Crystallizing Now: Data Science Comes Of Age With Gurol Kurt, Mary MacCarthy, And Zohra Ladha
With every passing day, the discipline of Data Science becomes clearer. Thanks to the hyperscalers plus the availability of proven algorithms, practically any company in the world can get involved. How so?
Check out this episode of DM Radio to find out! Host @eric_kavanagh interviews several guests, including: Gurol Kurt, Alterna CX; Mary MacCarthy, Hightouch; and Zohra Ladha, Tredence. Together, they break down what data science really means, why businesses need it to drive their processes, and how it is shaping our world. More and more, we are seeing data crystallizing into a force whose impact can no longer be ignored. Let this conversation reveal insights as data science comes of age, providing you with the information you need to grow alongside it.
—
Transcript
[00:43:06] Eric: We’re going to talk about a hot topic in our industry. It’s been crystallizing for the last few years now, quite frankly, data science. The title is crystallizing now. Data science comes of age. We have talked about it for a number of years and data science is more than predictive analytics for example or groaning algorithms around. Data science is the assiduous management of data assets at scale for the benefit of an organization.
We’re seeing data science platforms become robust. We are seeing a lot more companies understanding the end-to-end process. We have had predictive models for years. This is nothing new. We have had lots of different component parts of technologies, but it is coalescing into a cohesive picture to understand what it is. In the past, we have done shows like Data Science Versus Data Management, Will One Subsume The Other? I’ve argued that data science probably should subsume data management at least to a certain degree if you’re doing it correctly because what is science?
I’ll give my take on this, my elevator pitch, if you will. A lot of folks believe that science is a representation of the real world that is perfect and inaccurate, and that’s not the case at all. Science is a discipline. It is underpinned by a methodology which we call the scientific method and incumbent upon the scientists is the responsibility to do things in an orderly fashion to keep track of things. Don’t change more than one variable at one time, for example, because you want to be able to repeat what you have done.
I remember hearing some people talking about the repeatability problem and data science. I’m like, “If you have a repeatability problem, you’re probably not doing data science. You’re probably doing something close to some approximate nowadays’ science.” If you’re doing things scientifically, you want to be able to repeat them because you want to understand what’s happening and what you’re doing. You can optimize whatever process you’re looking to improve.
We’ll talk about all those things now with our several guests. I’m excited to have Gurol Kurt from the company Alterna CX. We’re also going to hear from our new friend, Zohra Ladha, from the company called Tredence, and last but not least, Mary MacCarthy is on the show from a company called Hightouch. They’re doing reverse ETL. We have got lots of different pieces and parts of the picture. Let’s go around the room and get introductions from everyone. First, Gurol Kurt, tell us a bit about you, yourself, and what you folks are doing at Alterna CX.
[00:03:06] Gurol: Thank you for having me. I’m glad to be here together with Zohra and Mary. I’m a Cofounder of Alterna CX, VR, and AI-based most customers’ solutions. We help companies generate promoter customers and grow them to upsell and cross-sell offerings and retain them. We do treatment to help them. We capture structured and unstructured data everywhere at every touch point.
Secondly, we use our AI models to make predictions on what is driving satisfaction and what are the root causes of satisfaction. Finally, we trigger actions that are necessary and most relevant improvements action whenever we come across a dissatisfied customer. We close the loop, in other words, and that’s how we help our customers by making use of our AI technologies.
[00:03:51] Eric: Let’s go around the room here. We’ll talk to Zohra Ladha from Tredence. Tell us a bit about yourself and what you’re doing at Tredence in the broader data science space.
We can analyze the data as much as we want and create a myriad of data in terms of insights and quantity, but when you don't take action, it's no good for anybody. Share on X[00:04:01] Zohra: I work at Tredence and I lead the data science practice with Tredence. It’s the data science solutions and an AI solutions provider. We work with a variety of Fortune 100 and Fortune 200 clients to use AI to solve their business, social, and economic problems. One thing that Tredence specifically focuses on is the last final adoption, which is about bridging the gap between insight creation and value realization, to delivering and measuring business value.
It is important when it comes to AI and ML because it’s all about using machine learning and AI to be able to deliver value and realize a substantial impact. If we’re not able to measure and realize that, most of the AI and ML are pretty much redundant. That’s something that doesn’t an organization we live great stress on, which is the last mile adoption.
[00:05:00] Eric: It’s important. It’s like getting into the end zone. You can drive all the way down the field. If you don’t punch it into the end zone, you have got your team tired, worn out, and probably a bit more bruised and you didn’t even get any points. Sometimes you got to take three points and get on the board, at least to get something. Last but not least, Mary MacCarthy is like Jill Smith in Ireland. I’ve got an Irish last name, Kavanagh. “Where did you get an Irish name like that?” One Irishman once asked me. I said, “From my father.” He accused me of being a Viking, but that was funny.
[00:06:10] Mary: Kavanagh is a very Irish name. I’m speaking to you from my home base in Los Angeles. I work as a Data Advocate for a company called Hightouch, which we all know the data warehouse is. Hightouch helps you get the data out of your warehouse through a process called reverse ETL or more broadly data activation.
