: Some genius on our marketing team for sure.
[00:06:21] Eric: You also mentioned Reverse ETL, which I’m seeing a lot about. It’s funny because it is reverse but there are lots of questions in the marketplace. Why wouldn’t I use my existing ETL tool or ELT tool to send things back the other way? The answer to that question lies in the complexity of the target systems and the capacity or throughput that these technologies have.
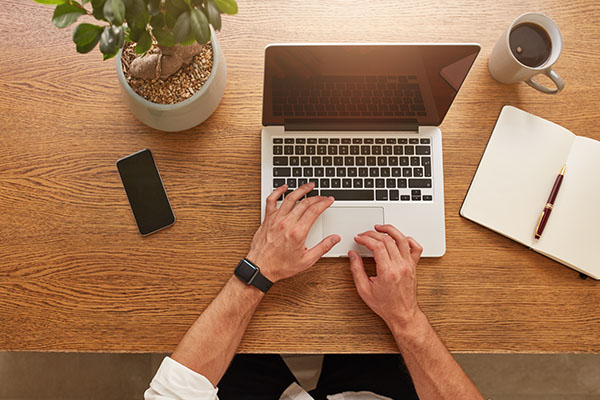
Data Orchestration: It’s being able to integrate and be flexible enough to work with existing incumbent systems while also providing value quickly.
You can have a technology that’s very good at doing one thing but not so good at doing something else. Tell us a bit about this Reverse ETL, which makes every last bit of sense in the world to me because you’re taking information from the warehouse and feeding it back into your operational systems, marketing system, Salesforce, HR or whatever it is. It’s like, “That’s what we should be doing.” Tell us a bit about that.
[00:07:12] Taylor: It’s a hot topic. More and more, we’re going to see a drive towards mixing analytical with operational. It’s inevitable, especially with these best-of-breed cloud data warehouses that are being more attuned to accommodate both. We’re going to see a lot of products built on top of the warehouse from inception to operational so being Reverse ETL is huge there.
You’ll hear diversity in data activation. The goal is to enable our end users or business users to make these data-driven decisions in their operational tools. You’ll hear things like the dashboard is dead. We need to have the information, whether it’s a recommendation or you need to act on this way in our operational platforms like a CRM tool, an ADP tool where the action takes place. That was the dawn of Reverse ETL and those types of use cases.
I will be very transparent. It will be a struggle or a battle between DWaaS. Reverse ETL is always going to be a data warehouse first. I’m sure Chris can speak on it too. Is it going to be extremely worthy? Is it truly operational? Are we going to send these direct from a source-to-target operational system with some real-time layer that does transformations or is it something where we’re going to take the opinion that our data warehouse is that layer? We’ll see both things grow but Rivery, as a product, is very ELT and data warehouse first. We’re using the data warehouse as a source for that Reverse ETL. I’m trying to be unbiased opinion for different use cases. They’re both very relevant. We’re going down the road of operational and it will continue that way.
[00:09:13] Eric: You gave me segues for both guests here. That was brilliant. What Chris is going to talk about is very interesting in a different way but let’s talk to Divyansh since we have a nice segue there on cloud data warehousing and Snowflake in particular. I came back from the Snowflake Summit, which was amazing. Guess what those folks are talking about? They’re talking about Unistore, building apps and bringing the code to the data warehouse. As I wrote an article about this, I thought to myself, “As a business person, I can’t assure to host my applications. Can I stand up a database in there?”
The answer is you can. This is a bit of a departure. Some savvy analysts think it’s a pretty clever thing for Snowflake to do because Snowflake has historically been the data warehouse. They have a very clean partner strategy. We manage the data. You do the analysis and build apps on top of it. That’s a brilliant way to go in terms of partner strategy because they’re doing it so well, at least, is one reason.
The entire world of data is up for grabs. Share on XBuilding in this environment brings some interesting challenges and ideally, it brings some real power too. Why don’t you tell us a bit about what you folks are doing because I find it very interesting? Let me characterize and tell me if I get it wrong. You are giving visibility into what you call metrics in Snowflake and then allowing business people to quickly grab these metrics and pull together views of the business, which is what analysis is all about. Tell us a bit about that.
[00:10:48] Divyansh: You mentioned Snowflake. It is such a core part of our strategy as well as how we think about it in Houseware. I’m one of the Cofounders of Houseware. The VVC asset is the data apps company. We see a lot more happening with trends around data orchestration and data activation, as Taylor mentioned, is that people don’t want to be using analytical data for analysis. They also want to use it for more operational and data activation use cases where all of this data goes into the sites of the data warehouse.
This is where it goes. Data is not used for anything apart from dashboarding and reporting, which is warehouse welcomes. It allows users’ inside functions like sales, customer success and marketing of a product to create these applications on top of it as internal applications in their organization. It combines the best of the breed of visualizations and activation into one single plane for them to talk to their users and take actions on top of it.
They started with a very simple question to us. “What would it take for us to flip the value of the data warehouse, which has largely been an engineering and a data team’s resource, to the people on the other side of the table who are essentially revenue function-oriented?” Another core defense we realized in the cultural difference between the users, the data team and the users on the revenue function is the language itself is very different.
The data team is always talking about tables, rows, columns, schemas and models, whereas in your revenue function, people inside sales product and customer success are always talking about metrics. Metrics are these first-class citizens that Houseware enables these users to look at and go deeper into. This is where the deal analysis happens.
[00:12:26] Eric: That makes a lot of sense to me. It makes it very business-friendly. What you want is for these things to be served like under-the-hood technology. You don’t need your business people to understand exactly how the ELT works or the ETL works. You don’t need them to be masters of SQL but you want the business people to know their business, what’s happening in the business and be able to pull from this trusted source, the data warehouse to get the numbers and wrap their head around something.
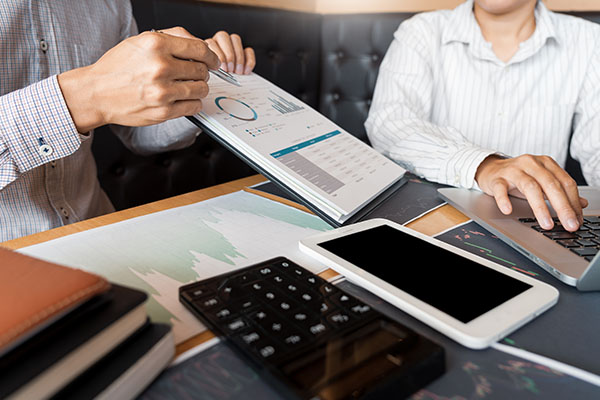
Data Orchestration: People don’t just want to be using analytical data for analysis. They also want to be using it for more operational use cases.
You’re enabling the building of apps around that as well, not just dashboards and views. This is another huge trend I’m seeing. We’ll talk to Chris Sachs about this in the next segment but there is going to be an entirely new generation of apps that are coming out. They will be data analytics-driven apps, very focused on the business and it’s the next generation of business functionality. What do you think, Divyansh?
[00:13:18] Divyansh: The entire world of data is up for grabs. They keep repeating time and again. The reason why I say this is because, for decades, we have seen so much great technology come and be a provision to data teams inside an organization. That is ETL, Reverse ETL and the data warehouses themselves. You have simple machine learning tools, complex machine learning tools and modern-day notebooks of the world.
The data have the arsenal goal but if we go back to the basics and the fundamentals, the eventual goal is to optimize the processes and the outcomes inside an organization to increase revenue. There’s a very important need for a company and a tool to come in. They need to consolidate all of these efforts that have gone into the technological part of fueling the data team into creating an experience that is super important for these end users.
From an infra landscape, you see the Snowflake strategy and what they are going after. Snowflake Summit 2022 made it super clear from Snowflake’s perspective that application is a core part of their strategy. They also realize this need for the cloud data warehouse. Data cloud is super essential because you’re not going to be calling this $100,000 spent on a data warehouse to be data spent. You have to also talk about the business value that you’re giving to your end users inside the revenue function. This is where things like Unistore and the native apps framework bring the operational data along with the analytics data into one single plane, which allows companies like us to flourish on top of it.
[00:14:53] Eric: You’ve changed the business process in a very fundamental and foundational way, which is to stay in the old world. You would have some business person go and ask IT to give them access to another databook or have someone write some code to grab some data, for example. That’s fine but it takes time, it’s slow and this process is latent. Frankly, the thought process is truncated. What you’re doing is enabling the self-service usage of trusted corporate data. That’s in the warehouse to build the next generation of apps and views real quick.