I don’t strictly work in the data science space. I have studied data science. I love learning about it. I love hearing what Gurol and Zohra have to say. I will admit I’m also a data science skeptic. An awful lot of companies need is not data science, AI, and ML. It’s better data management, the basics of a good warehouse, and good processes. I may end up playing a bit of a devil’s advocate here over the next hour. We’ll see.
[00:06:22] Eric: I don’t mind that at all. I was hinting in my opening that understanding what data science is, is pretty important because if you don’t understand what it is, you’re going to spend a lot of money doing interesting things that may or may not help the business. That’s a great segue to bring Zohra back into the conversation because you talked about the last mile. You talked about making sure that you are getting business value from this.
If you can think about it, no application can survive without data. Every application needs data. No matter what it is or what it does, it needs some form of data to come in and go out the other way. We’re in this remarkable transformation that is industry-wide. Global companies everywhere are redesigning workflows to optimize the use of the cloud, for example, to use the edge.
There’s another architectural change to sort through. Zohra, if you could walk us through, when you talk about the last mile, what is treating deliver that allows you to provide a conduit for business change? You also talked about tracking. What are you measuring to help the business understand if this is working or not?
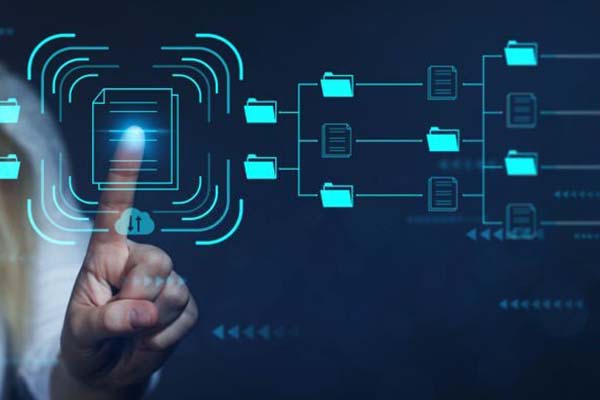
Data Science: What companies need is not data science and AI and ML. It’s just better data management, the basics of a good warehouse and good processes.
[00:07:26] Zohra: Data is the foundation for any data science that you do. We’re at a place where the data assets that we have are all there, in place, and ready to be consumed. They’re all integrated and synchronized. What we do at Tredence is we bring on a lot of our frameworks that enable us to build the data science flows more seamlessly. We leverage our experience across industries and clients to bring in a more generic framework.
When you specifically talked about how to ensure that what we are building drives value, that’s the starting point. That’s where we begin every time. What is it that you want to drive out of it? If it’s more about first defining the value that you want to drive out and looking at profitability, are you looking at customer satisfaction?
It’s going back to, “How do you solve this problem?” When you have that increase, the approach that we do is first, we define the object and the problem statement and then we worked towards solutions rather than the other way around, which is most of the time, the vanilla approach for building data science. That changes the game. You have an end objective in mind and you’re working towards that. You’re building the right analytics to drive that value, measuring that value, and realizing that value depends.
[00:08:56] Eric: You bring up a couple of good points here, which is, “Where do you leverage machine learning?” For the benefit of our readers, there are lots of different things that machine learning and AI can do. They’re increasingly interchangeable terms. There are some discrete differences, at least if you get down to brass tacks.
ML and AI are good at sorting through vast amounts of data and finding outliers. That’s one of the things they’re good at doing, classification. I view most AI as doing 1 of 2 things, generally. Classification is like grouping clustering, for example. These customers are in category A. These customers are in category B. There might be some overlap, but it helps you understand how to communicate to this group versus that group. The other thing is optimizing a decision point like pricing. Should we have this at $32 or $30? How much do we lose if we jack it up to $40? These are the things that AI and ML can generally do.
[00:09:52] Zohra: AI and ML can do this in much more. It enables the classification and grouping of customers or personas. It also enables this aggregation of persona in that way. It also enables you to get to that segment where each customer of yours is unique. If you’re able to identify that unique characteristic nature, which we called hyper-personalization of customers.
Optimization is also a great place where we use AI, but AI and ML now are being used to solve a vast majority of things, whether it’s supply chain problems, customer personalization, product pricing, transportation, larger states, climate, or weather predictions. It’s endless. I feel left out in terms of what you can do with AI.
[00:10:42] Eric: You referenced something that I picked up from my days working with SAP, which is a big player in the supply chain space. That is the supply chain of one. What they were talking about is this hyper-personalization thing, which is interesting. If you look at some of the bigger, more advanced brands out there, they will have models not just for a group of people, but for each person, company, or partner. They have to run the numbers and make sure you’re getting value from that. Talk quickly about the power of hyper-personalization and why that might make sense for a company.
It's not just about having the right data to make decisions. It's about being able to make the decisions in time. Share on X[00:11:16] Zohra: It does, but the world of data also find it important. With customers being exposed to so many things out there, differentiation has become even more difficult. It is talking about something unique to your customer, which is where hyper-personalization plays a big role. The end objective is always to be able to get to that segment of one. If you can identify that unique trait within a customer and the unique product or service that you want to talk to your customer about and the right way to talk about whether it’s the channel or the wording.
If you can build in that level of personalization, that’s where you bring in the differentiation and catch eyeballs. You’re able to grab the customer’s attention. It’s important in this world to aim for hyper-personalization and can drive personalization because with the flutter going around in people’s thoughts that people are exposed to that stuff, that’s the only way to stand out.
[00:12:14] Eric: Let’s jump back over and bring Gurol Kurt into the conversation from Alterna CX. What I love about your approach here. You are grabbing not just structured data, but also unstructured data. You think a traditional data warehouse is structured data. Back when people use the term structured data, usually what they mean is data in table format in a relational warehouse. That’s typically what you’re talking about.
That’s some percentage of data that matters, especially if you’re talking about customer experience. You want to be using natural language processing. You want to be using technology to sense the voice of the customer. If I’m upset and I’m talking to the customer service rep, there are technologies that can pick that up and go, “This customer is unhappy. It’s a high-value customer. Let’s bring in the big guns to make this person happy.” That’s the thing you’re doing.
[00:13:09] Gurol: In customer experience, the domain has significant change over the years. It has become more complex in a sense, and there are several factors for this. One factor is the diversification of the touch points. Traditionally, the typical customer touch points are face-to-face channels such as shops, branches, contact centers, mobile applications, eCommerce sites, etc.
Next to this, we now have the social media channels, WhatsApp, forums, chatbots, and store reviews that customers are reviewing. That’s one factor. Another one is the number of interactions significantly increased over the years. Instead of going to the shop or going to do eCommerce site and making an order, you look at the product online, make your iteration, go to the shop, try their products, and ask the shop to send the product via a carrier to your place, etc. There are now three interactions for interactions instead of one.
The other factor is the diversification of the contents. In addition to structured data, there is non-structured data everywhere. It’s not only the text open-ended feedback, which is a natural language processing that’s being processed. It’s also the speech of the customer and the speech recognition technologies that are enlightening the customer feedback. It’s visual recognition as well. The nature of all this feedback has changed. There is a lot more data now to process. It provides a lot of opportunities. The challenge is a bit different. The challenge now is to identify that 1% time of the insight.
[00:14:43] Eric: The exciting and frustrating part about being in this business is that as time goes by, there are these inflection points for how customers interact with their brands, for the data that you can capture, for the things that you can do with that data, and for the ways you can optimize them. This is why in many ways, it’s an era of partnerships.
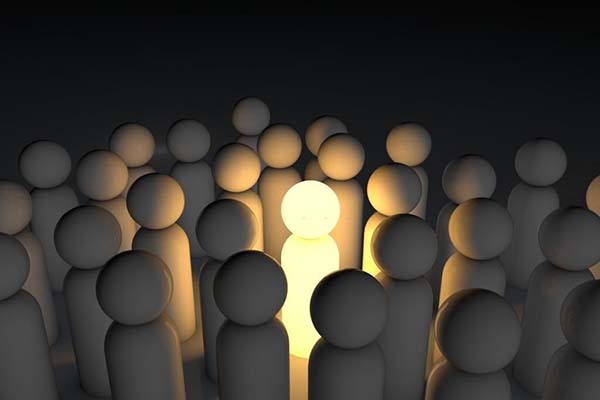
Data Science: It’s absolutely important in today’s world to aim for hyper-personalization. It’s the only way to stand out.
We see this in our industry with a couple of rising stars like Snowflake, for example, on one hand, and Databricks on the other. These are two powerful data platforms. They have their own architecture underneath and they are significantly different, but they’re the same in that they’re enabling companies to grab lots of data, make sure they purify that data and get high-quality data.
We’ll talk to Mary MacCarthy about this reverse ETL stuff. You take the purified data and feed it to your application. How about that for a crazy idea? It’s not enough to see in the warehouse what’s good. You want to be to pump that back into your applications and enrich them so your people can do an even better job.
I view data science as the totality of processes around which you manage data, capture data, refine data, enrich data, and deliver data. All of that is part and parcel of data science as a discipline and practice. What’s great about it is that you have this discipline that is baked into the scientific process, and that should have been there always with data, but it wasn’t. In many ways, data was an afterthought.
You can see this if you go back to the beginning of computers. Where was the data? It was on punch cards that you would stick in there. Even the first computer I had, the first portable computer was a compact portable back in 1986, 1987, or something. It was 50 pounds. It was heavy. You would load the OS on a floppy drive and all the data was stored on our floppy drives. It’s not an efficient way to store data, as it turns out. It turns out floppy drives are not great.
My point is that you could tell the data itself was an afterthought, which is bizarre. Why would you have it as an afterthought? It was early in the day. We still had to build stuff around it. These days, data is central to all of it. Data warehouses are everywhere. Data links are everywhere these days. Going to these link houses we talked about.
The point is you have to pull the data in, make some use of it quickly, and push it back out. That’s the space of the so-called reverse ETL. Mary MacCarthy, that’s your space. You folks help companies take data from the warehouse and fuel their applications with it to enrich the applications, get the latest data, get the latest numbers, and get the latest insights into the day-to-day business applications that run the organization.
[00:17:51] Mary: Hightouch is a warehouse-centric technology. Customers come to us and say, “We want to implement Hightouch.” We’re like, “First, do you have a warehouse as your single source of truth?” I wouldn’t usually refer to that type of knowledge as science, but I like the way you’re talking about data sciences, doing things in the right way, and the right processes.
Sometimes we use more of the analogy of software engineering but it is doing things scientifically. You don’t have one metric up here that says one thing and another metric over here that says another thing. There’s a different number down here. The reality of many companies is that. Your data is a swamp. It’s all over the place and you have inconsistent metrics.