[00:15:32] Divyansh: That’s exactly what we do. This is no longer the era where we treat our organization as siloed IT and siloed sales and marketing. We are living in this world where all people who are working in tech especially are by default technical. Whether you’re an account executive or DevOps person, you understand your core business so well that if you are given the right tooling, you can go ahead and pretty much do business at the speed of thought and don’t have to wait for a business request to be going into Jira.
If we go back to the basics and the fundamentals, eventually the goal was to actually optimize for the processes and the outcomes inside an organization to increase the revenue. The whole purpose of running at the edge is to reduce latency. Share on XThe team is thinking and taking their sweet time to come back saying, “This data is not there. We’ll probably take a lot of time to get this on the warehouse and then go from there.” The readily available data access on the data warehouse is something that we extend to our users and make it available very quickly.
[00:16:26] Eric: You give them access to these reports, not only reports but the metrics themselves, which are the data running the company.
—
[00:17:53] Eric: We’re talking all things like data orchestration. We’re going to talk about some real-time data, some data movement, edge computing and all kinds of fun stuff. I’m reminded of another fun quote when I was interviewing Dr. Michael Stonebraker on this show years ago. He was the godfather of the modern database or at least the Postgres database. He said, “Ninety percent of the code should be thrown away.”
His point was that the code running all these applications from way back when was designed in a much different world where you had to wait for a spinning disc, slow clock speeds or whatever it is on the inside. The workings of the machines and the operating systems they compiled for, much of that stuff has changed certainly in the cloud. The edge is almost cloud 2.0 if you break it all down. Next up, we have a fascinating character, Chris Sachs from Swim. Tell us a bit about yourself and where this all came from.
[00:18:56] Chris: Thanks, Eric. It’s good to be with you. I’m the Cofounder and CTO of Swim. At Swim, we’ve built a streaming application platform. The idea is that we need a lot more than analytics, processing data and putting it into the database. We want to build autonomous systems that consume streaming data at high rates, put it together and act on it in real-time.
To be able to run or compress the whole stack from ETL, data orchestration, running general purpose business logic, taking autonomous action, providing visualizations and observability for end users as quickly and directly as possible in a single vertically integrated stack. That’s Swim in a nutshell. We’re trying to pull the rope as tight as possible in the fastest, most direct way that we can analyze, understand and compute data to do useful stuff with it at the speed of reality.
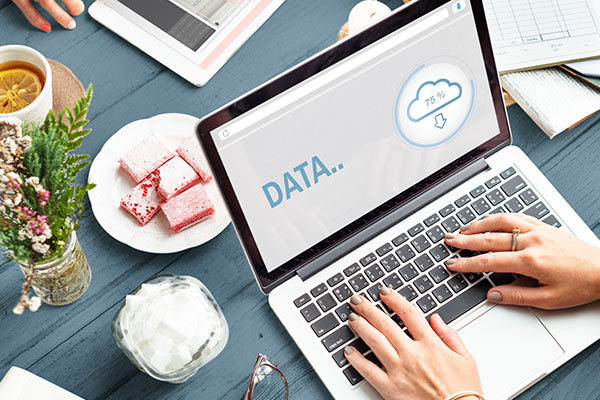
Data Orchestration: The data cloud now is super essential because you’re not just going to be calling this a hundred thousand dollars spent on a data warehouse to be just as “data spend”. You have to also talk about the business value that you’re giving to your end users inside the revenue function.
[00:20:01] Eric: You talk about how you started as an edge first company and out at the edge, you think about design points. When you’re working with trying to solve some big of the edge, you have to be very lean. It’s like the old days of programming. There’s this concept of code bloat that is processors got faster. Developers could get lazier writing a whole bunch of code to do something instead of writing it nice and tight, which is what it always should be. Ideally, you want your code as tight as possible. You want as few functions as possible to get the job done. You all cut your teeth out the edge, which taught you a lot of lessons. Tell us about that.
[00:20:42] Chris: We come at this data orchestration picture from a different angle. Swim started as an IoT automation platform where we would run distributed control logic out at the edge controlling smart lights and cameras. In binding necessity, we had to integrate a lot of the stack because the devices are more constrained but also because you’re very latency sensitive when you’re out. The whole purpose of running at the edge is to reduce latency. When you’re dealing with control and automation, you need to be coherent with the real world if nobody wants the light to turn on after they’ve left the room.
When you go to the cloud and in larger automation systems, you need to be real-time. If you have a network that goes down and you decide you want to restart a router, you don’t want to restart that router an hour later when the thing has already started working again. We had to compress the stack so that we could run on the edge. It turns out that there were a lot of incidental benefits of compressing the stack that help elsewhere.
[00:21:48] Eric: I heard you describe how you viewed the world. You did think through the entire end-to-end process and how much things have changed. You realize we need to build something from the ground up to reinvent the wheels because, for certain solutions at scale, you can’t optimize one piece. You were joking about how so much of the data world winds up becoming defacto Band-Aids for the mistakes we made last time and how many Band-Aids can you wrap around the cup before it leaks all out and you lose all your data, water and money? You had a very unique approach to the whole solution here and you re-architect it. Tell us about that vertical stack, what those components are and how it works.
[00:22:36] Chris: You have to get down to brass stacks to work in real-time. You’re only going to be as fast as the slowest component. It’s also worth keeping in mind that CPUs are much faster than networks. A lot of the data ecosystem is very federated. You have lots of middleware components, databases, message brokers, job managers and application servers and they’re all connected by networks, which run millisecond latencies. CPU’s worked at nanosecond latency. That’s six orders of magnitude faster. If you want to run at the speed of data or run business logic as fast as the data is coming in, you have to keep everything local on hand.
It’s like paying a million percent tax to go and query the database. We’re talking about data orchestration here so we’re talking about many different sources of data coming in and they’re coming in at different times. For some of our larger clients that are dealing with 5 to 10 million events per second, data doesn’t have much meaning on its own. You need context to make sense of it. The key is to be stateful and stream throughout the stack. That’s what we’ve done at Swim. We build the whole stack and stateful so it doesn’t forget what it’s doing to do everything in the stream.
You're only going to be as fast as the slowest component. Share on X[00:23:58] Eric: I’m glad you mentioned that. This is going to be a good round table topic. For the benefit of our virtual audience, the state is a very important concept in programming. I try to come up with examples to explain. Think about when you’re shopping online. You’re browsing, select a few things and put them in your cart. That’s part of the state. The state recognizes who you are and the fact that you have these items in your cart and then you want to go check out. You go to a different state. I’m in the checkout mode and want to make sure it’s all correct and give it my data. If you look at a lot of the tremendous innovations that have happened in the past years, they are all built around a stateless view of the world because they want it to be able to be scannable.
We hear all about Kubernetes. We talk about Kubernetes all the time in the show. It’s fascinating technology. It is brilliant what these folks at Google came up with but it’s all stateless. State then has to be stored somewhere else, either in a database or some system of record. That can get a little loosey-goosey. It can get hard to manage if things fall apart or pull it back together. There are challenges associated with that. What Chris is talking about here is they took an approach to circumvent that huge chasm, which everyone else has to jump over. Is that about right, Chris?
[00:25:17] Chris: It’s worth pointing out. We’re having a stateful conversation. I remember what I said and you remember what you said. To put in perspective what a stateless system is like, imagine after every question you asked me, I had to go walk 60 miles to check my notes, which is a query of the database. Remember, it’s six orders of magnitude longer than it takes a CPU to think. Instead of 1 second for me to formulate my thought, imagine it takes 1 million seconds while I go.
I check my notes, come back and give you a response but you’ve forgotten what you had said so you have to go and check your notes and come back. That’s how most software works. That’s why nothing is real-time because you check your notes, do one thing, forget and then start all over. You can never get to real-time in that way. It’s not because of anything fundamental. It’s because of the history that happens in these kinds of applications we used to build. What the industry is trying to do is different. It calls for a different approach.
[00:26:25] Eric: In a second, we’ll bring in our other guests for the round table but you have so much innovation all up and down the stack. You think about the data persistence side, streaming data and Kafka came out of LinkedIn. It’s run by Confluent but even Kafka persists in spinning disk, if I’m not mistaken. It still does. Here, that’s not real-time. You’re persisting it somewhere and then accessing it from the database. This is how things have been for years. You could almost say we’re coming back all the way around to a situation like a mainframe. That’s what the mainframe was to a large extent. You bought little bits of time from the mainframe.