You need to turn insight into action very fast in order to make an impact. Share on XIf you set up your warehouse properly, get your data in there, unify it, enrich it, and transform it, that’s beautiful and great. You have got a great data warehouse. Unfortunately, that becomes yet another data silo. The beauty of a reverse ETL and our brand of it, in Hightouch, is we will help you get it back out to the business tools that your teams working on. Whether that’s the CRM like Salesforce., HubSpot, your marketing tools, ad spend tools or even other internal databases where you might need that data. It’s all consistent enriched data from that single source of truth.
It sounds obvious. You would think this is how things would have been done all along, but data evolved in a way that wasn’t necessarily scientific. The reality of those pipelines is that they’re hard to build. When you buy a tool like Hightouch, we do that for you. It’s plug-and-play. It works fast. The pipelines are built automatically. We integrate with over 100 destinations and we maintain it for you. We take all that hard, many hours of long data engineering work, which is frankly expensive. We do all that for you with our product.
[00:19:28] Eric: I have a tagline. I’ll throw it. It’s a play on words like the famous Las Vegas tagline. “What happens in the warehouse should not stay in the warehouse.”
[00:19:37] Mary: We’re working as data professionals towards ending the siloing of data. We want data to be empowering business decisions. If it’s stuck in another silo, that’s no good to us. If most, it’s going to analytics. Analytics is great, but that is one step towards eventually making business decisions. Whereas with reverse ETL, we’re getting it straight back into places where you can use it that day in real-time, your marketing tools, sales tools, and ad tools. We’re not saying we’re replacing analytics. There will always be a place for analytics and BI but we’re giving you this other way of also using your data in parallel.
[00:20:14] Eric: You talk about data silos and there are also departmental silos. There are organizational hierarchies that tend to foster siloed approaches to things which is not good. You don’t want your salespeople in a different world from your marketing people. You don’t want admin in a different world altogether accounting, but you want us for all these departments to be connected and you want that connective tissue to be the data and the data flows.
[00:20:40] Mary: You want it accessible like our tool to get the data from the warehouse into those tools. You don’t even have to know SQL. You can write the models to send it there, to think it in SQL, or you can do it in a no-code way for people who aren’t SQL users. Our mission is to get people access to the same data and the same metrics. We’re working in a way that each team and department are data-driven. We all talk about data-driven companies, but most of us who work in data know that most companies aren’t data-driven. The other guests might feel differently, but that’s what I have seen.
[00:21:14] Eric: There are levels in the depths of being data-driven. What I love is that in the warehouse world, we built data warehouses because people figured out several years ago that it was hard to query an operational system like your ERP. It was not designed for giving visibility into trends and things of this nature. They figured out, “Let’s go ahead, pull this data out, and we’ll load it.” That’s the ETL. We extract it from the source system like an ERP, Enterprise Resource Planning. We transmit and transform it into a particular schema and load it into the warehouse.
The analysis was done in the warehouse by a handful of people who would go, “We should buy more red suits instead of blue suits.” They email someone and say, “Why don’t you buy more red suits than blue suits?” They came back and say, “I don’t like those blue suits. I don’t like red suits. Why do I want to do that?” It was this offline process that was not terribly efficient. What you want is for the warehouse to be the trusted source of purified information. You want to open those spigots to send the targeted data back to the different applications where it matters most.
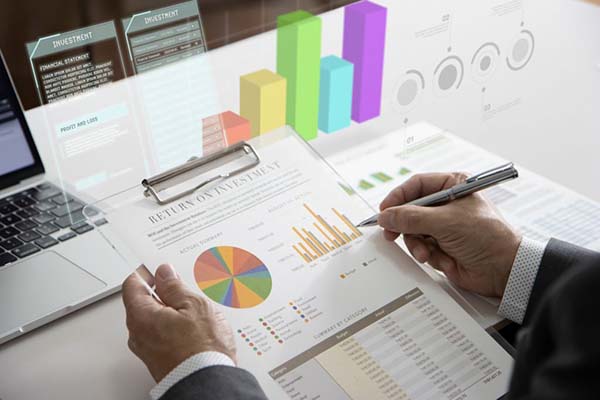
Data Science: We all talk about data-driven companies, but most of us who work in data know that most companies aren’t data-driven.
From marketing, you want to know, “Who are my high-value targets? Whom do I want to be marketing to with these services?” For accounting, you want to know, “How quickly do I need these numbers versus those numbers?” There are all these different use cases around that. The key is that you do want to have this trusted source. That’s the warehouse and Hightouch comes in to hydrate all the other applications.
[00:22:44] Mary: You can bring the data science models into that. Ideally, your data science model can be stored also in your warehouse. You have some brilliant data scientists who built a propensity score for which customers are most likely to buy a certain product. Let’s get that out to the people who can use it the most, the ad people, the sales team, and the marketing team. As much as I’m a data science skeptic, let’s talk about data science and how it fits into the rest of the data infrastructure. To me, that is the discussion that sometimes is left out. You’re much an expert on that. I think you’re also a data infrastructure nerd, Eric.
[00:23:18] Eric: I get into this stuff. It’s exciting, and what our readers should understand here is that everyone on this episode can collaborate. It’s not like you’re going to go with Hightouch, Tredence, or Alterna CX. They are different use cases, but they’re all built around the data foundation. What I keep proposing to folks is that data science represents a maturation of the data management world and an appreciation for what’s out there.
For example, we hear about observability all the time now. That’s not because it wasn’t there before. It’s because we are able to observe so much more than we were able to observe several years ago to do lots of different things that are happening. Maybe, Zohra, I’ll throw it over to you to comment on that. We’re constantly in this constantly-changing world where there are more information sources coming online. We can do more with it. We need to personalize more. The individual companies need to be focused on that last mile to bring your concept into the picture here. That’s a good place to start.
I gave a webinar talking about assumptions, priorities, and execution. I’m going to go ape on people. You make certain assumptions about the marketplace, what you’re doing, and what your tech stack is. You build your priorities around that. How can we make more money or get new customers or whatever? You execute and you can continuously go back and reevaluate. I have different assumptions.
We’re in one of those periods of time right now, where we are coming full circle on lots of different issues. We can start having much more practical conversations about how to apply AI and ML. It used to be a great thing in search of a use case. We have got lots of use cases. The key is how quickly can we get them done and how effectively can we get them done.
[00:25:04] Zohra: There is no debate on how you can use the AI and ML or what they can be used for. There are thousands of use cases that anybody can come up with. It’s about which ones you prioritize first and how you prioritize. At Tredence, we work with a lot and we have our own in-house activators and frameworks that we bring in first of all. That starts across the entire life cycle from being able to prioritize your use cases. Which ones are quick to market and have a high impact? How do you prioritize your use cases on various metrics?
It could be business outcomes, speech to implementation, or a combination of a lot of things, but it is our first job, to be able to prioritize in those spaces and use the infrastructure and platform to be able to build it. One thing that I would like to mention and bring it up here is the explainability of AI, which is becoming increasingly important. That’s something that is specifically focused on because we have always been focused.
Data science is not just about data and machine learning. It is about the domain. Share on XThat is also one of the things that are relevant about the new age of data science which is coming in because the focus has always been on getting the right accuracy, getting the models, and being able to predict, but now, the focus is moving on why, how, and what is happening. Being able to explain what is happening is extremely important because we have much data and sensitive information out there.
It’s important to be able to not make not only the right use cases but fulfill the right MO that is in line with business ethics and a lot of things. That’s something that Tredence focused on. It’s the whole life cycle. It’s not just about prioritizing use cases, but also prioritizing the AI and ML that you’re using to build these use cases.
[00:26:59] Eric: For our readers to understand, there is this concept of a black box. For years, a lot of analytical software was delivered as a black box. The company bought a particular technology. They came in and say, “We’re going to optimize your pricing for these different widgets that you’re selling. You’ll have to trust those that it works.” In a lot of cases, it did work because they came up with good models. They just didn’t want to share what those models were.
I’m pretty sure that the Open Source Movement started with Linux and Linus Torvalds. It climbed up the stack. The Apache web server was the first one out of the patchy. Now, we have had Duke and all these open source projects. There are hundreds of them. Not all of them get big, but they’re out there. That changed the dynamics about what people expect and what people want. There are lots of benefits to open source. It’s not perfect like anything. There are some downsides, too.
I’ll throw this over to Gurol from Alterna CX to comment on, but I do believe that the spirit of transparency has taken hold. That’s good for lots of reasons. One of which they say is with open source, “Bad code goes away because you have got a lot of eyes focused on the code. You get the community collaborating to build things. You have committers at staunch competitors working together on a particular project because a rising tide lifts all boats.” The explainability is great. It’s spinning out of that spirit of transparency, but what do you think, Gurol?
[00:28:24] Gurol: it’s explainability as well as actionability. We can analyze the data as much as we want, and we can create a myriad of data in terms of insights and quantity. When you don’t take action, it’s no good for anybody. At Alterna, we focused on a couple of things. First, we said, “What are the teams? What are the experiences that are creating certain emotions in the customers? What’s making them happy? What’s making them upset, angry, or excited?” Secondly, what is driving these emotions? What are certain aspects of customer experience? Is it the wait time? Is it the employee attitudes? Is it the ease of access? What is causing these emotions?
Finally, we also wanted to say, “What if we improve this area? What if we make an improvement in satisfaction in delivery time or ease of access? How much effect is it going to have on the ultimate outcome in customer satisfaction and my KPIs?” All of these models that we built are around actionability. We are trying to predict the angry customers, what’s causing the anger in them, and what we need to fix first to address that anger. We can retain the customers and the customer becomes an ambassador because we listened to the customer and we took action and the customer became this way.
[00:29:44] Eric: There’s an interaction. This is a good point that Gurol is making. You want to be able to track what you’re doing, make sure it’s working, and benefit from that virtuous cycle of learning and getting better. We have been talking to Mary MacCarthy from Hightouch doing reverse ETL, Zohra Ladha from Tredence doing AI and ML, especially around supply chain stuff, and Gurol Kurt from Alterna CX, dialing in from Turkey. We have got Turkey, Canada, and LA on the show. Pittsburgh for yours, truly. We’re truly global.