I can’t remember what they called but the point is we’ve seen so much innovation up and down the stack in networking, processors, hardware and software in all these devices. If you think about it, it takes time to build software or something. If you want to build what Chris and his team have done at Swim, you have to have the gumption to do so and take the time and effort to build this thing correctly. You have a target audience. You folks are very good in telco, where you have tremendous amounts of data. It’s important to know what’s happening when calls are dropped and things don’t work well. You’re cutting your teeth in that world but there are use cases across the board.
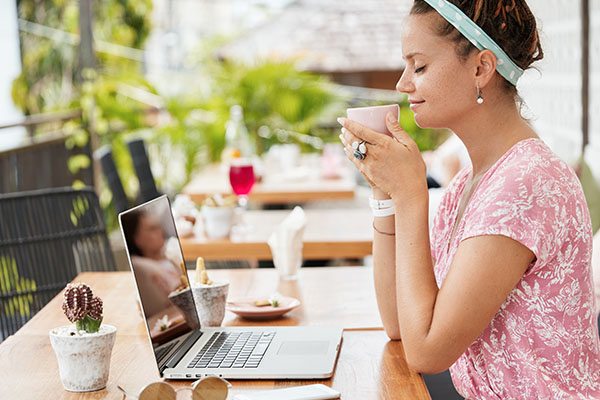
Data Orchestration: When you’re dealing with control and automation you really need to be coherent with the real world.
[00:27:48] Chris: If you look at the broad industry trends, time cycles are getting faster and faster across the board and telcos are particularly acute. Even if you’re a shopping application and you have pickers go into a store, if a store runs out of a product, you want to be able to redirect them on the fly or you might be making a drone delivery. Maybe in flight, you decide you need to redirect that drone. The trend is towards faster time cycles. In the traditional approach, it gets exponentially more expensive to do real-time because you’re always pulling lead systems.
I like to compare it to accelerating a photon to the speed of light. It’s never going to happen. It takes infinite energy because you keep having to query and ask but if you build a system to work a photon, you naturally do your computing as the data arrives and remember the context you need. You can’t help but operate in real-time. Reset that cost curve and the complexity function in the process because you’ve stripped out the layers of bandages. A lot of the complexity in the data space is trying to work around the fact that we’re stateless. You have caching servers and all of this that’s there to work around this core assumption that no longer applies.
[00:29:13] Eric: That’s a good way to put it. I’ll bring Taylor McGrath back in from Rivery to comment on this. My old co-host years ago used to always talk about horses for courses, meaning there are different race tracks. You want different horses to perform on a different racetrack because some are good in the mud, on the flats or whatever the case may be. The business will always have to make those determinations. There is something to be said for what Chris is saying about rethinking and reevaluating many of the assumptions that we made, how we built these systems and understanding there’s a new way to build them going forward.
[00:29:43] Taylor: I’m pretty impressed by what Chris has described. If I’m being very honest, it’s even further ahead than a lot of businesses, especially specific industries and when it comes to the data world that’s focused on analytics and getting their feet wet in operational analytics. It’s going to be very use case driven. Like the examples Chris gave, there is real-time retail in telco that already needs this real-time to have state applied. Something that will be ever important with these is that it’s treated like a true product. You have all the capabilities in terms of observability and the actual delivering everything around.
The definition of a product also has to be real-time. It’s difficult turning these use cases into real-time. That’s why we still see this data warehouse first mentality. You can treat these things as products because they are mostly batch-driven, have metadata and are accessible. The use case here will steer organizations in the way that they strategize in terms of if this is necessary to be real-time, a new age thing like Swim or something we can generalize our general population of users and the data warehouse.
[00:31:23] Eric: There’s only so much budget in every organization and so many things that can be done but one of the cool aspects of what Chris has built and the rest of you on the show as well is that we are in a very transformative period in this industry. It’s quite remarkable. I was talking to Mike Olson of Cloudera years ago about how there’s this redoubling. It gears around AI, awareness and self-learning systems that are not going to sit on top of all this data and do interesting things. We need to be able to be aware of all of that.
It's super hard in an analytics process to really see what the top line impact is on the revenue. Share on X—
[00:34:32] Eric: Divyansh, I was thinking to myself, I love this concept that Taylor throws out their time to next use case. That’s a good metric. Also, time-to-next app and time-to-next innovation. What we’re seeing here is we’ve talked about that cycle times are condensing. It used to take a year to stand up a data warehouse. Now it takes 1 week or 2 weeks, depending upon the size of your organization.