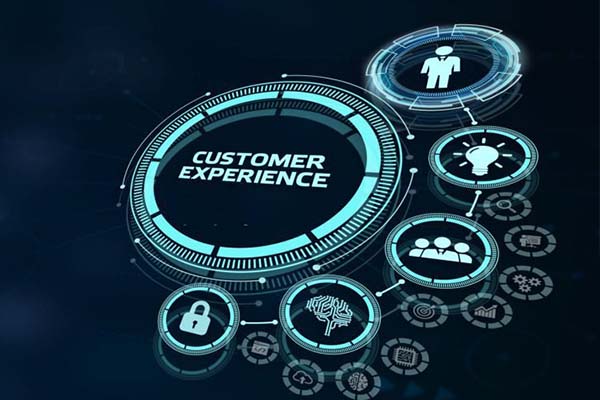
Data Science: The supply chains definitely could be a root cause of the dissatisfaction, but it’s the entire customer experience journey and all the stages and touchpoints that can create those reasons.
Around use cases, voice supply chain, pretty hot area these days when you have got COVID and wars going on. You have got all kinds of downstream effects of this form of war in Ukraine. That’s where technology can come in handy to find new sources and optimize the flow of goods from one place to another.
[00:31:01] Zohra: These are times of a lot of uncertainties. COVID has taught us a lot in terms of supply chain and we have seen massive changes. That’s where AI and ML have been important to us being able to try some of these supply chain efficiencies, whether it’s in terms of being able to forecast demand, managing suppliers, managing things, and optimizing inventory with our clients.
We have been able to show so many efficiencies in terms of dollars saved and losses recovered in terms of timely delivery, transportation, and logistics. We are getting goods on time. AI and ML have been instrumental in solving the entire supply chain problem and in every stage that we have seen, we have been able to show the cost savings, revenue increase, or other efficiencies in a tangible and impactful way.
[00:31:56] Eric: That’s important for lots of reasons. Think about the supply chain real quick, back over to you, Zohra. If you’re a car manufacturer and there is a particular part that now can not get here because it was made in some part of Ukraine, for example, the more lead time you have to understand it’s not going to be there, the better off you can be. You can either pivot and get a new part to fill that in or pivot your manufacturing to different models of cars. These are difficult decisions to make. Unless you have all the data in a timely fashion, you can’t make that.
I spent years in the print publication business before going digital. When I went to do a press check one day, I’m down there talking to the guys, running the machines, and the guy told me, point blank, “When these machines aren’t working, we’re not making money.” You want to make sure that the machines are humming all the time because as soon as they stop, it’s a gigantic cost center that’s sucking up all of your cashflow. It’s important to get that stuff.
[00:32:53] Zohra: It’s not about having the right data to make decisions. It’s about being able to make decisions in time or rather before time so that you can have a backup plan. If some part of the car is not going to be available, what is the replaceability and where do you get that from? If you’re able to predict out-of-stock or inventory mismanagement ahead of time, you’re able to be able to predict demand well ahead of time, knowing what’s coming up. You can plan better and identify it by the substitutability, whether it’s increased manufacturing or efficiencies in processes. It’s important to be able to do that. What’s the right data with enough lead time to be able to make amends?
[00:33:36] Eric: I’ll throw it over to the Gurol Kurt from Alterna CX. One reason why customers make it unhappy is that their products aren’t there and they want to buy, or their parts aren’t there when their car breaks down. The supply chain is part of this, but you’re able to capture why they’re upset. Maybe go and explain to someone, “You guys should have had Tredence working with you to solve the supply chain problems because now all of our customers are unhappy.”
[00:34:00] Gurol: The supply chains could be a root cause of the dissatisfaction, but it’s the entire customer experience journey, all the stages, and all the touch points that can create those reasons. I want to elaborate a bit more on the actionable insights. Generating actionable insight is important, but how you turn that insight into action is also important. You need to turn your insight into action fast in order to make an impact.
Data science is a continuous learning process. Share on XFor instance, traditionally, whenever a customer had a headache situation, she wrote a satisfaction case on social media. Maybe you send out a survey and the customer responded back. By the time you took action, it’s maybe 5 or 10 days later. The customer is already gone. One strength that we are seeing is tying this insight into fulfillment systems, such as robotic process automation systems. It’s not every case because the human factor is still important for customer experience.
In those cases, where it makes sense to automate the floor, for instance, whenever you catch the dissatisfaction case, the customer dissatisfied about a certain fee or commission that the customer paid, you understand that customer is right. You can automatically treat integration trigger the RPA tool that is in charge of refunds.
The system is to refund and you immediately messaged the customer saying, “Dear customer, we heard you. You are right. We are sorry. We will refund you.” Can you imagine, you tell about your dissatisfaction and that company takes care of it in half an hour? You immediately turn that unfortunate case into a fortunate case for the company. These are the things that we need to be paying attention to.
[00:35:37] Eric: I’ll throw it over to Mary MacCarthy to comment on from Hightouch. Getting that data from the warehouse into your applications quickly is important. The cool thing is I learned this a long time ago from a psychologist or an expert in psychology talking about engagement. What they watch out for and the worst-case scenario is apathy.
When someone doesn’t care, it’s hard to persuade them to go one way or another. When someone is agitated, annoyed, or irritated, they are very much engaged in the conversation. It’s an opportunity to do good customer service if you feel the anger and they sense that you understand and appreciate the gravity of the situation. That’s when you can turn around a bad situation to make it a good situation, which winds up improving morale, net promoter score, and all these different things come into play.
In order to do that effectively, that customer service rep or whoever is representing your brand in this particular unpleasant situation needs the right information to be able to make a decision. They need to see the odd. This is a high-value customer. He bought these five things. He called and emailed two days ago. The more that the person can communicate they’re on top of the situation, the better off they’re going to be. That happens with data.
[00:36:54] Mary: Eric, Gurol, and Zohra, you all speaking my language. You’re talking about taking the work that we do. As data practitioners, that’s hard work that we all care about, make sure that you can take action on it, and do it in a timely manner. Here in Hightouch, we leave most of the fancy stuff to you guys, to the data scientists, and the people building the thing. We do that last part for you and get it where you need it.
The customer service example is perfect. Our most common use cases sending data from the warehouse to a CRM like Salesforce. Let’s say we have a company that has a customer service agent or manager. Somebody is talking and a customer wants to talk about renewal. They’re maybe not happy about something. Typically, the customer service manager won’t have a real 360 view of information about that customer, because some of that is in dashboards. Some of the maybe product uses information is stuck in a report.
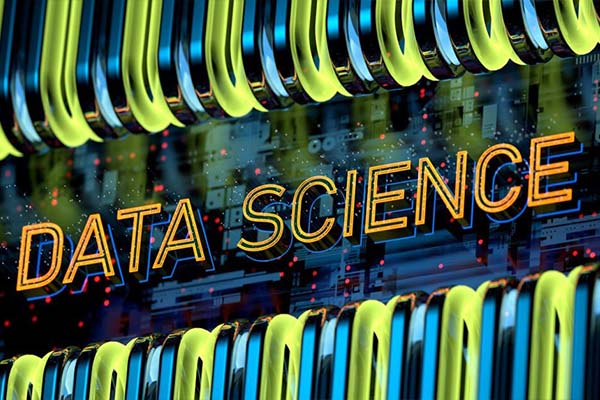
Data Science: It’s not data science. It’s not analysis for the sake of data analysis that we should be offering because not everybody can do it. We should be offering value by creating actionable insight, and we need to be flexible while we do that.
With Hightouch, they have the full 360 views of the customer of their product usage when they signed up, how long they’ve been with us, and everything. They can bring all that information to that conversation. In a company nowadays, all that data is there, but it’s usually not in the right place, the right person, or at the right time. That’s what we can do for you.
[00:38:02] Eric: There is so much data all over the place, but is it at the right place at the right time? Can someone get access to it quickly? These are all important points. Maybe for the last thought, I’ll bring Zohra back in from Tredence. You’re talking about supply chain dependencies. Data is important. Understanding dependencies is as important as the data because when this domino falls, that domino is going to fall out. All these things are going to happen. In the supply chain world, those dependencies, you’re talking many to many types of situations and it can go off the rails.
It takes a lot of time to get that right but here comes a fantastic benefit of machine learning and AI. As machine learning algorithms, they don’t sleep and go on vacation. They could sit there, crawl across data all night and all day, and focus on the important things. What are the high-value products that we need to sell? The further you get down in terms of profitability, the less important they probably become. Machine learning can be useful in distilling dependencies and giving useful recommendations to the company.
[00:39:05] Zohra: AI makes a contribution in terms of prioritizing and crystallizing insights. It mines through millions of data points and picks up the relevant insights and relevant learning. Most importantly, one point that it also does is that keeps learning from its own models, which is important in the sense that milestones and insights are taking action. It may or may not have it well so can trace that back into models so that your models can learn better, and compute what is working as well as what is not working. That’s important.
[00:39:47] Eric: It’s time for the bonus segment on our show. We have been talking to Gurol Kurt, Mary MacCarthy, and Zohra Ladha. Zohra, you’re on a roll there right before the main show was ending but you bring up a good point, which is this virtuous circle. You want to constantly be on top of this. I point this out all the time, but it bears repeating that no matter how good your AI and ML become, no matter how good your automation and data are, there will always be a need for people to run these systems and manage them. It’s in understanding the complexity and having the domain expertise that the magic happens.
[00:40:24] Zohra: Data science is not about data and it’s not about machine learning. It is about the domain. It’s a data scientist that wears many hats. It is the domain and match. The ML behind it is understanding the data. Now, it’s also understanding the nuances of software engineering to be able to make viable data science products. It’s a lot of things that go into building a complete data science product.
Without domain expertise, understanding the industry, and understanding the functional domain, you don’t make full use of that machine learning because there is a nuance to it in terms of how the business works. That goes whether it’s the supply chain or any other domain and how these domains work because there are many nuances outside of that data does not capture that you need to incorporate into your models. That’s important. That’s why it’s never a one-person job. We always say, “Data scientists are unique unicorns.” The definition of a unicorn keeps changing. There are more features getting added to the unicorn.
[00:41:27] Eric: I’ll throw this over to Gurol Kurt because it is an interesting dynamic in the industry. You can go out and use all sorts of algorithms for free like TensorFlow. You can grab all kinds of algorithms and roll your own. What the big guys like Google Cloud Platform, Microsoft Azure, or Amazon Web Services want is for you to do the computing in their environment, because they’ll charge you for the computing. That’s how they make money. It’s sticky.