We’re going to see that starting to collapse in terms of new business processes, new functionality, new features or whatever it may be. To facilitate that process, it helps to see what’s happening and you’re giving easy use or ease of access to metrics inside of Snowflake, which can then be used to help the business people figure out what to do and what next app to build. Maybe it’s going to require real-time of what Swim provides or it doesn’t. That will depend upon the use case and business needs. My point is that we’re getting somewhere in terms of giving the business what it needs to know and what should be done next. What do you think, Divyansh?
[00:35:36] Divyansh: I love the time-to-next use case as the North Star metric to go towards specifically for a company Rivery. We think about these things in Houseware. How do they use this latency for the business? You also need to be able to measure the business value that you’re getting on the other side. It’s super hard in an analytics process to see what the top line impact is on the revenue. You can always think about metrics in their essence that you see like an increase in the daily number of orders. That still doesn’t mean that the use case you spent six hours working on increased the number of orders. Attribution is a big challenge our customers face and the warehouse helps not just to see these individual metrics.
There’s a click on an app to several orders but also see the relation between them. It’s a complex, hard problem that our customers tend to think of. Creating their apps on top of it and then thinking of things like the time-to-next use case comes into the context for our customers. More of what we’re seeing is consumers in their personal lives expect an experience like Uber, where they click on the cab and book these cabs at that very moment.
You don’t wait 30 minutes, it goes into a queue and then you see the cab is getting booked. Something similar in business metrics is it’s time to change. It’s no longer a time where you want to be seeing your daily number of active users as of the last day. People are coming to the last fifteen minutes and how we can bring it much closer to the last second is critical. That’s how we and our customers think about this as well.
[00:37:16] Eric: Taylor, I’ll bring you back into the equation here. A lot of it it’s going to be consulting or talking to the business, whether it’s an internal business analyst or the process analysts, for example. The hardest part is going to be shedding old mindsets about how things have to be. There is a certain point at which you have to rip the Band-Aid off and deal with the immediate pain to get deeper and solve things for the long-term because this scale-out world is very different from the world in which many of the applications that are running a business were designed.
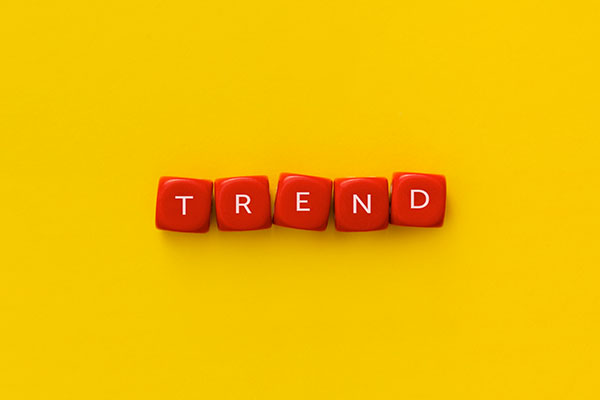
Data Orchestration: The trend is towards faster and faster time cycles.
[00:37:51] Taylor: It’s a good point. I forget the exact quote but something about the danger of incrementalism. You’re slightly incrementing again and again that essentially you don’t extract any change. An example of that is companies are like, “We’ll move this one component to the cloud. We’ll do this thing in real-time. We’ll make this one little piece better.” It’s revisiting data strategies and even operational strategies in terms of the technologies currently out there, what we want to serve and making those decisions as opposed to changing one thing at a time.
[00:38:47] Eric: We have some good questions from some of our members in the audience. One, I’ll throw over to Chris. We have an attending who says, “I developed Beijing predictive analytics stub apps to think in terms of high-frequency trading scenarios and business dynamics. Can a Swim platform accommodate this? I’m thinking that’s exactly what you do.”
[00:39:03] Chris: Part of our strength is the ability to run general purpose compute at the speed of data, whereas a lot of traditional analytics systems require these pure functional approaches to developing analytics. In one of our use cases, we run the self-training neural networks where we’ll take data from traffic management systems about traffic lights. We feed that into a neural network and guess what will happen a second from now. We wait and see what happens and we measure the error. Interestingly, we can run a training and prediction cycle in less time than it takes to get a packet out on the network. It shows how expensive it is for computers to access the network. Beijing inferencing systems are a great use case for us.
[00:39:54] Eric: I’ll bring a hunchback into the conversation here. It all comes down to using the data intelligently to come up with some new ideas. The discovery side of that equation is so important because it helps generate ideas and gives the business people some nudge that they can understand and then use to cast a new net or come up with a new plan.