Data is a human commodity. All the things that make it beautiful are the human factors and the people who work with it. Share on XYou can go ahead and roll your own, but it’s a hard thing to do. I’ll throw it over to you to comment on how, as a corporation, as a going concern, Alterna CX, you need to stay on track with building out your indicators or your signals that you’re able to capture from behaviors and emotions and focus on the usability side of the equation to make it more attractive for companies to work with you instead of trying to roll their own.
[00:42:21] Gurol: We need to create value at the end of the day. It’s not data science. It’s not analysis for the sake of data analysis that we should be offering because not everybody can do it. We should be offering value through creating this actionable insight and we need to be flexible while we do that. All these big companies want us to use their platforms and resources while we are doing this analysis. We never rebuild our models. We offer flexible options and different environments that can be used to run these models. We try to be as open as possible.
I want to mention one more point. Zohra was mentioning about the continuous learning process. This is a continuous learning process. There’s also a conscious journey in the sense of unsupervised data generation. When the models continue to learn, they do learn, but there are also unsupervised models that we are working on and we are offering.
Its purpose is to create hidden insights. It has nothing to do with training. The models try to understand what the customers are talking about and around those topics that we and our client companies didn’t guess before. There is something new going on and the customer started talking about it. Our models try to find those hidden topics and insights using price. The learning feature is not only around supervised training but also unsupervised training as well.
[00:43:46] Eric: I gave a keynote a few years ago before the pandemic came along about artificial intelligence versus data warehousing. Will one take over the other? My whole argument was, “No, you want both.” You want to have your trusted view of the world from the data warehouse, but you also want artificial intelligence to be scanning and looking for things. AIs are just algorithms at the end of the day. You’re applying algorithms to data sets and spitting out results, which are either scores or some histogram of some kind.
You’re looking for stuff that’s anomalous. You’re looking for strange things out there. The concept I came up with that I would be curious to hear your thoughts on here is that with data warehousing and AI, you have your right and your left eye. If you only have one eye, you can’t do depth perception because your brain can’t train it and understand how far away this is from me. That’s what you get when you combine AI with data warehousing. You get some depth perception to help you understand your environment, what’s happening, and what you can do about it. What do you think?
[00:44:52] Gurol: When the AI and the data environment get together, they create the magic. The human side is important. People who are looking at and interpreting the data, and taking action are important. The database warehouse environment, the AI itself, and the people, when they get together, create the magic.
[00:45:15] Eric: Final comments from Mary MacCarthy. What’s the name of your show, The Data Tea? Where can people find it?
[00:45:19] Mary: The Data Tea, LinkedIn Live every week on my employer’s page Hightouch. You can look for Hightouch, or you can look for me, Mary MacCarthy. I read posts there. I’m bringing in an interesting personality. I spoke to Kevin Hu, who’s the Founder of a data observability platform called Metaplane, and various conversations about the data culture wars that we’re all thinking about.
I would like to end by saying that I love that this was a data science conversation that was extremely practical in what everybody had to say. It wasn’t my critique of data sciences. Much of it is hype things that companies don’t necessarily need until they have the rest of the infrastructure. Those are the two eyes, the right eye and the left eye of the data warehouse and the AI, but you need the data warehouse first. Start with one solid eye and add in the other eye. I appreciate that you kept that practical down-to-earth factor and added in as Gurol did the human aspect. Ultimately, data is a human commodity. All the things that make it beautiful are the human factors and the people who work with it. Thanks for keeping it real.
[00:46:27] Eric: Folks, look these guys up online. Gurol Kurt from Alterna CX, Mary MacCarthy from Hightouch, and Zohra Ladha from Tredence. We’ll talk to you next time.
Important Links
- Data Science Versus Data Management, Will One Subsume The Other?
- Alterna CX
- Tredence
- Hightouch
- Snowflake
- Databricks
- Apache
- TensorFlow
- LinkedIn – Hightouch
- Mary MacCarthy – LinkedIn
- Metaplane
About Zohra Ladha
A senior analytics professional with over 14 years of progressive, multi-geography experience in the Analytics Industry, with specific expertise in Customer analytics, Marketing ROI optimization, AI, Machine Learning and Big Data. I specialize in helping organizations institutionalize analytics to transform decisions and achieve measurable impact.
Specialties:
– Data Science, Machine Learning, AI
– Consumer and Business Banking, Credit Card, Travel, Retail and Loyalty Analytics
– Analytics Consulting
– Client Management
– Business Development
About Gurol Kurt
Before starting Alterna CX, Gurol was a customer experience director at Telia Sonera, the Nordic telecom company overseeing customer experience transformation of 7 countries. Prior to that, he was a partner at Peppers and Rogers Group, a niche management consulting house known as the authority for customer centricity, working with clients in North America and Middle East.
About Mary MacCarthy
Just another over-educated woman.
Host of The Data Tea ☕, a weekly show featuring creative, innovative, and inspiring voices in the data industry. Drop me a DM here on Linkedin if you’d like to suggest a guest!
Check out my new Linkedin newsletter: “In The Comments.” It’s a look at what’s driving conversations in the data community:
https://www.linkedin.com/newsletters/in-the-comments-6932086963881558016/
AI AIML Data Analysis Data Management data science Domain Expertise