The closer you get to the front lines of the business, the more people will understand the nuances of that particular domain. I can tell you that the data-driven economy that we have is changing fast. Consumer behavior changes quickly. I noticed, as a marketer, that I’ve been doing direct email for many years, which is a pretty long time. Email is still king in the enterprise. In my opinion, it’s still the best way to get people to transact with you and do something.
Social is great for eyeballs but behavioral chat patterns are changing, especially when you have a pandemic comes along. That was a huge forcing function. My point being unless you have your finger on the pulse of the data of your organization, you’re probably going to miss a change. If you miss two changes, it’s like Calculus class. You’re not going to be able to catch up but what do you think, Divyansh?
If you're just slightly incrementing again and again and again, you don't actually exact any change. Share on X[00:41:16] Divyansh: One of the consumer behaviors that we have seen change with the advent of the modern stack has largely been around how data was always daunting. If you think about data, you always have this concept of big data. It’s on Hadoop somewhere. Someone in IT is accessing that. Now, it has become a lot friendlier to users as they can think about things in terms of tooling remedy and essentially enables us into the operational systems and systems that are familiar to them.
Something like Salesforce or Zendesk is a big trend that we index a lot on with our customers. We’re seeing them use that a lot. More importantly, what these tools have been allowing us to do is that these metrics are much more familiar to anyone inside the business. Missing that pulse is super harmful to any business.
If these metrics are then available to them and they’re operational tools or they’re able to get in front of the users than taking a decision inside a meeting room is the most important aspect of running a data-driven business. I’m seeing that a lot more often, especially with new wage industries like media entertainment and SaaS.
These companies are leading the way in terms of how you can hyper-personalize the customer or user experience and not invade the right into people’s inboxes and see if they’re coming back to you. That’s super keen on and almost seeing that change drive the next generation of tools that are getting built from a consumption experience perspective or how this data enables these business users on the front line.
[00:42:46] Eric: That’s a good point. Media companies are almost at the end of our show here but a big thanks to our guests. I’m thinking Netflix. Netflix lost a million subscribers in their most recent report. You’re like, “There must have been leading indicators for that. There must’ve been signs leading up to it.” Chris, I’ll let you take a 60-second shot at that. They need real-time analytics.
[00:43:12] Chris: They do and they need a lot of contexts. The signal doesn’t stand out if it’s sub-sampled. Sometimes, there’s not a lot of lead time and also, they get averaged out in the tyranny of averages. When you’re not real-time, you end up sub-sampling, you average it out and you lose that signal. Even if a human doesn’t need to know continuously, a lot of times, your analytics benefit, you get higher resolution and those signals reappear.
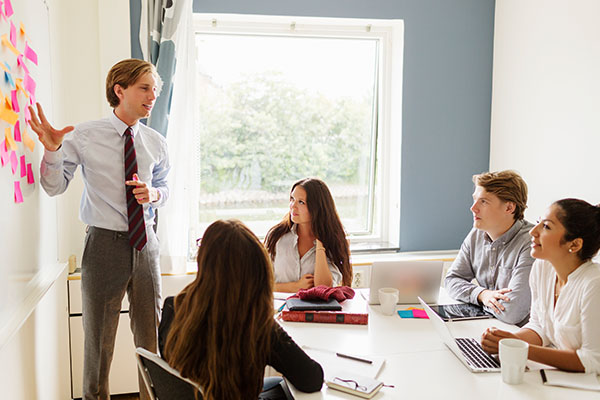
Data Orchestration: The use case here really will steer organizations in the way that they strategize.
[00:43:45] Eric: They didn’t know that thing called bias. There’s confirmation bias and ethical bias. You want to be able to get some contrast and things but the days of me thinking that I’m right and not caring about the fact that I’m wrong are coming to a close in the business world because there’s not going to be any room for errors that big. You need to know what your customers want and what your prospect is looking for. You need to act on it pretty darn quickly. Look at these folks online. It’s always a pleasure to talk to experts.
Important Links
- Snowflake
- Rivery
- Houseware
- Swim
- Jira
- Dr. Michael Stonebraker – Previous Episode
- Confluent
- Mike Olson
- Salesforce
- Zendesk
data analytics data supply chain data-driven metrics ETL operational analytics technology